Diagnostic analytics is a type of data analytics that uses past data to uncover factors and variables that contributed to the outcomes of particular events. This technique drills into data, offering insights into why certain situations occurred and determining root causes. With these takeaways, businesses can make data-driven decisions and better plan for the future.
Data’s true power only emerges when we learn from it—understanding why things happen and applying the insights to solve business problems.
You can get to that why with diagnostic analytics!
This type of data analytics helps you identify trends and determine the root causes of specific outcomes or events.
Table of contents:
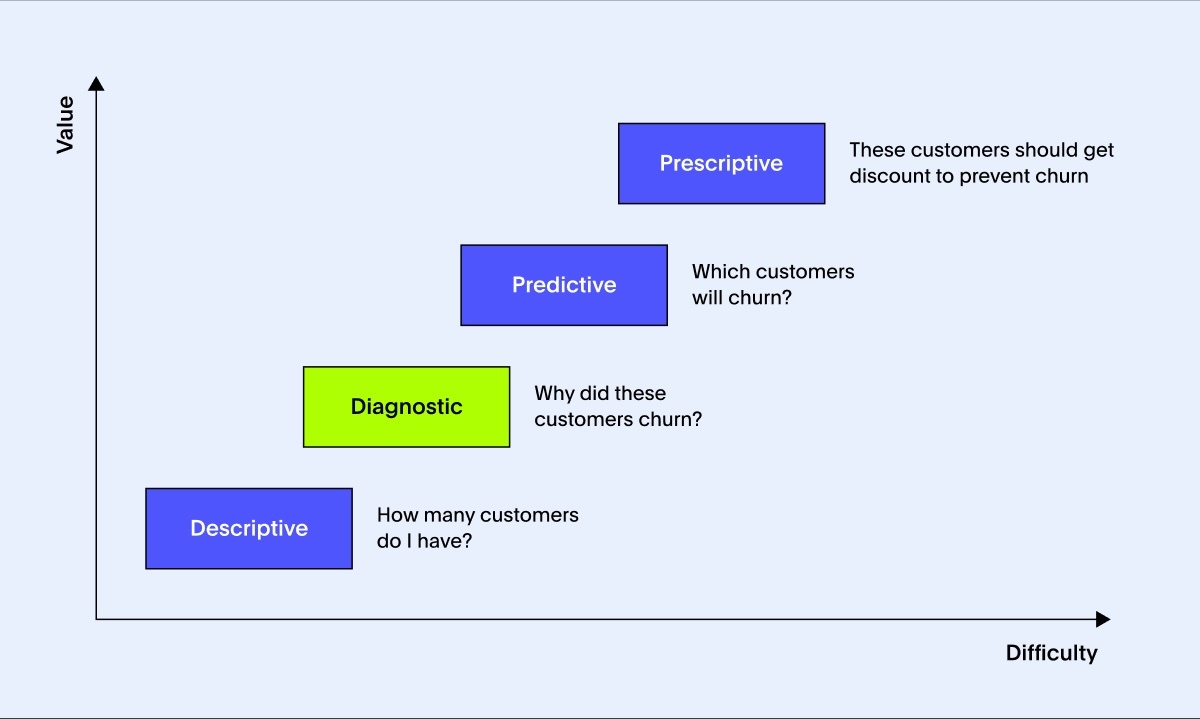
Picture this: You are a manufacturer and want to analyze your inventory performance and need optimization suggestions. Here’s how combining the different types of data analytics methods can help you glean insights from complex datasets and take action:
Descriptive analytics answers: ' What has happened with the inventory levels over the past year?'
Diagnostic analytics answers: ‘Why did specific inventory issues or fluctuations occur?’
Predictive analytics answers: ‘What will your inventory requirements be for the next quarter?'
Prescriptive analytics answers: ‘What specific actions can be taken to prevent stockouts?'
These powerful data analytics techniques serve two distinct purposes. First, they empower you to understand past events and their causes. Second, they also anticipate future trends and suggest actionable steps to meet inventory demands and reduce operational expenses.
Deciding the right type of diagnostic analytics requires an understanding of the dataset’s nature and the desired outcome. Here are some of the most common use cases for your consideration:
Hypothesis testing: This statistical method assesses the plausibility of a hypothesis by using sample data. Specifically, it helps data scientists evaluate whether observed patterns are statistically relevant.
