In a landscape characterized by relentless waves of data, organizations are either overwhelmed or empowered. That distinction depends entirely on their ability to harness data’s potential.
In a world where every click, transaction, and interaction leaves a data footprint, you have to ask yourself: How can I derive meaningful insights from an ever-expanding dataset? Big data analytics is the answer.
Table of contents:
The term "big data" refers to extremely large volumes of data that traditional processing methods may struggle to handle efficiently. Therefore, big data analytics is the process of examining large and complex datasets, commonly known as big data, to uncover valuable insights, patterns, trends, and correlations. Techniques such as statistical analysis, machine learning, and predictive modeling are all used in this process.
By exploring both the quantitative and qualitative aspects of data, big data analytics delves into the nuances of unstructured and semi-structured data types. Beyond the traditional trio of volume, variety, and velocity, this analysis also takes into account dimensions such as veracity (ensuring data accuracy and reliability) and value.
We’ve been hearing about big data for so long that it’s become synonymous with data itself. However, that doesn’t tell the full story. Big data analytics is important for several reasons, and its significance has grown in parallel with the increasing volume of data.
Informed decision-making
Big data analytics provides valuable insights from large and complex datasets. You can make more informed and data-driven decisions based on these insights.
OrderPay’s customers wanted to get answers to detailed data questions about performance and customer behavior to make smart, profitable decisions. To scale the business up profitably, OrderPay rolled out ThoughtSpot across the company, allowing users in sales, marketing, product, customer support, and operations to play their part. New self-service access to analytics means everyone can explore, drill down, and analyze data in areas like spend, revenue, tips, performance by date, location, and many other variables to make data-driven decisions. Today, more than 70% of the company’s users log on and interrogate the system often.

Improved operational efficiency
Analyzing large datasets can help you identify inefficiencies and streamline processes. This optimization leads to improved operational excellence, reduced costs, and better resource utilization for your organization.
Identifying market trends and patterns
With big data analytics, you can assess market trends and consumer behavior. This helps in identifying patterns and predicting future trends, enabling your business to stay competitive and responsive to market changes.
Personalized customer experiences
By evaluating customer data, you can gain a better understanding of individual preferences and behaviors. This information can be used to personalize products, services, and marketing efforts, leading to enhanced customer experiences. Understanding customer behavior allows your organization to proactively address issues or concerns, thereby reducing the likelihood of customer churn.
Supply chain optimization
If you are a manufacturing or retail business, delving into data throughout the supply chain can help your organization optimize inventory management, logistics, and distribution processes. This leads to cost savings and improved supply chain efficiency.
Optimizing marketing strategies
You can use marketing data analytics tools that combines big data to optimize marketing strategies by understanding customer preferences, behavior, and the effectiveness of marketing campaigns. This helps in targeting the right audience with the right message.
Fraud detection and security
Big data analytics plays a vital role in identifying and preventing fraudulent activities. By analyzing patterns and anomalies in large datasets, your organization can detect unusual behavior and take timely action to prevent fraud.
Big data analytics refers to processing, cleaning, and analyzing enormous quantities of raw data collected and turning it into a powerful asset. Here is how this process works.
Step 1: Data collection
The first step involves gathering large amounts of raw data from various sources, including data warehouses, structured sources (such as databases), semi-structured sources (like JSON or XML files), and unstructured sources (like text documents, images, videos, and social media). Data warehouses serve as centralized repositories, consolidating data from multiple sources and providing a unified view for analysis. Business intelligence tools may also play a role in extracting, transforming, and loading (ETL) data into the analytics pipeline.
Step 2: Data storage
Once collected, the data needs to be stored in a way that allows for efficient processing and analysis. Big data platforms, such as Hadoop Distributed File System (HDFS), NoSQL databases (like MongoDB or Cassandra), and cloud-based storage solutions (like AWS, Google Cloud, Snowflake, or Databricks), are commonly used for this purpose.
Step 3: Data processing
Big data processing involves cleaning, transforming, and preparing the data for analysis. This step often includes handling missing or inconsistent data, converting data into a standardized format, and performing other preprocessing tasks.
