Sales forecasting is an essential tool in any business’s sales process. Knowing your prospects’ buying patterns and predicting future demand gives you a competitive edge, allowing your team to respond rapidly when the market shifts or customer needs evolve. With the right methods of sales forecasting for your business, you can avoid missed targets and ensure there's always enough supply to meet demand.
If you want to stay ahead of the competition and maximize profit potential, it is essential to understand the nuances of sales forecasting and know how to apply 8 effective methods.
What is sales forecasting?
Sales forecasting is the process of estimating future sales revenue based on historical data, market analysis, and other relevant factors. Accurate forecasting enables businesses to adapt to changing market conditions, identify growth opportunities, and optimize their operations.
8 effective sales forecasting methods
1. Time series forecasting model
Time series forecasting models are used to project future sales based on past sales trends. These models take into account the seasonality of a product or service to better predict future demand. Essentially, a time series model is a mathematical equation that takes in data from previous periods, such as monthly or quarterly sales, and uses it to estimate future outcomes.
A common example of a time series forecasting model is an autoregressive (AR) model. An AR model is commonly used in finance analytics. It works by taking the previous values of the time series and using them to predict future values. For example, you might use an AR to predict a stock’s future price based on its past performance.
These models often fall out of favor in times of economic instability and uncertainty, like we experienced during the COVID-19 pandemic. That’s because time series forecasting models implicitly assume that the future will resemble the past. So, in the case of the AR, this type of model wouldn’t necessarily be able to account for unstable market conditions.
2. Regression forecasting model
Regression forecasting models use historical data and linear regression techniques to predict upcoming sales. This method looks at many different factors over time including: market conditions, customer sentiment, economic trends, consumer behavior, competition, and more.
These models are useful for analyzing how changes in one factor can influence another factor’s outcome. This analysis can help a company more accurately anticipate future sales and plan accordingly.
Regression forecasting models can be used across a range of industries:
These models are especially helpful in financial services, where they can be used to predict stock prices, market trends, and customer demand patterns.
For retail sales analysis, regression forecasting models can help with inventory management and marketing campaigns by predicting customer behavior and product sales.
These models can also be used in healthcare to anticipate patient demand for services, as well as predict pharmaceutical and medical device sales.
3. Historical forecasting model
Historical forecasting uses historical data to make predictions about future sales. It is widely used in retail businesses, as well as within other industries such as banking and insurance.
By analyzing past sales figures, companies can better anticipate deviations from previous performance and adjust their strategies accordingly. These projections help businesses stay one step ahead of the competition, anticipate changes in the market, and ensure they are meeting their customer's expectations.
For example, a retail business may analyze past sales figures over a period of years to identify seasonal trends or other patterns. These metrics could then be used to forecast future performance. This exercise can help that business plan inventory and staffing based on expected customer demand, taking into account anticipated shifts in consumer preferences or trends. By using historical sales forecasting methods, businesses can anticipate and plan for forecasted changes.