Anomaly detection: Diagnostic analytics also help you identify unusual patterns or outliers in data. This technique essentially helps you pinpoint irregularities that might be indicative of problems or potential threats.
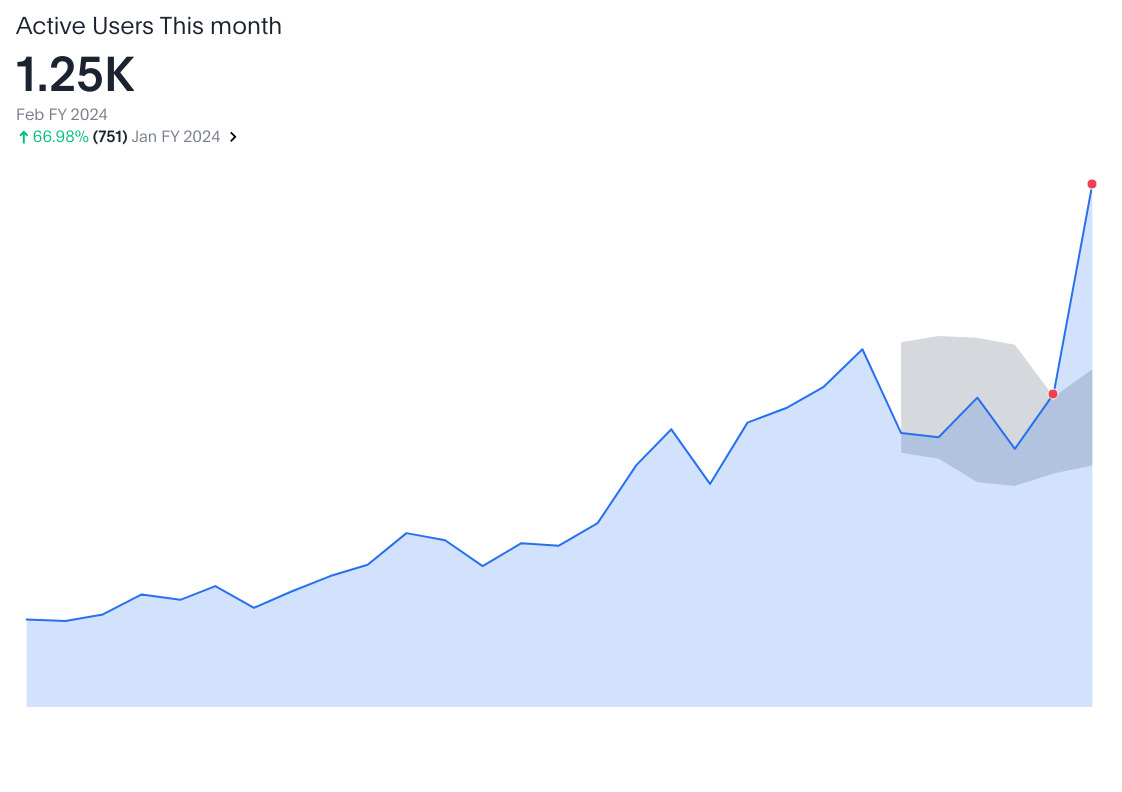
3. Root cause analysis: This technique helps you understand the root causes of problems to identify solutions. First, the process involves analyzing data to identify the origin of the problem. Second, it involves learning the cause of the problem. And finally, based on the learnings, you can take action steps to fix the problem.
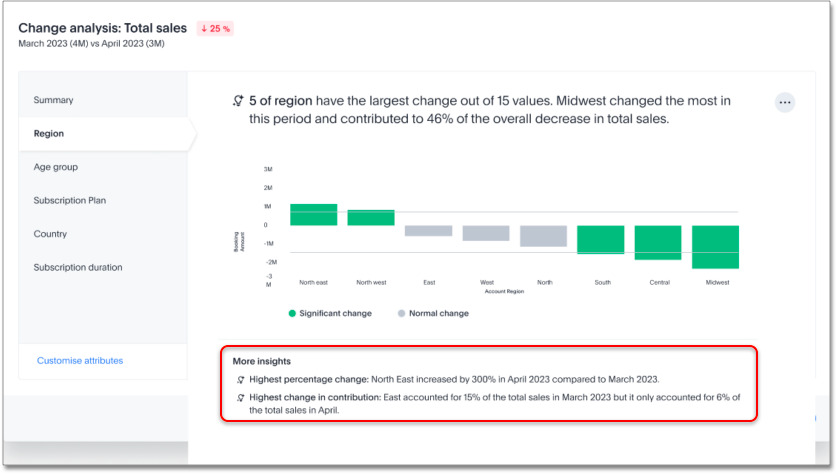
4. Correlation: Understanding the relationships between variables is important to diagnostic analytics, that’s why we use the correlation method. This method puts this analysis into practice by rigorously examining data to establish true cause-and-effect relationships.
