Supply chain analytics can be complex and difficult to understand for some companies. For any business producing a product or service, they must procure various raw materials and component parts, manage their inventories and production processes, and ultimately get a high-quality product to their end customer in a timely manner.
However, a supply chain is rarely static, and things can change fast. This is something we’re all keenly aware of coming out of COVID-19 induced market instability—a period that saw unprecedented supply chain failures and shipping delays across almost every industry. This situation highlights the importance of supply chain data. Those who had a view of their entire supply chain could make data-driven decisions, pulling away from their competition and driving success for their organization.
But what exactly is supply chain analytics, and how can you use them to join the ranks of successful businesses? Keep reading to find out.
What is supply chain data analytics?
Supply chain data analytics is the computational analysis of the systems and processes involved in a company’s supply chain. This involves integrating and evaluating data from all parties involved in producing a good of service—including suppliers, distributors, shipping providers, warehouses, and more.
By integrating data from various data sources such as your inventory management and procurement systems, analysts can study how materials or component parts are being sourced, assembled, transported, and ultimately provided to an end consumer as a finished product or service.
Once integrated and centralized, your data can then be processed and displayed through data visualizations. These visualizations help users understand the relationship between these systems and processes, showing what’s happening, why it’s happening, and potential actions that will make the overall supply chain more efficient.
Why is supply chain analytics important?
We’ve already discussed the complexity, unpredictability, and volatility of supply chains. With supply chain data analytics, companies can monitor and analyze these complex structures, adjusting processes and operations to optimize the supply and demand relationship with high-quality, on-time, and lean production.
For example, many manufacturing companies are dependent on raw materials that fluctuate in price and supply over time due to broader macroeconomic, environmental, and weather conditions. Due to elements like seasonality, weather, or other factors that are out of the control of the manufacturer, customer demand for the end product can also fluctuate independently of the raw materials.
Having supply chain data analytics systems in place can help businesses understand how these different supply and demand conditions affect factors such as inventory levels, delivery timeliness, and labor needs so that management can adjust operations in response to — or ideally in preparation for — these changes.
How supply chain analytics works
Supply chain data analytics is a process that involves:
Capturing data from multiple sources
Integrating that disparate data
Analyzing the results through ad hoc analyses, visualizations, and reports.
Since most supply chains involve a network of different organizations and business departments, there are typically numerous different systems that are producing relevant data.