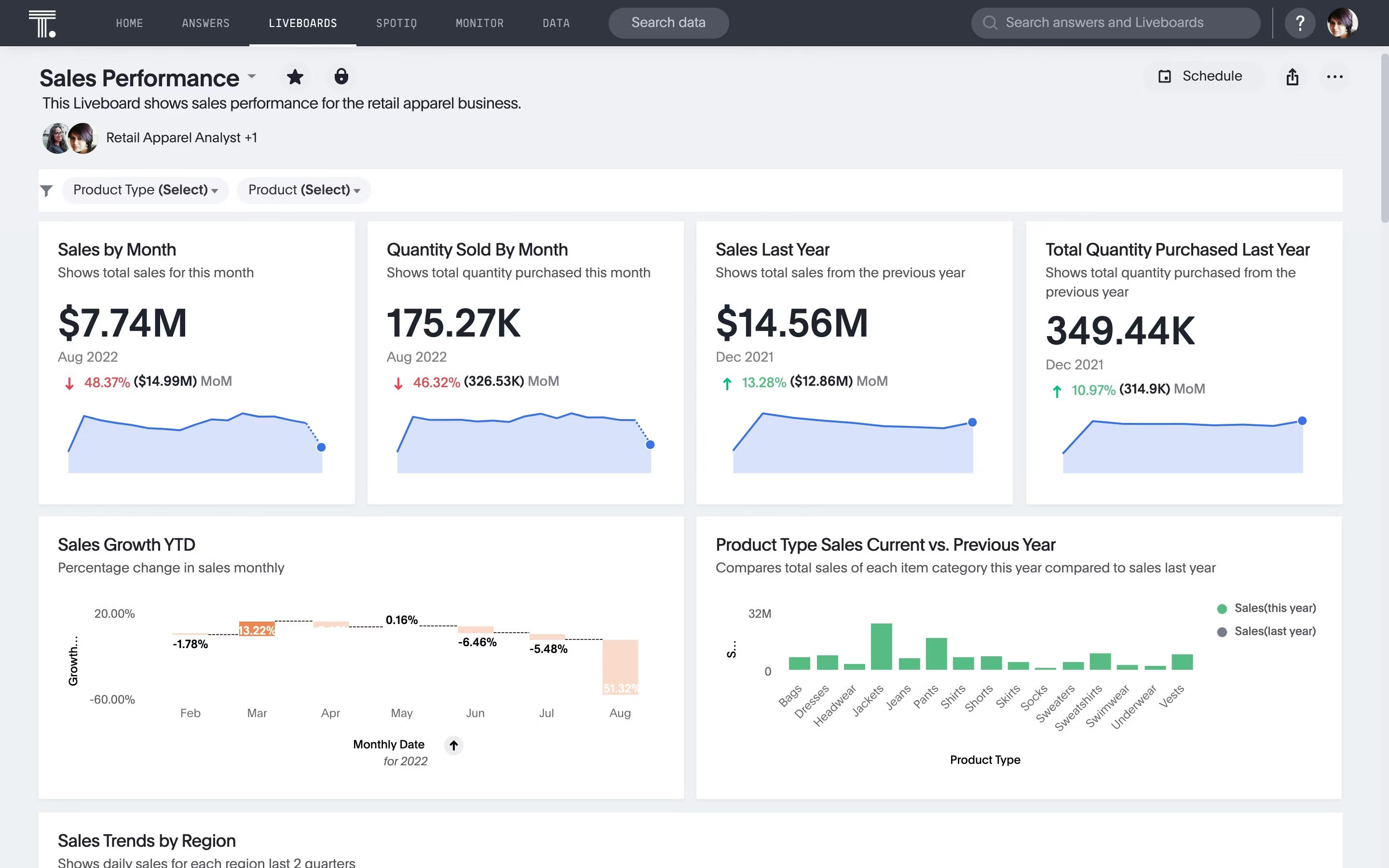
4. Opportunity stage sales forecasting model
Opportunity stage sales forecasting models use current customer pipelines and open opportunities to predict upcoming demand. These models look at factors like customer sentiment, product popularity, current buying trends, and more to provide a better understanding of what customers may be purchasing soon.
This type of forecasting model is particularly useful for businesses that sell products and services with longer sales cycles, such as manufacturing or SaaS companies.
One example of opportunity stage forecasting is using sales pipeline metrics. This involves tracking the movement of leads through each stage of the sales pipeline—from generating initial interest to closing deals. Organizations can use this data to gain insights into customer behavior and identify areas for optimization or improvement. The resulting forecasts can then be used to create better strategies and make more accurate predictions, helping you become a more data-driven business.
5. Lead value sales forecasting model
Lead value sales forecasting models use data from customer leads to predict future demand. The data can be pulled from a variety of factors such as contact information, past purchases, interests, demographics, and more. Once analyzed with the lead value sales forecasting model, this data provides an accurate estimate of which leads may be likely to convert into customers in the future.
This type of model is especially useful for predicting short-term revenue projections. By measuring a variety of variables, business leaders can gain an understanding of the forces influencing their sales. You can then leverage these factors to create more accurate forecasts.
With lead value sales forecasting, businesses can identify areas for improvement to:
Increase profitability,
Determine the optimal product pricing
Plan for changes in market conditions.
6. Length of sales cycle forecasting model
In length of sales cycle forecasting models, data from previous customers’ purchasing behavior is used to forecast the time it will take for potential customers to purchase a product or service. This model looks at customer segmentation, customer lifetime value, product popularity, geographic region, and other factors to estimate the average time it will take for customers to make a purchase.
Accurately forecasting the length of a sales cycle can be beneficial for both the buyer and seller. It allows each party to better understand the expected timeline of the process and set realistic expectations.
For example, if a buyer is expecting an immediate response from a seller, they may become frustrated if the seller takes longer than expected to respond due to other commitments. On the other hand, a seller may underestimate the time needed to close a deal if they are not aware of how long their typical sales cycle is. By understanding the length of the sales cycles, both parties can be better equipped for successful transactions.
7. Intuitive sales forecasting model
Intuitive sales forecasting is a method for predicting future performance using intuition, experience, and judgment. Therefore, it is a more qualitative approach to forecasting.
This model is often used in businesses where forecasting based on historical data may not be as reliable due to the nature of the industry or products. Intuitive sales forecasting can also be useful for making decisions about marketing spend, strategies investments, and improving product development kpis.
The most common approach to intuitive sales forecasting involves gathering insights and opinions from key stakeholders in the business before making a decision. This allows leaders to make more informed decisions by taking into account multiple perspectives. Self-service data analytics tools like ThoughtSpot helps concerned persons with proper insights so that they can take informed decisions.