Step 4: Data analysis
This is the core of big data analytics. Various analytical techniques are applied to uncover patterns, trends, correlations, and other valuable insights from the data. Common analysis methods include statistical analysis, machine learning, data mining, and predictive modeling.
Step 5: Data visualization
After completing the analysis, data visualization tools play a key role in transforming the insights from big data analytics into visually intuitive charts, graphs, dashboards, and other representations. Notable platforms like ThoughtSpot facilitate self-service analytics through natural language queries, while Sage offers comprehensive reporting and real-time analytics, ensuring decision-makers can easily interpret and act upon complex data findings.
Step 6: Interpretation and decision-making
Analysts and decision-makers interpret the results to gain a deeper understanding of the patterns and insights revealed by the analysis. This information is then used to make informed decisions, optimize processes, identify opportunities, or address challenges.
Marketing
In the digital marketing world, there is certainly no shortage of data. Website, social, google, CRM, vendors—you likely have more data than you know what to do with. For instance, your marketing team might use big data to analyze social media interactions, website visits, and purchase history to create targeted and personalized marketing campaigns. By understanding customer preferences and behavior, your organization can optimize marketing strategies, allocate resources effectively, and ultimately improve return on investment (ROI).
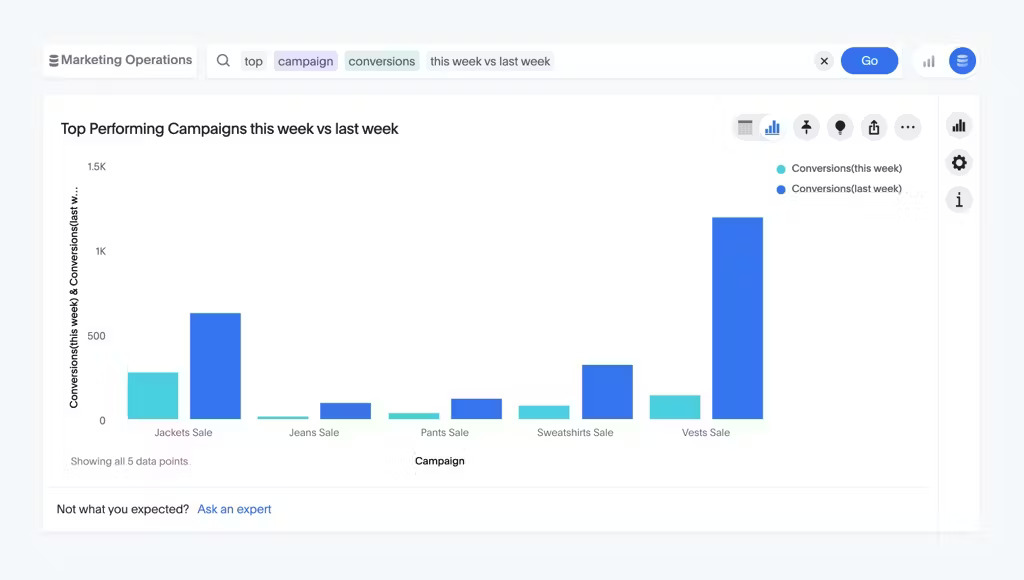
E-commerce
Let’s take a big data analytics example of Amazon. The dominance of e-commerce giant Amazon in the market has reshaped the landscape for smaller online businesses. They face a significant challenge if they're not part of the Amazon marketplace. This has direct implications for big data. Despite challenges, the competitive nature of the industry necessitates the effective use of big data for smaller businesses to innovate, differentiate, and stay relevant in the evolving e-commerce landscape. This includes browsing history, search queries, purchase history, and feedback. By applying machine learning algorithms, e-commerce platforms can provide personalized product recommendations, optimize pricing strategies dynamically, and forecast demand for various products. Additionally, analyzing customer feedback and reviews, including NPS surveys, helps businesses measure customer satisfaction and loyalty.
Healthcare
Having the right data at the right time can be the difference between life and death. Big data analytics in healthcare involves the analysis of electronic health records (EHRs), medical imaging data, patient histories, and other healthcare-related information. This allows healthcare providers to identify patterns and trends, predict disease outbreaks, and personalize treatment plans.