For example: Let’s say your business orders raw materials from different providers. You will need to reconcile and link your internal inventory data with your external vendors’ various systems and the relative shipping providers. These connections will empower you to track how component parts and raw materials are flowing into your warehouses and production centers.
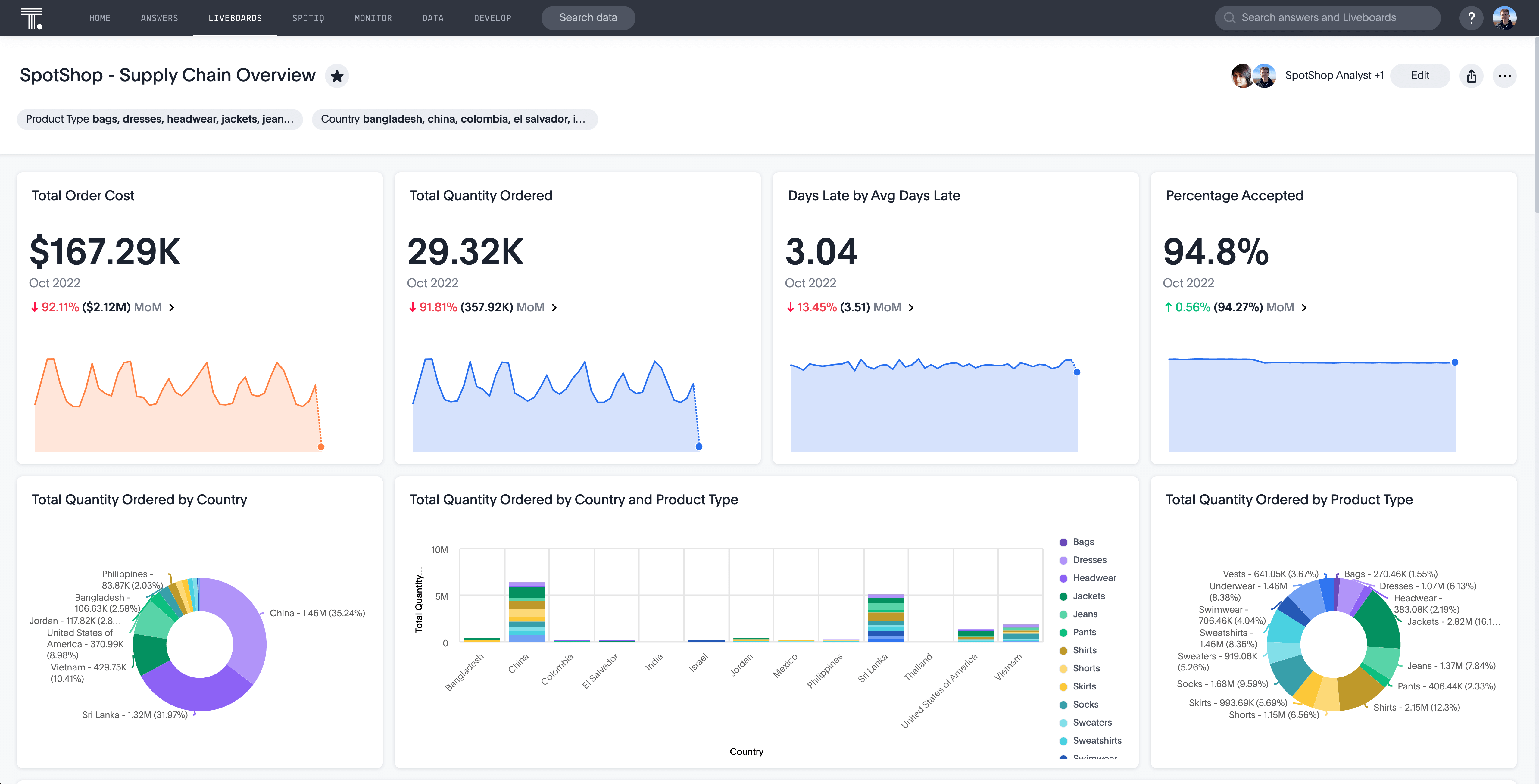
However, integrations alone are rarely sufficient for starting an analysis. In order to provide value, data needs to be cleaned, standardized, and integrated so that it can be easily queried and reported on. This is particularly the case when pulling data from different companies, as they likely have different standards for how data is structured, how products are identified, and how the data interfaces are exposed. Data processing pipelines allow businesses to take these varying standards and bring them into a consistent format for analysis.
Once the data is integrated and processed into a centralized repository, analysts can build out models, visualizations, and reports—giving supply chain professionals the opportunity to explore the data directly with modern business intelligence tools. Now that both teams have visibility into their data, they can draw inferences about the health of their supply chain.
5 types of supply chain analytics
Supply chain analytics is a broad stroke—using data to find out what happened, why it happened, and to predict the future state if you do, or don’t, take a specific action. That said, you can actually break supply chain analytics into five different types of data analytics:
Descriptive analytics
Descriptive analytics is the process of interpreting historical data. The result is often used to answer the questions “what happened?” or “what is happening?” in a business’s operations.
In the context of the supply chain, this involves understanding the historical state of everything from inventory levels to delivery times to consumer reviews. These different pieces can be viewed over time to see how they fluctuate or are changing over time.
Diagnostic analytics
Diagnostic analytics takes descriptive analytics one step further and tries to understand the relationship between various data points. More broadly, it answers the “why” of the historical data that descriptive analytics surfaces.
For example, consider if your descriptive analytics shows low inventory levels unable to meet customer demand and slow supplier deliveries, Your diagnostic analytics would try to determine if the slow deliveries caused a shortage in some key component, resulting in insufficient product production.
Predictive analytics
Predictive analytics uses statistical analysis, often computing correlations between various datasets to determine some type of causal relationship, to make projections about the future.
To continue with our supply chain example, let’s say the diagnostic analytics determined that the slow deliveries did cause the low inventory. Predictive analytics would build on key indicators from this example in order to flag potential problems in the future.
Maybe, for example, colder months of the year cause road problems that lead to frequent delivery delays. By running a statistical analysis between the delays and local weather data, analysts can determine if there’s a strong correlation between the two and decide whether it warrants a further investigation to determine causality.