5. Diagnostic regression analysis: Regression analysis identifies the relationship between a dependent variable and one or more independent variables. Although this method also uses advanced statistics, the intent differs from hypothesis testing. It helps you understand the impact of different factors on a specific outcome.
Now that you know the types of diagnostic analytics, let’s drill into how the entire process works. Here are seven main steps data analysts follow to ensure data contributes meaningful value to business priorities:
Define the problem: Clearly articulate the event, outcome, or business problem you aim to address. This step helps in setting boundaries for the investigation.
Collect relevant data: Identify the data you need to collect for the analysis. This data may come from various sources, including customer transactions, marketing campaign data, and other relevant information.
Process the data: Use data cleaning tools to preprocess data for accuracy and reliability. The process involves handling missing values, removing redundancies, and checking for duplicate values.
Decide the right analytics technique: Utilize hypothesis testing or regression analysis to pinpoint factors that might have influenced the probability of the event occurring.
Apply statistical methods: Use statistical techniques to understand the dataset and establish relationships between variables.
Visualize the data: Leverage data visualization tools to visualize your findings. By doing so, you can better understand the insights derived from the data to detect anomalies or outliers.
Interpret the results: Based on the insights from your analysis, create actionable strategies to address issues, optimize processes, and capitalize on opportunities.
All too often, businesses still make critical business decisions based on gut feeling and assumptions. Research by Gartner shows that 83% of percent of business strategies fail due to faulty assumptions. To avoid falling into this trap, you can leverage diagnostic analytics to see benefits like:
Enhanced visibility: Diagnostic analytics meticulously examines past data to identify the reasons behind operational bottlenecks. Empowered with these insights, business leaders can streamline workflows, optimize resource allocation, and reduce lead times.
Informed decision-making: By leveraging diagnostic analytics, you can identify crucial trends, spot anomalies, and run a root-cause analysis. This empowers you to make decisions grounded in data, taking guesswork out of the equation.
Optimized processes: Organizations use diagnostic analytics to drill down into the data and understand correlation and causation. With these insights, you can pinpoint functional areas that need further attention and implement measures to improve performance.
Happier customers: Knowing why your customers churn is a challenging task. Diagnostic analytics can help you determine which points in the customer journey are the most likely to cause churn and identify any outlying triggers. These insights can help you identify unmet customer needs and craft personalized experiences to reduce churn rates and boost revenues.
Reduced risks: Identifying risks early on is crucial for long-term business success. Diagnostic analytics help you learn from past experiences, refine risk mitigation strategies, and enhance overall risk management effectiveness. For instance, banks use this technique to analyze historical data related to credit transactions to gain an overview of customer repayment patterns and refine their existing lending practices.
1. Finance
Diagnostic analytics finds a very practical application within finance—planning and budgeting. By analyzing finance KPIs and historical data, you gain insights into business performance and dig deep into data for optimal resource allocation.
For instance, using diagnostic analytics, you can analyze past data to identify irregularities, expense patterns, or budget variances within different departments. This will help you locate the areas where capital leaks are occurring and take appropriate measures—like revising budget allocations or implementing cost-saving strategies.
2. Human resources
Research by Gallup shows that nearly 80% of employees worldwide are unengaged, indicating that they may lack a sense of connection, motivation, or enthusiasm for their work. This lack of engagement can have negative implications for productivity, job satisfaction, and overall organizational performance.
Instead of turning a blind eye to these scenarios, HR leaders can apply diagnostic analytics to understand which department has the highest attrition rate and decipher the reason behind this attrition. Using these insights, you can create strategies to address employee concerns and foster a positive work culture.