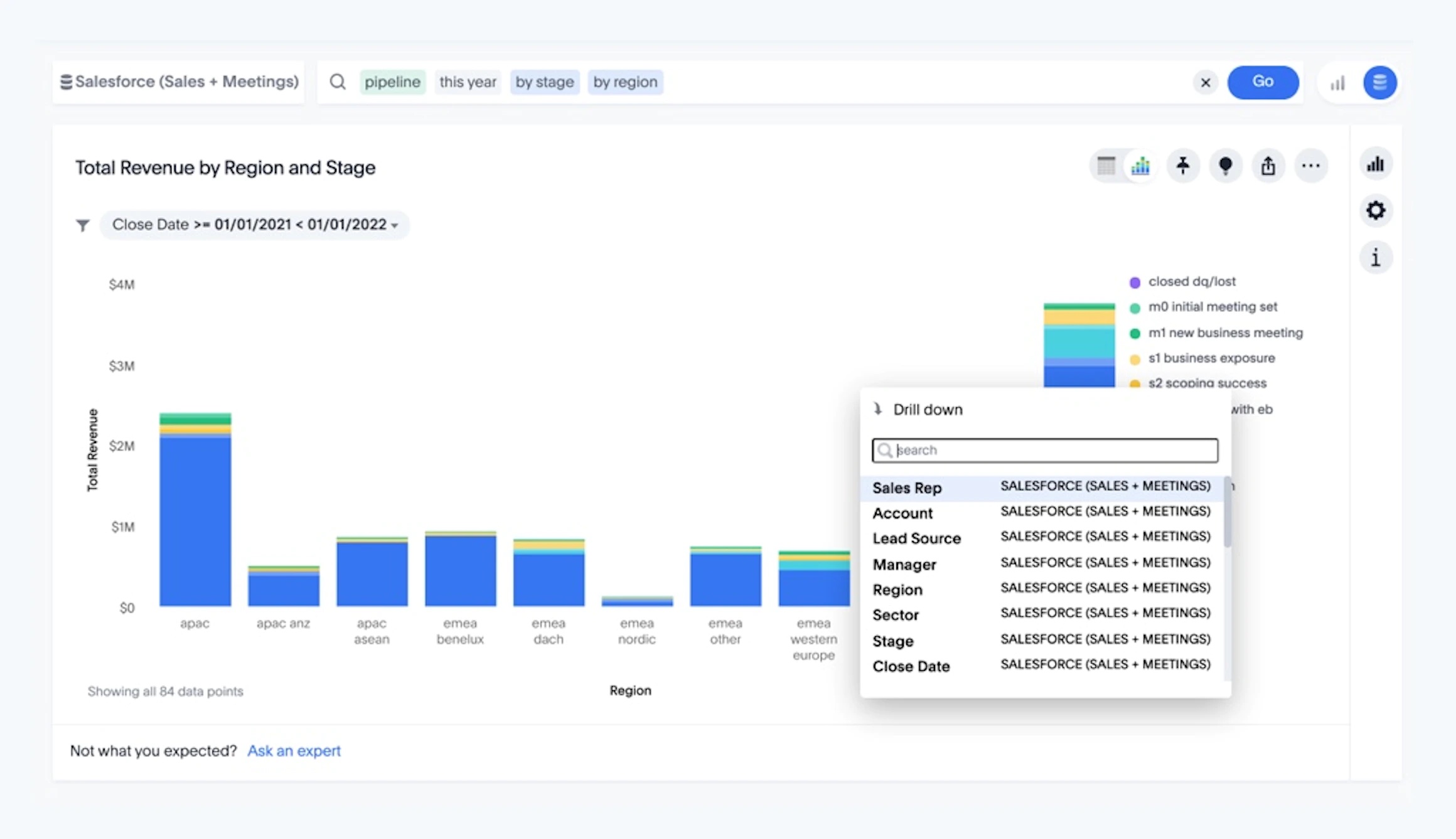
Additionally, this method can be used to gain a better understanding of customer preferences and market trends.
8. Multivariable analysis sales forecasting model
As the name suggests, multivariable analysis sales forecasting models use multiple variables to accurately predict upcoming demand. Some of the variables can include:
Customer demographics
Seasonality
Economic indicators
Marketing campaigns
Product launches
Competitor activity
By analyzing these factors together, businesses can more accurately identify correlations between different elements. These correlations help leaders make better data-driven decisions about what strategies will be most effective in driving sales.
For example, a business may find that a certain set of customer demographics have higher conversion rates, or that seasonality is an important factor for certain products. By understanding these correlations, the business can create targeted marketing campaigns or adjust product launches to capitalize on these effects and drive sales.
Easily start enhancing sales performance
Knowing the sales forecasting methods that can help your sales team boost its success can positively impact your entire business. AI-Powered Analytics are beneficial when discovering how to monetize data and maximize return on investment.
It's important to remember that forecasting doesn't only refer to predicting the future—it also includes taking action based on what is known today. So it’s important to invest in the right business intelligence platform to organize, store, and experience your data. Look for self-service analytics solutions that empower sales leaders to get hands on with the data. This will provide the most value.
While there are plenty of analytics solutions on the market, ThoughtSpot provides easy, intuitive access to clean and reliable data. Discover the value of unlocking insights and making data-driven decisions at scale—sign up for a free trial today.