Analytics is a key driver for ZS Associates, a firm dedicated to helping its customers, many of whom are in the healthcare industry. By actively working to disrupt legacy cultures, ZS Associates is enabling self-service analytics and fostering a data-driven culture within its customer base. In addition to leveraging predictive analytics to anticipate prescriber behavior and patient therapy outcomes, they also actively contribute to the broader digital transformation in healthcare.
The ecosystem has been quite sophisticated, talking about the AI use cases, you actually can predict the likelihood of a prescriber writing a script before a script is being written. You can actually prescribe, can actually predict a patient dropping a therapy before it actually drops, or you can actually predict a plan changing their formulary status before it actually happens.
Big data analytics also plays an integral role in pharmaceutical research. By analyzing large datasets to identify potential drug candidates and streamline clinical trials, life-saving medications can come to market more safely and quickly.
Media and entertainment
Streaming services like Netflix use algorithms to analyze user viewing habits, likes, and dislikes, enabling them to recommend personalized content. Moreover, as streaming services continue to dominate the media and entertainment landscape, advertising becomes even more critical for legacy brands.
In either case, big data analytics helps content creators understand the impact of their productions, optimize distribution channels, and target advertising more effectively. Here’s how Netflix’s VP of Data & Insights, Elizabeth Stone, puts it in an episode of The Data Chief podcast:
“We don't want to be fearful of placing big bets. We want to be constantly pushing ourselves to be more innovative and certainly more excellent over time. And we want to use data and analytical thinking to really try to make the best decisions we can.”
Banking
Big data analytics is fundamental in banking for risk management, fraud detection, and customer relationship management. Banks analyze large datasets containing transaction histories, customer interactions, and market trends to identify unusual patterns that may indicate fraudulent activities. Predictive analytics assists in assessing credit risk, and customer segmentation helps in tailoring financial products and services to specific customer needs. Big data also plays a role in optimizing operational efficiency and complying with regulatory requirements.
Government
Governments utilize big data analytics to enhance public services and policy-making. By analyzing data related to traffic patterns, urban planning, and public sentiment from social media, governments can make informed decisions about infrastructure development, policy, and social services. Big data also aids in emergency response by predicting and monitoring natural disasters, disease outbreaks, and other critical events. Furthermore, data-driven governance can help optimize public resources and improve overall service delivery.
In practical terms, this means that data analytics plays a crucial role in shaping the urban landscape. San Francisco's experience showcases how the government leveraged big data analytics to address real-time challenges, especially during the COVID-19 pandemic.
DataSF's mission is to empower use of data. We seek to transform the way the City works through the use of data. We believe use of data and evidence can improve our operations and the services we provide. This ultimately leads to increased quality of life and work for San Francisco residents, employers, employees and visitors.
Telecommunications
In the telecommunications industry, big data analytics is employed to optimize network performance, improve customer service, and manage resources efficiently. Network data, call records, and customer feedback are analyzed to identify areas for improvement, predict network failures, and enhance the quality of service. Predictive maintenance helps telecom companies proactively address network issues, reducing downtime. Additionally, customer analytics aids in understanding user behavior and preferences, allowing telecom providers to offer personalized services and improve customer satisfaction.
1. Descriptive analytics
Descriptive analytics focuses on summarizing and presenting historical data to provide insights into what has happened in the past. This process involves organizing, aggregating, and visualizing data to identify patterns or trends.
Example: Summarizing sales data from the past year to understand monthly trends, identifying peak seasons, or determining product performance.
2. Diagnostic analytics
Diagnostic analytics seeks to grasp the reasons behind a specific event or outcome from the past. This type of data analytics technique dives into data to unveil the fundamental causes behind trends or anomalies.
Example: Investigating a sudden drop in website traffic by analyzing user engagement metrics, evaluating page load times, or examining marketing campaigns during that period.
3. Predictive analytics
Predictive analytics uses statistical algorithms and machine learning techniques to forecast future trends or outcomes based on historical data. The process facilitates informed decisions and the foresight to anticipate potential scenarios.
