We’ve been discussing the potential of data analytics for the past three decades. And while we have experienced bursts of progress and new trends, many businesses are still unable to extract real-time insights and make in-the-moment decisions.
The reason? Most organizations think data analytics follows a one-size-fits-all approach. So, they are stuck creating static dashboards that offer limited real-world business value. This point is furthered by recent Accenture research, which shows that 81% of organizations lack an enterprise data strategy to fully capitalize on their data assets.
The solution? Stop following these archaic processes and reimagine your data strategy. By applying specific types of data analytics to unique business challenges, you’ll put data at the center of decision-making.
Table of contents:
Picture this: You are an HR manager looking to understand employee attrition and create effective strategies to improve employee retention. Here’s how using the different types of data analytics can help you make an informed decision:
Descriptive analytics answers: ‘What is the current attrition rate across different departments?’
Diagnostic analytics answers: ‘Why are employees leaving certain departments or job roles?'
Predictive analytics answers: 'Which employees are at risk of leaving in the near future?'
Prescriptive analytics answers: ‘'What actions can be taken to reduce attrition and improve employee retention?'
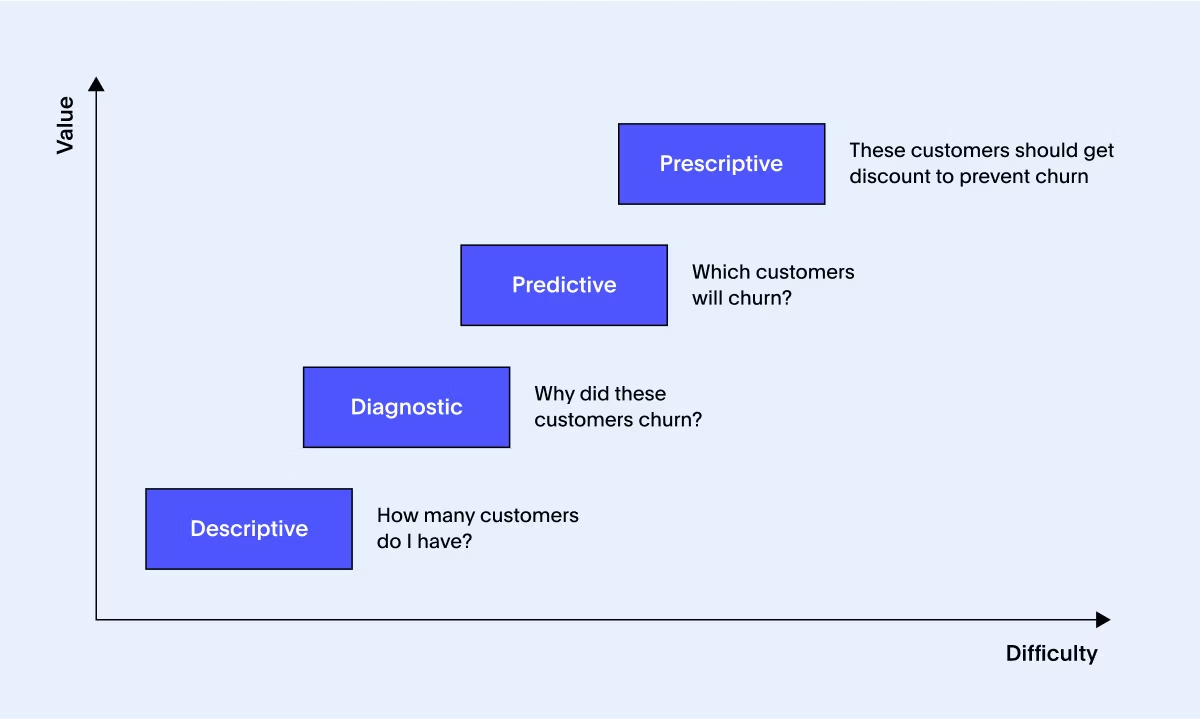
All-in-all, these powerful data analytics techniques provide a holistic understanding of your business. First, you understand past events and uncover their root causes. Next, you leverage big data and AI models to get actionable recommendations. Now that we've a basic understanding, let's understand each type of analytics in detail.