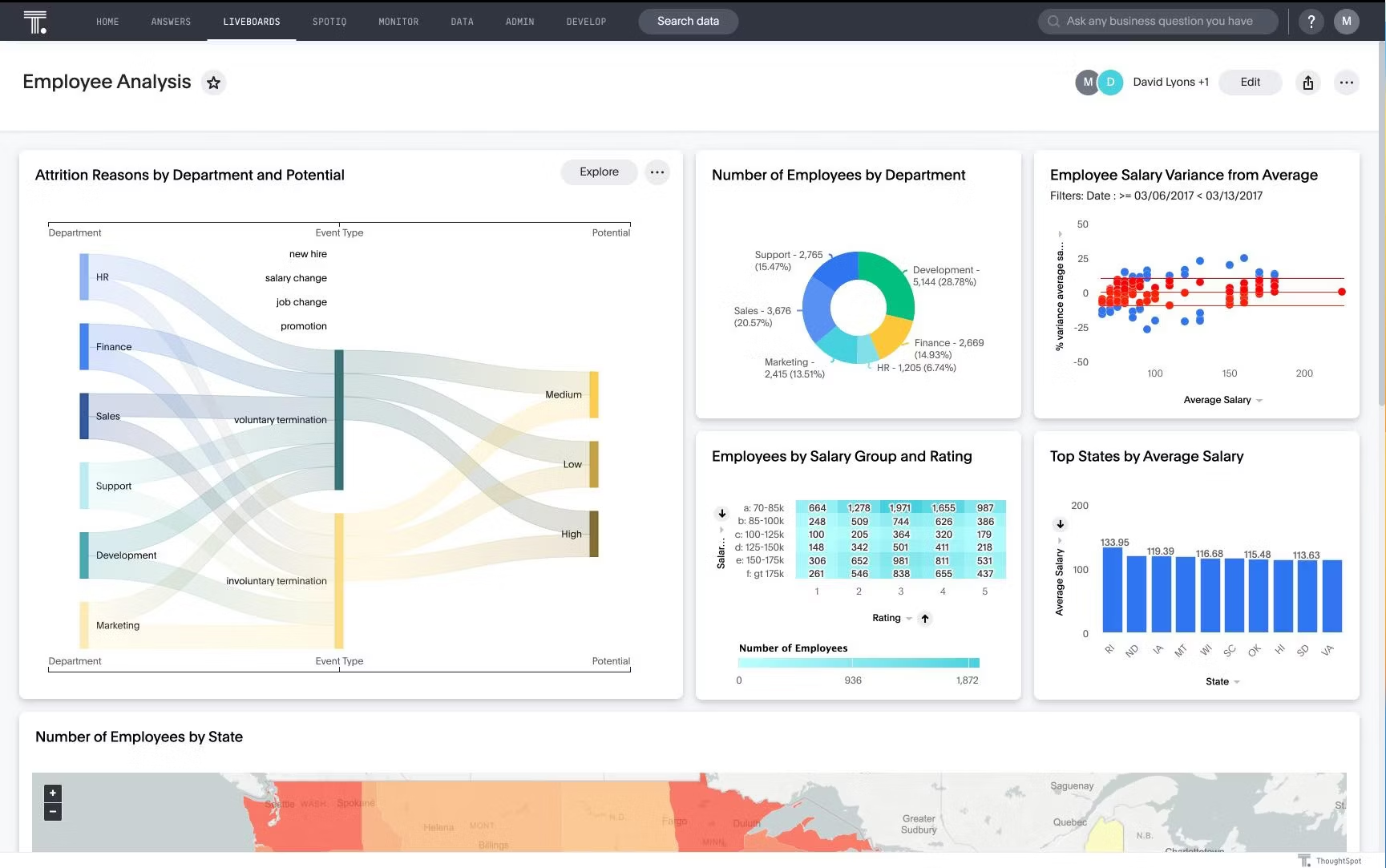
3. Digital marketing
Have you ever looked at the performance of your digital campaigns and thought, ‘I wished I could understand why my ad clicks and impressions are low’? With diagnostic analytics and interactive dashboards, you can. In this diagnostic analytics example, you can dissect the various elements of your campaigns, you can identify underperforming channels, scrutinize the impact of campaign messaging, and retarget the audience—optimizing the performance of existing campaigns.