Example: Predicting future sales by analyzing historical sales data to identify potential growth or decline.
4. Prescriptive analytics
Prescriptive analytics goes beyond predicting future outcomes, offering actionable recommendations to optimize desired results by suggesting the best course of action for achieving specific goals.
Example: Recommending personalized marketing strategies for individual customers based on predictive analytics insights, aiming to increase customer engagement and satisfaction.
While important, implementing big data analytics comes with its set of challenges, ranging from technical complexities to organizational and cultural hurdles. Let’s explore three of the common challenges in big data analytics, and tips for overcoming them:
Data security and privacy concerns
One of the primary challenges in implementing big data analytics revolves around ensuring the security, privacy, and effective data governance of the vast amounts of data involved. As organizations collect and analyze massive datasets, they face the constant risk of unauthorized access, data breaches, and privacy violations.
Adhering to regulatory frameworks and implementing robust security measures, including data governance practices, becomes paramount to safeguard sensitive information and maintain trust among users. ThoughSpot follows security due diligence in the development, data handling, and deployment of all product enhancements. Security gates are in place at every stage of the Software Development Lifecycle (SDLC). In the case of ThoughtSpot Sage, a thorough risk assessment of Azure Cognitive services has been carried out by checking their certification and security posture.
Technical challenges
Scalability is a significant technical challenge in the implementation of big data analytics. Managing and processing large volumes of data can strain traditional IT infrastructure. To address this, organizations need to implement scalable architectures, such as distributed computing and storage solutions, to handle the increasing volume, velocity, and variety of data. Another technical challenge lies in integration, as big data analytics often involves combining data from diverse sources with different formats and structures. Ensuring seamless integration across various platforms, databases, and data types is a complex task that requires careful planning and implementation.
Wellthy, a company that streamlines caregiving, successfully addressed its scalability challenges by implementing ThoughtSpot. Prior to this, the organization grappled with a legacy business intelligence tool that demanded manual generation of reports and requests using Python and SQL, leaving little room for proactive data initiatives. ThoughtSpot's user-friendly interface allowed real-time data exploration without heavy reliance on manual coding. Wellthy also invested in data literacy training, reducing dependency on the analytics team for routine queries. Shifting focus from manual reporting to empowering business users resulted in a 281% increase in active users on ThoughtSpot.
Skill gaps in the workforce
In data and analytics trends 2024, AI jobs are predicted to diversify as companies grapple with the challenge of determining the ownership of AI initiatives. The emergence of GenAI technologies, including tools like GPT from OpenAI, Claude from Anthropic, and Vertex AI from Google, is noted for their reduced need for bespoke training.
The field of big data analytics demands a specific set of skills that are not always readily available in the workforce. There is a shortage of professionals with expertise in data science, including skills in statistical analysis, machine learning, and data modeling. Organizations struggle to find and retain skilled data scientists who can derive meaningful insights from complex datasets. Additionally, the rapid evolution of big data technologies requires constant skill updates.
Professionals need expertise in distributed computing platforms, programming languages, and database management systems. Furthermore, possessing domain knowledge is crucial for effective big data analytics. Lack of domain expertise can hinder the ability to ask the right questions, interpret results accurately, and derive actionable insights from the data.
Hadoop
Hadoop is an open-source framework for distributed storage and processing of large datasets. It consists of the Hadoop Distributed File System (HDFS) for storage and the MapReduce programming model for processing. Hadoop enables the parallel processing of data across a distributed cluster of computers, making it suitable for handling vast amounts of data.
NoSQL databases
NoSQL databases are a category of databases that provide a flexible and scalable approach to data storage and retrieval. They are designed to handle unstructured and semi-structured data, making them suitable for big data applications. Examples of popular NoSQL databases include:
MongoDB: A document-oriented database that stores data in JSON-like BSON format. It is known for its scalability, flexibility, and ease of use.
Cassandra: A highly scalable and distributed NoSQL database designed for handling large amounts of data across multiple commodity servers without a single point of failure.
Couchbase: Combines the flexibility of JSON documents with the performance of key-value stores. It is used for real-time applications and provides a distributed architecture.