Descriptive analytics focuses on answering the question, ‘What is happening?’ or ‘What has happened?’ by analyzing past data. Of all the types of data analytics, this is the most straightforward approach as it summarizes and simplifies the main features and characteristics of complex datasets through interactive visualizations.
How does descriptive analytics work?
To deploy descriptive analytics, analysts and data scientists typically follow five steps:
Define clear objectives or areas of interest you want to explore in the dataset.
Gather data relevant to your dataset.
Clean data for accurate results.
Apply advanced statistical techniques to condense large datasets into concise summaries.
Use cutting-edge BI tools like ThoughtSpot to run real-time data analysis, create interactive visualizations, and gain AI-assisted insights to make informed decisions.
Descriptive analytics examples
Descriptive analytics offers organizations a data-driven perspective on their operations. It’s no wonder that more businesses across every industry are starting to adopt this powerful approach to problem-solving. Let’s explore some popular descriptive analytics use cases:
Customer relationship management: With descriptive analytics, you can analyze customer data, including past surveys and feedback forms, to get insights into how customers interact with your products or services and what they want from your product. Armed with this information, you can adjust your offerings and optimize touchpoints across all channels.
Financial reporting: Finance teams often leverage descriptive analytics to summarize large volumes of transactional data, compare performance for optimal resource allocation, and assess the organization’s financial health.
Supply chain management: By monitoring supply chain metrics and assessing historical lead times, supply chain leaders can better understand the root causes for delays, minimize disruptions, and identify cost-saving opportunities.
Want a real-world example? Here’s how Cox 2M reduced time-to-insight by 76% with ThoughtSpot:
As the company grew, Cox 2M’s data team was spending a lot of time manually creating reports. The cost to run ad-hoc requests was also mounting—reaching a staggering $90,000.
Cox 2M’s data team now leverages ThoughtSpot AI-Powered Analytics to seamlessly manage billions and trillions of rows of live data and deliver real-time insights, saving up to $70,000 in data costs. They don’t have to build a new dashboard for every query, reducing time-to-insight by 76%. And with features like ‘AI Highlights’ and ‘SpotIQ,’ users were able to discover outliers, cross-correlations, and trends.
This quote highlights how Josh and his team leverage ThoughtSpot to find hidden insights and solve customer problems.
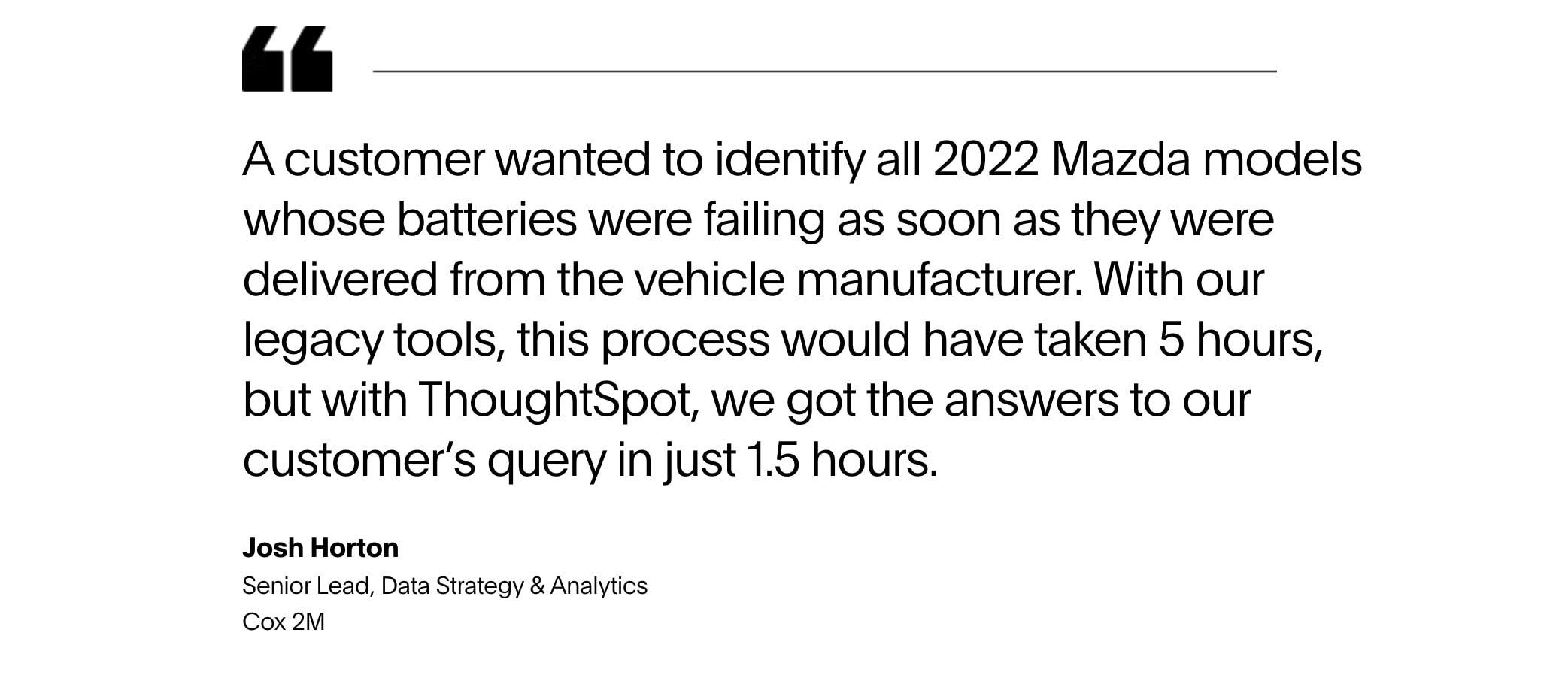
💡Read in detail about descriptive analytics here.
Predictive analytics uses historical data to answer the question, ‘What may happen next?’ Businesses employ this model to predict future outcomes, find patterns, and identify risks or growth opportunities. While descriptive analytics serves as a reflective mirror, showing us a holistic picture of our past activities, predictive analytics acts as a crystal ball, providing a sneak peek into the future.
