According to a recent CIO.com article, less than a third (29%) of data and analytics leaders are confident that their company’s executives see a link between investing in data and analytics and staying ahead of the competition. And only 44% believe their teams are effective in providing value to their organization. How confident are you in your ability to deliver value?
I've been in data and analytics for over a quarter century, and during that time I’ve learned what delivers business value and what doesn’t. The most successful data and analytics initiatives are aligned with business goals and processes, and they empower people in all areas of the business to continuously adapt and improve. In this blog I’m going to show you how you can help your organization drive operational excellence by shifting your mindset from technology projects to business outcomes.
What is operational excellence
Operational excellence (OpEx) is a management methodology that focuses on improving efficiency, effectiveness, and agility in an organization. It emphasizes continuous improvement within processes and across functional activities to maximize the flow of value to customers. Chief Data and Analytics Officers (CDAOs) may be familiar with other operations methodologies that use continuous improvement principles, such as DataOps (data operations), MLOps (machine learning operations), and FinOps (financial operations).
These continuous operations approaches all contribute to operational excellence by improving the quality across:
improving outputs
streamlining processes
maximizing utilization of resources
Yet, overall organizational performance can still be sub-optimal if these multiple “Ops” activities are not working in alignment with each other and the strategic goals of the enterprise. Without visibility and understanding of the entire value chain of data to analysis, to decisions and business outcomes, employees’ efforts to improve operations in one area can cause problems in other areas. This blog will look at three ways data and analytics leaders can implement operational excellence to improve business outcomes.
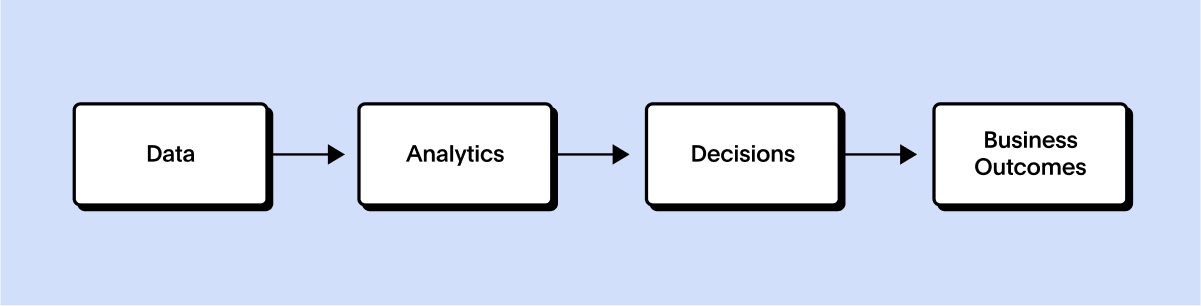
Your data and analytics strategy serves as a foundation for operational excellence by ensuring that data-driven business initiatives are aligned with business goals, operational processes that impact goals are understood, and the impact of the strategy is measurable through business metrics. It must clearly document how to manage and use data to make effective decisions, efficiently execute those decisions in day-to-day operations, and continuously improve and adapt to stay competitive.
Aligning data and analytics to business goals
Aligning your data and analytics strategy to business goals is foundational to operational excellence because it ensures that data and analytics initiatives are directly tied to your organization's overall objectives.
Here are a few benefits of aligning data and analytics with business goals:
Clear direction for resource allocation
Stronger coordination of cross-team activities
Measurable performance management
Guidelines for continuous improvement
One of the many responsibilities of CDAOs is to communicate the vision of how data and analytics can help executives and line-of-business owners improve their organizational performance. The key to getting their buy-in and support for the data analytics strategy is ensuring alignment with stakeholders’ goals.