Prescriptive analytics
Prescriptive analytics uses the analysis of predictive analytics to determine potential actions based on the projections. Rather than just showing the plain facts, this type of analysis draws broader meaning about the data so business leaders can solve issues before they arise.
Let’s continue with our previous example. After confirming the strong correlation between cold weather and delivery delays due to road problems, the next question is how we fix this. Analysts can run simulations with proposed solutions to see how they affect key metrics, like delivery timeliness.
Perhaps another shipping method is more reliable in the winter, or the company could boost inventory in preparation for winter delays. Data analysis and modeling can help to automate and test these scenarios before operators decide to invest in the ultimate solution.
Cognitive analytics
Cognitive analytics is the use of machine learning and AI to make sense of complex and interconnected data. This type of analytics effectively runs predictive and prescriptive analytics models on wide ranges of datasets, trying to understand relationships that would otherwise be infeasible for a human to manually experiment on.
For instance, a cognitive analytics model may try to map the delivery delay and inventory planning data from various data sources to find unexpected relationships. This analysis could uncover a strong correlation with rising natural gas prices in the winter. This discovery might lead analysts to hypothesize that the suppliers’ input costs are putting a seasonal strain on their businesses. Without being able to run these broad types of regression analyses, it would be very difficult for a human analyst to navigate these seemingly random relationships.
Features of effective supply chain analytics
While there are so many different types of businesses and supply chain structures, there are a few standard features that almost every effective supply chain analytics system has::
Data visualizations
Data visualizations are used by analysts to represent raw datasets in an easy-to-grasp, visual format. For example, bar charts, histograms, and line graphs are all examples of visualizations that you can create to show how different parts of a supply chain are operating.
Security
Supply chains consist of sensitive operational data about a business. Therefore, it’s important to use industry-standard security practices and ensure your data is access controlled.
Comprehensive analysis
Supply chains aren’t just about the raw materials and shipping providers. Any data-producing element of the process should be integrated to ensure that analyses calculate all relationships and correlations that ultimately impact strategic business decisions.
Digital modeling of the supply chain
The physical supply chain processes should be replicated in a digital modeling system—often referred to as the “digital twin”—so analysts can easily iterate on and experiment before the business makes material investments in adjusting the physical systems.
Internal and external data integration
In addition to internal integrations that pull data from things like inventory management systems, analysts should pull information from external sources that affect their supply chain, such as public weather datasets or feeds from social media APIs.
Intuitive, collaborative access
While data needs to be secured and access controlled, it should also be easy to share amongst analysts to help with the collaborative and iterative process of improving models and visualizations. In addition to increasing creativity, it’ll reduce the duplication of efforts, particularly with data pipelines that are often generalizable.
Uses of supply chain analytics
Supply chain analytics is relevant to nearly all types of businesses, as its purpose is simply to understand and drive action for operations around producing a product or service. Here we’ll explore some common examples of how businesses can leverage supply chain analytics to optimize inventory, improve production quality, reduce stockout costs, and hedge raw material costs.
Project future demand to optimize inventory levels
Consumer demand for products can vary widely between businesses. For instance, some businesses are highly seasonal and can have big spikes in different times of year or around holidays, while others may be much more consistent over time.
At scale, there’s often sufficient data to project demand into the future using statistical analyses of sales data. By doing so, the business can understand what upcoming sales forecasts may look like to ensure there’s sufficient inventory in place while keeping costs low by not producing more than is practically needed.
That was also the case for new-comer airline, Flyer. As they looked to disrupt a legacy industry, they knew that understanding and executing on demand would be the key to their success. That’s why they invested in supply chain analytics early, and that investment paid off. In just one quarter, they were able to increase revenue by 313%.