How does predictive analytics work?
Predictive analytics uses statistical modeling, data mining techniques, and machine learning to analyze large datasets and predict the likelihood of an event occurring. To apply this types of data analytics, you need to build a model and choose the correct analytical technique, depending on the problem to be solved and the nature of the dataset.
To deploy predictive analytics, here are the six steps you should follow:
Define the business problem.
Gather relevant data for analysis.
Pre-process the data to remove missing values.
Choose the right modeling predictive technique. Some of the most common types of analytics models include linear regression, decision trees, cluster models, and time series models.
Develop and train the model leveraging machine learning, AI, and advanced statistical techniques.
Test your machine learning model on a test dataset to assess how accurately the model analyzes new, unseen data.
Predictive analytics examples
Predictive analytics can help you boost operational efficiency, identify growth opportunities, and manage risks effectively across a wide range of industries. This includes sectors, such as banking, retail, utilities, public services, healthcare, and manufacturing. Here are some popular use cases:
Inventory management: Retailers and e-commerce businesses use this type of analytics to anticipate consumer demand for products and perform sales forecasts. By analyzing past sales data, critical retail KPIs, and market data, you can optimize inventory levels, manage resources, prevent stock-outs, and operate more efficiently.
Credit scoring: Banking and financial institutions leverage predictive analytics to make predictions about a customer’s ability to repay a loan from their past credit history. The insights help decision-makers make data-driven decisions on who is likely to default on a loan and which customers pose a high credit risk.
Sales forecasting: Sales teams use predictive analytics to estimate future sales revenue and customer demand based on historical data, market analysis, and other relevant factors. Doing so helps businesses create personalized marketing campaigns, adjust product launches, and capitalize on opportunities that can drive sales.
💡Read in detail about predictive analytics here.
Unlike predictive analytics, which focuses on future outcomes, prescriptive analytics helps decision-makers identify the best course of action to help them achieve their business goals. The primary goal of this model is to answer the question: ‘What should we do?’.
How does prescriptive analytics work?
Prescriptive analytics relies on advanced techniques, such as machine learning, neural networks, recommendation engines, and mathematical algorithms, to offer actionable guidance on what to do next. Of all the types of types of data analytics, this model factors in past and present data to simulate scenarios and offer unbiased recommendations.
Here are six steps you should follow to deploy prescriptive analytics:
Define the business problem you want to address.