Spark
Apache Spark is an open-source, distributed computing system that provides fast and general-purpose cluster computing for big data processing. It supports in-memory processing, making it faster than traditional data processing frameworks like Hadoop MapReduce. Spark provides libraries for various tasks, including data processing, machine learning, graph processing, and stream processing.
ThoughtSpot
As the only AI-Powered Analytics provider in the experience layer of the modern data stack, ThoughtSpot can serve as your main self-service analytics tool or complement your existing BI tool or process, and it’s easy to integrate into your existing data pipeline. Utilizing advanced language models such as Google PaLM and GPT, ThoughtSpot empowers all users within an organization to explore and analyze data on their own using natural language search—without relying heavily on data analysts or IT specialists.
With the help of our interactive Liveboards, you can create and share data stories and apply drill-downs and filters to explore specific data points. Using augmented analytics, ThoughtSpot auto-analyzes your data and alerts users to identified patterns and trends.
For example, Canadian Tire used self-service BI from ThoughtSpot to quickly identify changing demands from customers and shift inventory in the early days of the pandemic. In doing so, they were able to grow sales by 20%, despite 40% of their brick-and-mortar locations being shut down during quarantine.
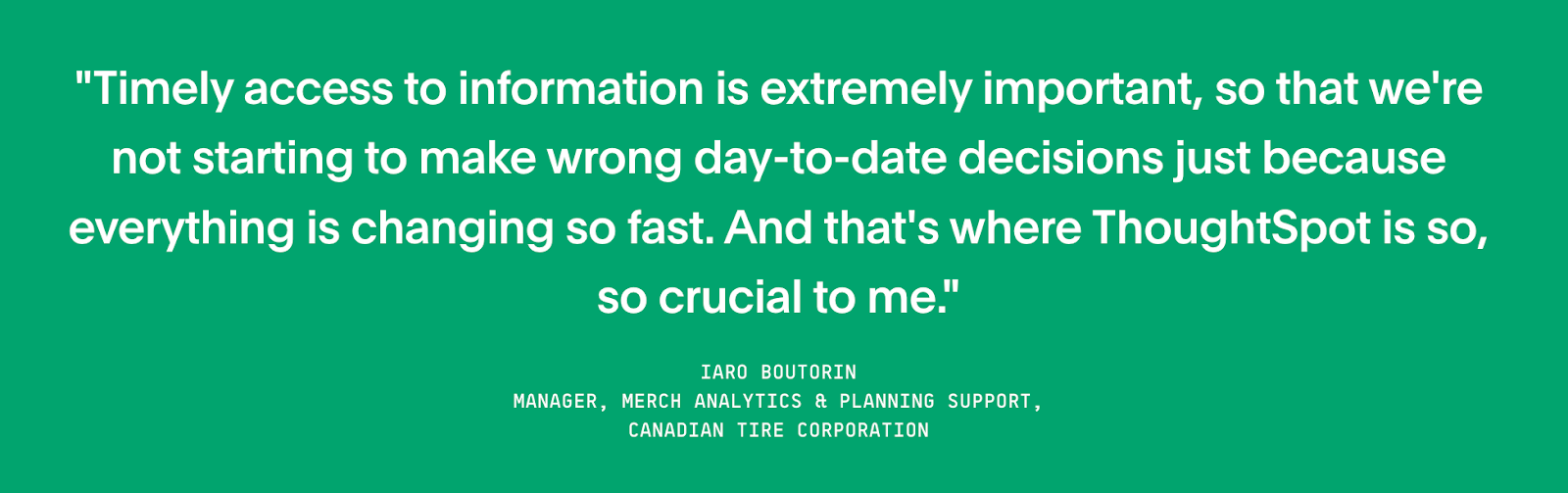
Big data analytics is indispensable when navigating the complexities of today's data-rich environment. The tools utilized in this transformative process are not merely solutions—they are opportunities to reshape the way your organization leverages data.
ThoughtSpot, with its innovative approach and advanced capabilities, goes beyond a standard big data analytics tool. By making data analytics accessible to all, you can generate more value from your data—a goal everyone will benefit from.
Join our weekly live demo to see the power of ThoughtSpot for yourself.