A few examples of stakeholders and their goals include:
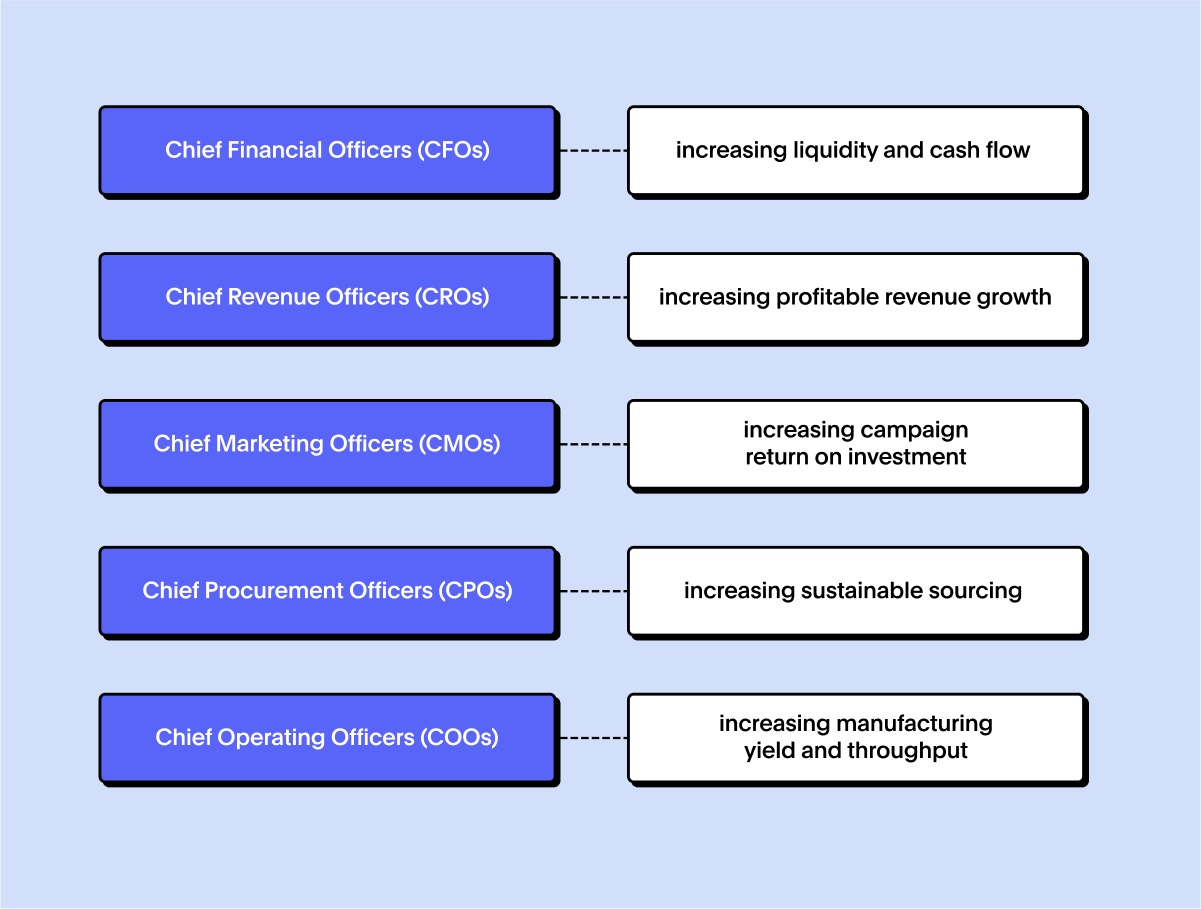
Mapping business goals to operational processes
Understanding the relationship between business goals and related business processes is essential for operational excellence. It facilitates alignment, efficiency, measurement, continuous improvement, and ultimately helps organizations achieve their desired outcomes. Let’s see how processes map to three of the business goals outlined above.
Liquidity management: The business goal of liquidity management, ensuring sufficient cash flow for operational needs, is impacted by processes such as financial close, accounts payable, and accounts receivable. These processes directly affect cash flow by managing timely invoicing, collections, payments to suppliers, and financial reporting. Utilizing efficient Invoice templates can streamline the invoicing process, ensuring timely payments and contributing to effective liquidity management.
Profitable revenue growth: The goal of profitable revenue growth is impacted by processes such as lead generation, opportunity management, and order fulfillment. These processes drive customer acquisition, effective sales management, and timely delivery of products or services—all of which contribute to increased sales and revenue growth.
Sustainable sourcing: The goal of sustainable sourcing is impacted by processes such as local sourcing, recycled sourcing, and alternative sourcing. These processes involve identifying and procuring materials, products, or services that align with sustainability principles, reducing environmental impact, and supporting environmental social and governance (ESG) compliance.
Establishing metrics to demonstrate business impact
By establishing relevant and meaningful metrics, organizations can more effectively measure and manage the impact of data and analytics on operational excellence and overall business performance. To assess the impact on business outcomes, consider the factors that influence the outcomes, such as process efficiency, employee productivity, and speed of decision making. Next, identify the data-centric metrics that impact these factors, including data accessibility, data completeness, and data quality. By establishing a hierarchy of metrics, you can illustrate the connection between data metrics, process metrics, and strategic business KPIs used to measure business goals.