Gather relevant data for accurate results.
Develop the model using AI, machine learning, and advanced statistical techniques.
Test and train the model to check accuracy.
Map the outcomes and check whether they align with your business objective.
Monitor and adjust the model accordingly.
Prescriptive analytics examples
Advanced BI tools and prescriptive analytics have made it possible for businesses to analyze large datasets and make in-the-moment decisions. Here’s how different industries are using prescriptive analytics to get the most value out of their data:
Handling multiple IT requests: To keep up with the constant barrage of security and IT tickets, businesses need the right analytics capabilities to make the correct operational decisions. With an intuitive BI tool like ThoughtSpot, you can schedule alerts to quickly identify the problem. Consequently, you can apply prescriptive analytics models to get guidance about the next step.
This is exactly how data cloud leader Snowflake cleared its IT backlog by 20%—read the entire case study here.
Employee training programs: Prescriptive analytics models are helping HR leaders bridge skills gaps within their workforce. The model can recommend personalized learning paths for individual employees based on their roles and job responsibilities, ensuring everyone has the skills they need to excel.
Campaign optimization: As competition becomes fierce, it is critical to create campaigns that stand out. With prescriptive analytics, you can optimize your ongoing campaigns by getting insights into which channels are effective, what timing is the best, and which messaging will appeal to your target audience.
For instance, suppose you receive an alert indicating a significant spike in cost-per-click for one of your primary paid advertising campaigns on Google AdWords, jumping from $0.50 to $20 per click. You need to quickly take action so you don't use your entire monthly SEM budget. Predictive analytics can provide recommendations on actions that can help you optimize your campaign.
💡Read in detail about prescriptive analytics here.
Diagnostic analytics examines past data to identify the root causes behind a particular outcome. This type of analytics aims to answer the question, ‘Why did this happen?’ It focuses on uncovering insights into historical data patterns, anomalies, and correlations to facilitate a deeper understanding of a particular business problem.
How does diagnostic analytics work?
Diagnostic analytics explores the relationships between variables and uses advanced statistical methods to pinpoint the root causes of specific events.
Here’s how this type of data analytics technique works:
Define the problem.
Collect relevant data for analysis.
Pre-process the data for accuracy.
Decide the right type of analytics technique. Some of the popular ones are: Hypothesis testing, anomaly detection, Root cause analysis, and regression analysis.
Apply statistical methods.
Leverage BI tools to visualize data.
Interpret data and share your findings.
With GenAI making data analytics more accessible than ever, you can dig deep into data, understand where things have gone wrong, and proactively take measures to address risks. A great example of this is ThoughtSpot’s AI-driven SpotIQ, where you can analyze large datasets and automatically spot anomalies, correlations, and trends in your data. This AI-powered feature can help you get to the ‘why’ faster, reducing time from insight to action.
Diagnostic analytics examples
Diagnostic analytics helps organizations dig deeper and find areas for improvement, implement changes, and optimize business processes. Here are some known use cases of diagnostic analytics across industries:
Optimizing patient treatments: Diagnostic analytics can help healthcare providers analyze patient records and identify patterns leading to specific medical conditions or treatment outcomes. They can understand factors contributing to patient readmissions and identify areas for improvement in healthcare delivery.
Customer retention: Diagnostic analytics can help you address customer churn by examining historical data to identify the reasons behind customer attrition. This includes identifying engagement patterns, analyzing channel performance, and pinpointing drop-off points.
Website traffic: If you notice a sudden drop in website traffic, it is critical to pinpoint the exact cause so you don’t lose prospects. Using this type of analytics, you can analyze data on user behavior on the website to identify and resolve the issue.
💡Read in detail about diagnostic analytics here.
Establishing a modern data infrastructure and choosing the right BI tool is the key to leveraging advanced types of data analytics techniques. Without access to real-time insights, your team won’t be able to make timely decisions and drive business value.
That’s why companies like Accern, HP, and Odido are turning to ThoughtSpot’s AI-driven, BI solution to transform their raw data into actionable insights. ThoughtSpot’s unique and powerful approach to data exploration and analysis allows users to ask questions in natural language and get AI-assisted answers and insights.
Not convinced? Schedule a free demo of ThoughtSpot to see for yourself.