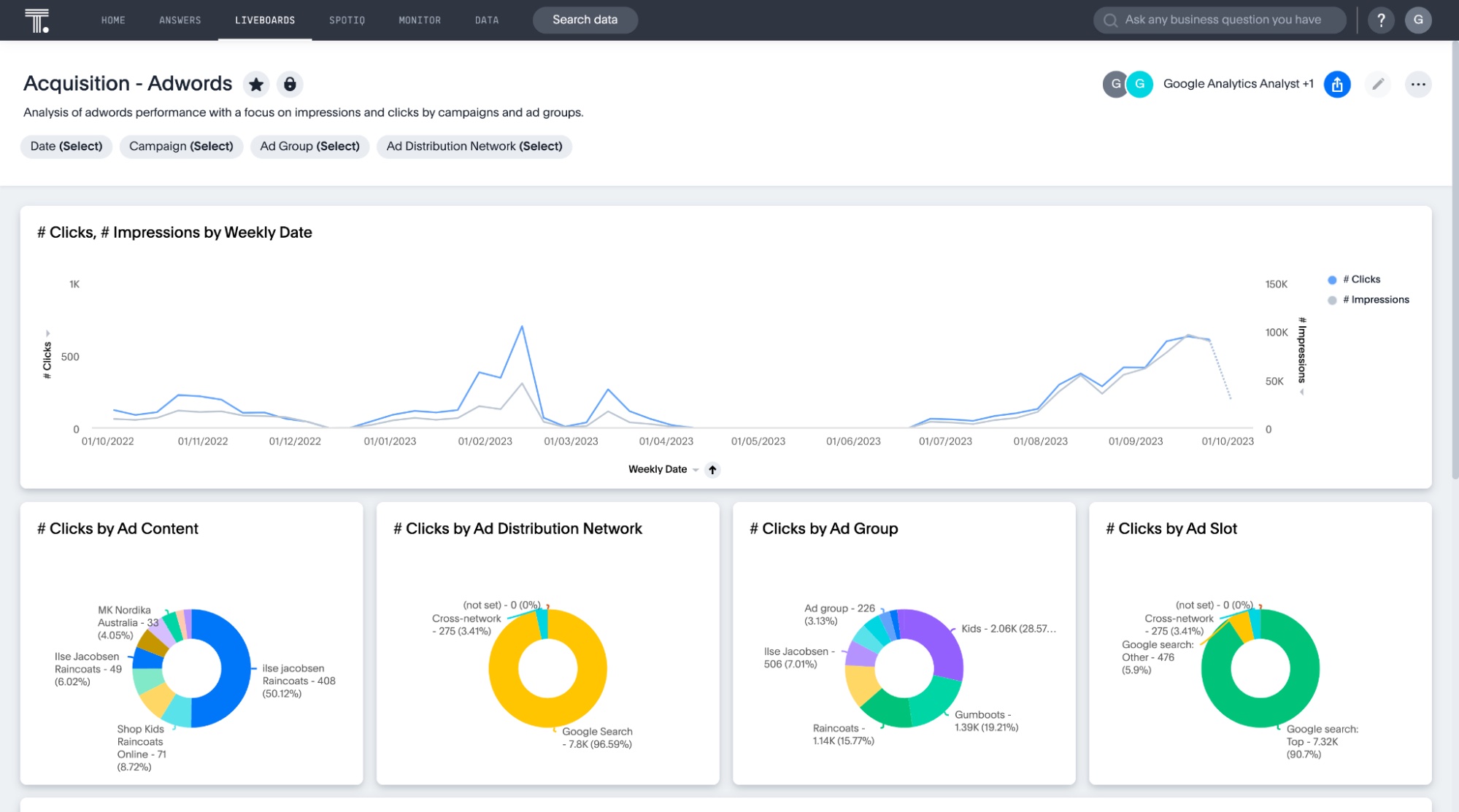
4. Marketing and sales
Another diagnostic analytics example is marketing and sales. You can analyze patterns and trends in customer behavior to identify products that are frequently purchased together. This information helps you create targeted cross-selling offers and suggest complementary products to customers based on their previous buying patterns, inevitably driving your company’s bottom line.
For instance, Canadian Tire leverages ThoughtSpot AI-Powered Analytics empowers you to identify cross and up-sell opportunities in their pets department. By using ThoughtSpot to analyze the purchasing behavior of new pet owners, Canadian Tire was able to suggest related products to these customers. These insights helped them drive sales by almost 20%.
GenAI has sparked the ‘Data Renaissance’ by closing the gap between data and outcomes. With AI-Powered analytics like ThoughtSpot’s SpotIQ, you can uncover anomalies and find hidden insights within minutes. Whether you want to identify relationships within variables or find patterns within large datasets, ThoughtSpot delivers trusted and personalized insights.
Experience how ThoughtSpot unlocks the true value of your data—schedule a demo today.