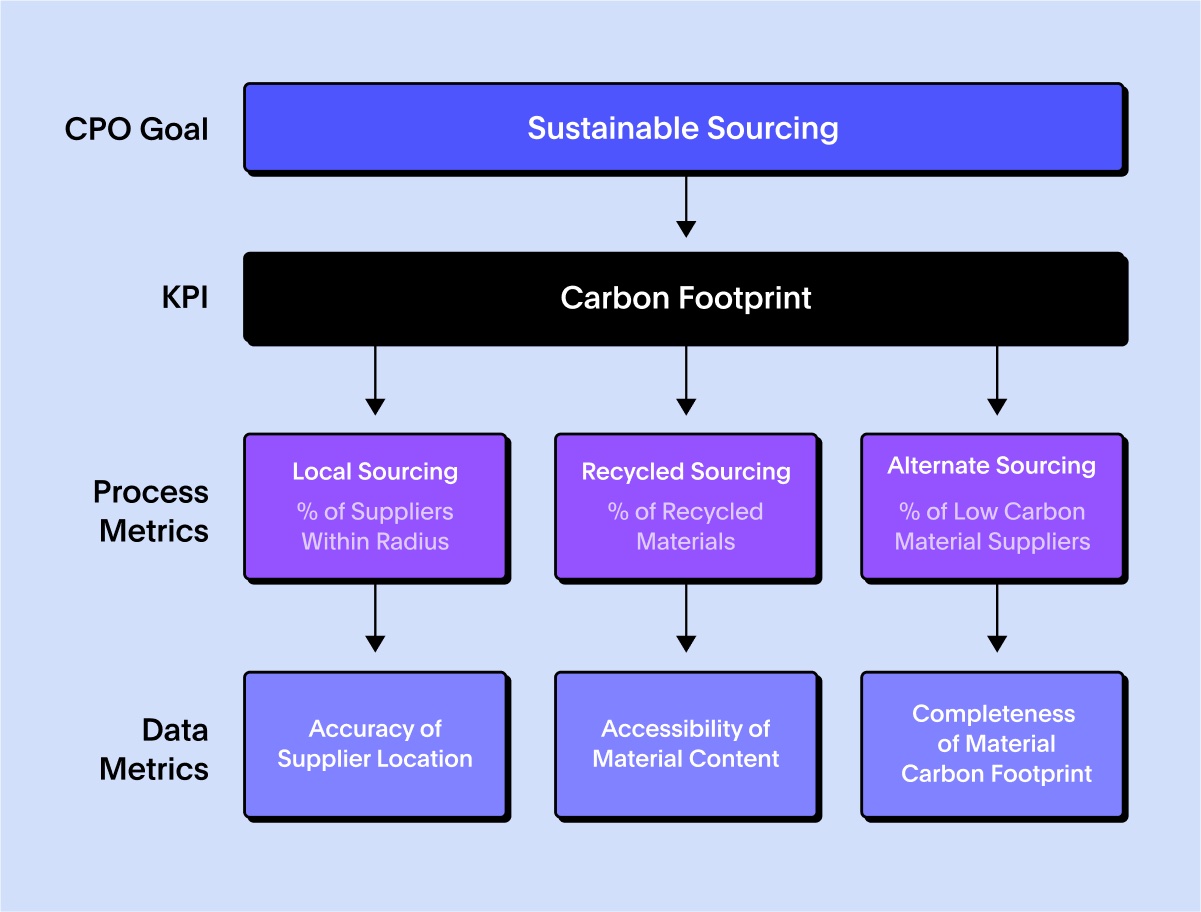
Data governance serves as an operating model for operational excellence by providing a framework and structure for managing and leveraging data effectively across an organization. Establishing policies for data and analytics, defining roles and responsibilities, and facilitating alignment and collaboration across teams helps increase accountability, coordination, and productivity that improves overall operational performance. Let’s break that down:
Establishing policies and procedures for data and analytics
Data governance promotes operational excellence by establishing policies and procedures for data and analytics. This involves defining guidelines, standards, and best practices for data management, data privacy, and analytics initiatives. By having clear policies in place, organizations ensure consistency, accuracy, and compliance in data and analytics related activities. These policies provide a framework for operational practices, promoting operational excellence by ensuring that data is managed effectively and used appropriately throughout the organization.