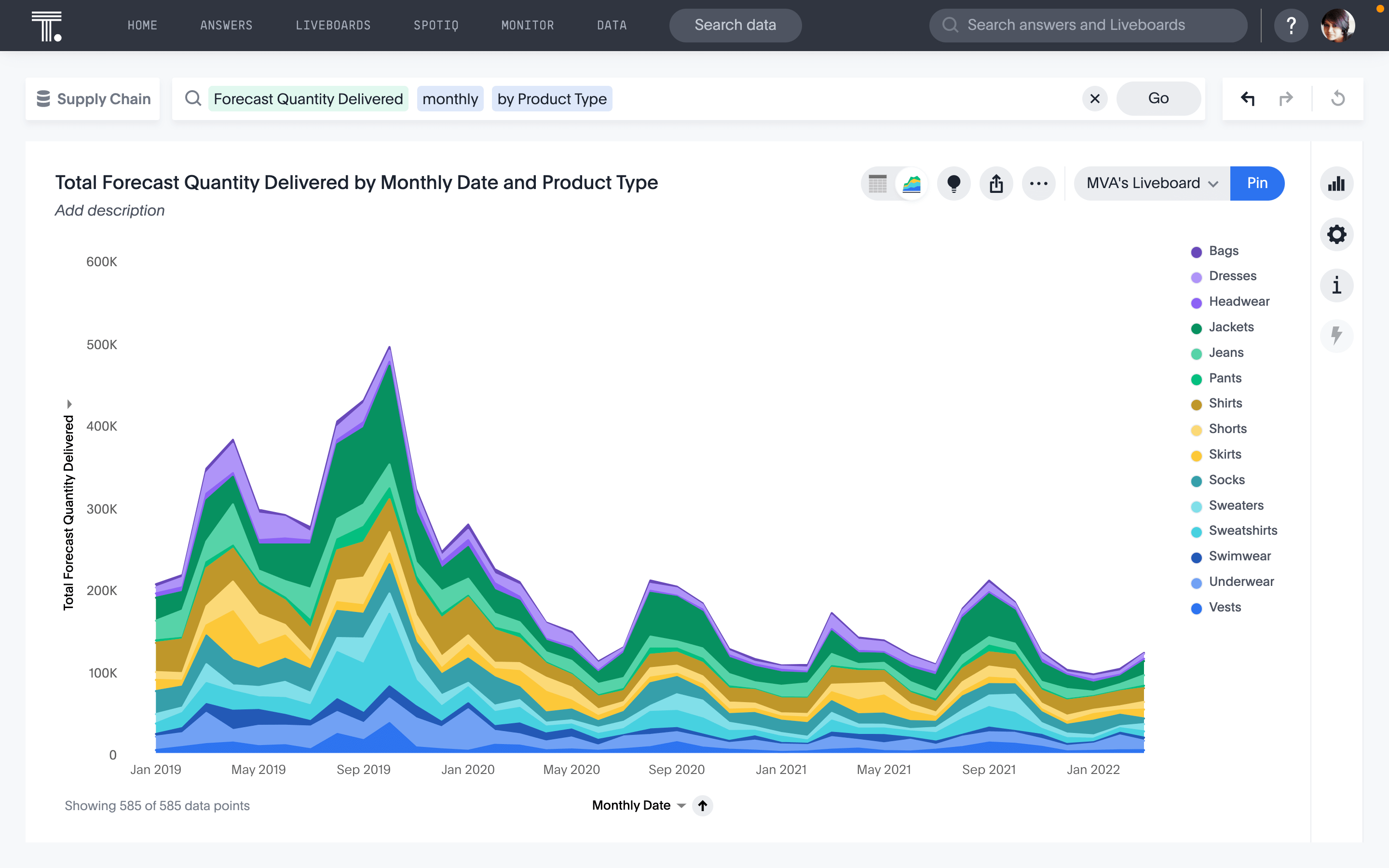
Understand production quality
End customers provide a lot of actionable data through online reviews, support phone calls, social media posts, and much more. Much of this data is available to companies to integrate and aggregate so that analysts can start to stitch together common complaints or feedback.
This customer feedback data can then be used to understand potential issues with product quality or even ways the product could be modified to better fit consumer needs. Ultimately, the end consumer’s opinion on a product or service is what matters most. So, understanding their experience is invaluable.
As a SaaS provider, you can also leverage this data as an added value for your customers. That’s the case for OrderPay, a London-based hospitality and restaurant payment solution trusted by over 2600 businesses in the UK. With inflation, soaring energy costs, price hikes, and staffing shortages, OrderPay’s customers wanted to get answers to detailed data questions about performance and customer behavior to make smart, profitable decisions.
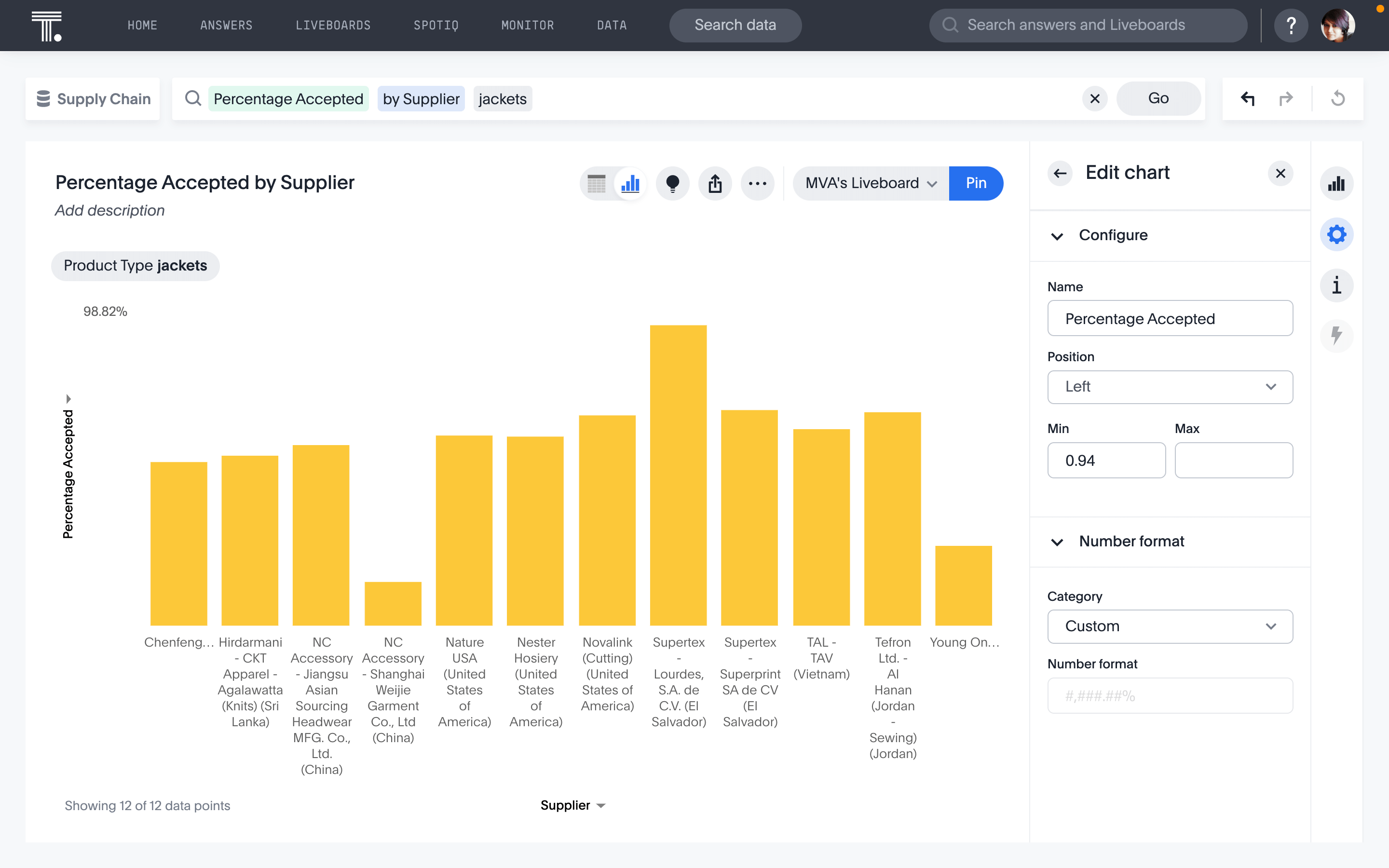
Model commodity pricing
For many businesses that rely on standard commodities like oil or grain, there can be a lot of fluctuation in pricing of necessary raw materials due to macroeconomic conditions that are out of the control of the business. Many businesses use historical data about things like prices and weather to predict what may happen to their costs so that they can hedge them with financial instruments.
For instance, it’s very common for airlines to lock-in their oil prices using futures contracts — where they commit to buy at a specified price and time in the future. While it’s certainly difficult to predict the way that a commodity is going to move, this practice has the added benefit of allowing businesses to know exactly what their costs are going to be over the duration of that contract and plan accordingly rather than being exposed to market fluctuations.