Defining roles and responsibilities for data and analytics
By creating a common framework for data responsibilities across teams, data governance helps drive individual productivity and organizational performance. This includes identifying data stewards, data engineers, analytics engineers, data scientists, and other roles. Defining the specific responsibilities of each role in managing and using data, such as ensuring data quality, building data pipelines, and training machine learning models is a vital part of managing a data team. That’s because clear roles and responsibilities foster a sense of ownership and accountability, ensuring that teams understand their specific contributions to data and analytics related activities. This clarity helps avoid duplication of efforts and ensures tasks are performed effectively and efficiently, contributing to operational excellence.
Facilitating alignment and collaboration across teams
Data governance helps drive operational excellence by making execution of your data and analytics strategy more effective and efficient. It improves data literacy by providing employees with a shared understanding of data and analytics related concepts, terminology, and best practices. Data governance fosters collaboration as teams and individuals recognize the interdependencies of their activities and begin to understand how their efforts contribute to broader organizational objectives. It helps establish the overall structure and sequence of activities, enabling efficient hand-offs between teams and clear delineation of tasks at each stage of the workflow. Data governance establishes communication channels where teams can learn from one another by sharing their expertise, discussing lessons learned, and developing cross-functional best practices.
AI and advanced analytics serve as an execution engine for operational excellence by modeling scenarios and outcomes, recommending actions for different scenarios, and generating insights using natural language. Accelerating understanding of what might happen, how to respond, and deciding on the best course of action is critical to turning operational excellence from a theoretical concept into real-world management practice.
Without execution your strategy is just documentation.
Increasing operational agility with predictive analytics
By anticipating risks, identifying opportunities to optimize resource allocation, and driving continuous process improvement, predictive analytics increases operational agility. Predictive analytics use historical and real-time data to identify patterns, trends, and anomalies that enable the early detection of potential risks like supply chain disruptions, customer churn, and cash flow shortages before they escalate into major issues. Comprehensive modeling of scenarios and outcomes using predictive analytics can be used to optimize capital allocation strategies across a portfolio of projects based on projected risk, return, and alignment with strategic goals. By considering a range of possible outcomes and their associated risks and likelihood, predictive analytics enable continuous process improvements, such as predictive maintenance schedules to optimize equipment throughput, reduce downtime, and improve production yield.
Guiding decision making with prescriptive analytics
Prescriptive analytics plays a crucial role in operational excellence by guiding adaptation to changing conditions with actionable recommendations. By analyzing customer purchase and browsing behavior, demographics, location, inventory availability, and other information, prescriptive models can make product and promotion recommendations that increase conversion rates and average order values. Prescriptive analytics can improve in-full and on-time delivery rates by analyzing factors such as location, inventory availability, transportation routes, and traffic conditions to recommend optimal delivery schedules, routes, and methods. They help optimize inventory levels by analyzing demand patterns, lead times, and production capacities to recommend optimal reorder points, safety stock levels, and replenishment strategies, preventing overstocking or stockouts, reducing carrying costs, and improving operational efficiency.
Empowering data-driven decision making with Large Language Models
Large Language Models (LLMs) empower broad and consistent use of data across an organization by simplifying data discovery, curation, understanding and use. LLMs' natural language processing (NLP) capabilities enable individuals of all technical skill levels to explore and analyze data by simply making requests and asking questions in plain language without the need to understand complex technical queries or involve data scientists and analysts. By automating and accelerating data cleaning, data transformation, feature engineering, and other repetitive tasks that traditionally require significant manual effort, LLMs increase the productivity of technical experts like data engineers and scientists and accelerate time to value. Training LLMs in specific business domains promote data fluency and culture with context-specific insights and interpretations that assist in the production of summaries and narratives that help audiences understand the implications for improving efficiency, effectiveness, and agility in their area of expertise.
Conclusion
In today's dynamic and highly competitive business environment, operational excellence stands as a powerful differentiator that can drive performance to new heights. Data and analytics leaders play a pivotal role in helping their organizations fuel greater levels of business efficiency, effectiveness, and agility with data and analytics. Powered by a well-crafted data and analytics strategy, supported with a robust data governance operating model, and empowered by AI and advanced analytics, CDAOs can cultivate a culture of continuous improvement and performance optimization.
The time for action is now. Are you ready for success in an ever-evolving business landscape? Having the right advanced analytics solution can help you achieve your goals of operation excellence—providing the collaboration, governance, and advanced analytics you need to empower anyone in your organization to make data-driven decisions.
Request your no-commitment, one-on-one demo to see if ThoughtSpot is the right data analysis solution for your business. If you’re already a ThoughtSpot user, you can stay up-to-date with more CDO trends and best practices by listening to The Data Chief Podcast—new episodes are added every other week.