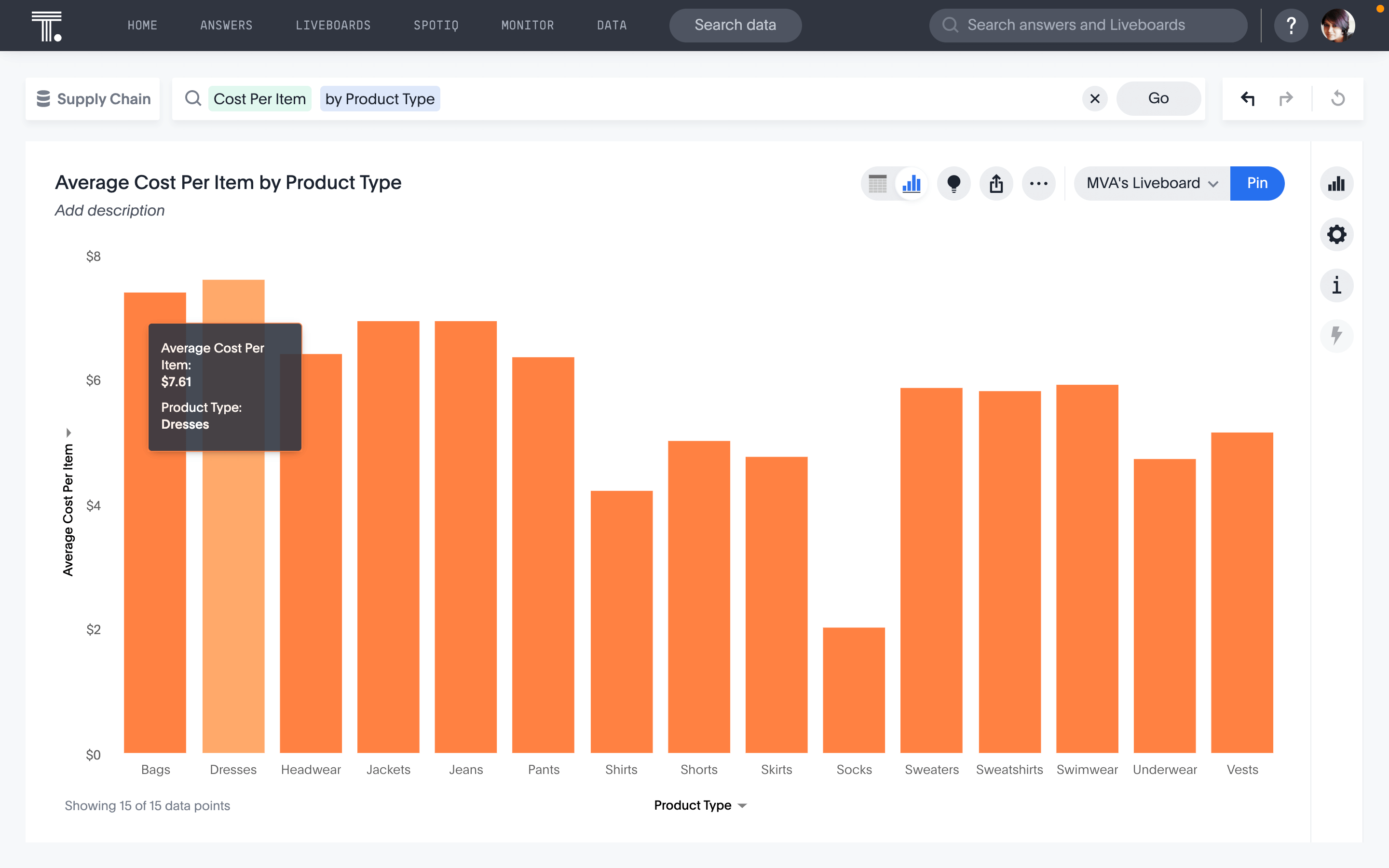
Improve your supply chain today
Supply chain analytics allows businesses to integrate and analyze all of the data related to their supply chains to better understand what is going on and how it can be improved to drive better efficiencies. However, doing so requires a robust analytic stack that can integrate disparate data sources, aggregate them using secure data pipelines, and build reports and data visualizations in a collaborative manner.
ThoughtSpot can power your supply chain analytics efforts. See for yourself—try a free trial of ThoughtSpot today.