You've likely encountered the terms "business intelligence" and "data analytics" in your journey through the modern business landscape. However, their interchangeable use often sparks the question: Are they truly synonymous?
Despite their shared foundation in data, business intelligence and data analytics have distinct roles. By the end of this article, you'll be well-versed in the differences between business intelligence vs data analytics with the insights needed to strategically integrate them into your business operations.
Table of contents:
Business intelligence (BI) refers to the technologies, processes, and tools that organizations use to collect, analyze, and present business data. The primary goal of business intelligence is to provide actionable insights that support better decision-making within an organization. BI involves transforming raw data into meaningful and useful information, which can then be used to identify trends, patterns, and opportunities and monitor and assess business performance.
Key components of business intelligence
Data collection: Gathering data from various sources, including internal databases, external sources, and sometimes even real-time data streams.
Data warehousing: Storing and organizing large volumes of data in a centralized repository, often known as a data warehouse, to facilitate efficient retrieval and analysis.
Data analysis: Using various analytical techniques and BI tools to examine and interpret data. This can involve querying, reporting, data mining, and other methods to extract meaningful insights.
Data visualization: Presenting data in a visual format, such as charts, graphs, and dashboards, to make it easier for you to understand complex information and identify trends.
Reporting: Creating and distributing reports based on the analyzed data to provide stakeholders with relevant information for decision-making.
Dashboard development: Building interactive dashboards that allow you to monitor key performance indicators (KPIs) and track the overall health of the business in real time.
Business performance management (BPM): Aligning BI processes and tools with organizational strategies to monitor and improve overall business performance.
Examples of business intelligence
1. Sales performance analysis:
In the context of sales, BI tools are crucial for in-depth analysis of sales data. Through meticulous examination of sales across diverse regions, product categories, and periods, the company gains actionable insights. Empowered with BI-generated reports and dashboards, sales leaders can visually grasp key metrics, uncover trends, and make informed decisions to boost sales, identify top-performing products, and refine marketing strategies.
2. Financial analytics
In the financial domain, BI tools play a vital role in crafting comprehensive financial dashboards and reports. By merging data from various sources, these tools offer a consolidated view of revenue, expenses, and overall financial health. Finance professionals can quickly identify critical areas, monitor essential key performance indicators, and make strategic decisions to optimize financial performance and ensure fiscal health.
BI tools also play a crucial role in incorporating insights related to customer churn, providing a holistic view of the financial impact of customer attrition. This integration enables decision-makers to understand revenue fluctuations, adjust forecasts, and strategize financial initiatives to counteract the effects of customer churn.
To better understand the use of business intelligence in finance, let’s take a look at a real-life scenario of Modern Restaurant Concepts. In optimizing growth at Modern Restaurant Concepts, former Finance Director Lisa Sauer streamlined reporting by adopting ThoughtSpot. Now, the Finance team is looking closely into actual sales versus forecasts, budgeting more easily, quickly course correcting, and setting more transparent goals.
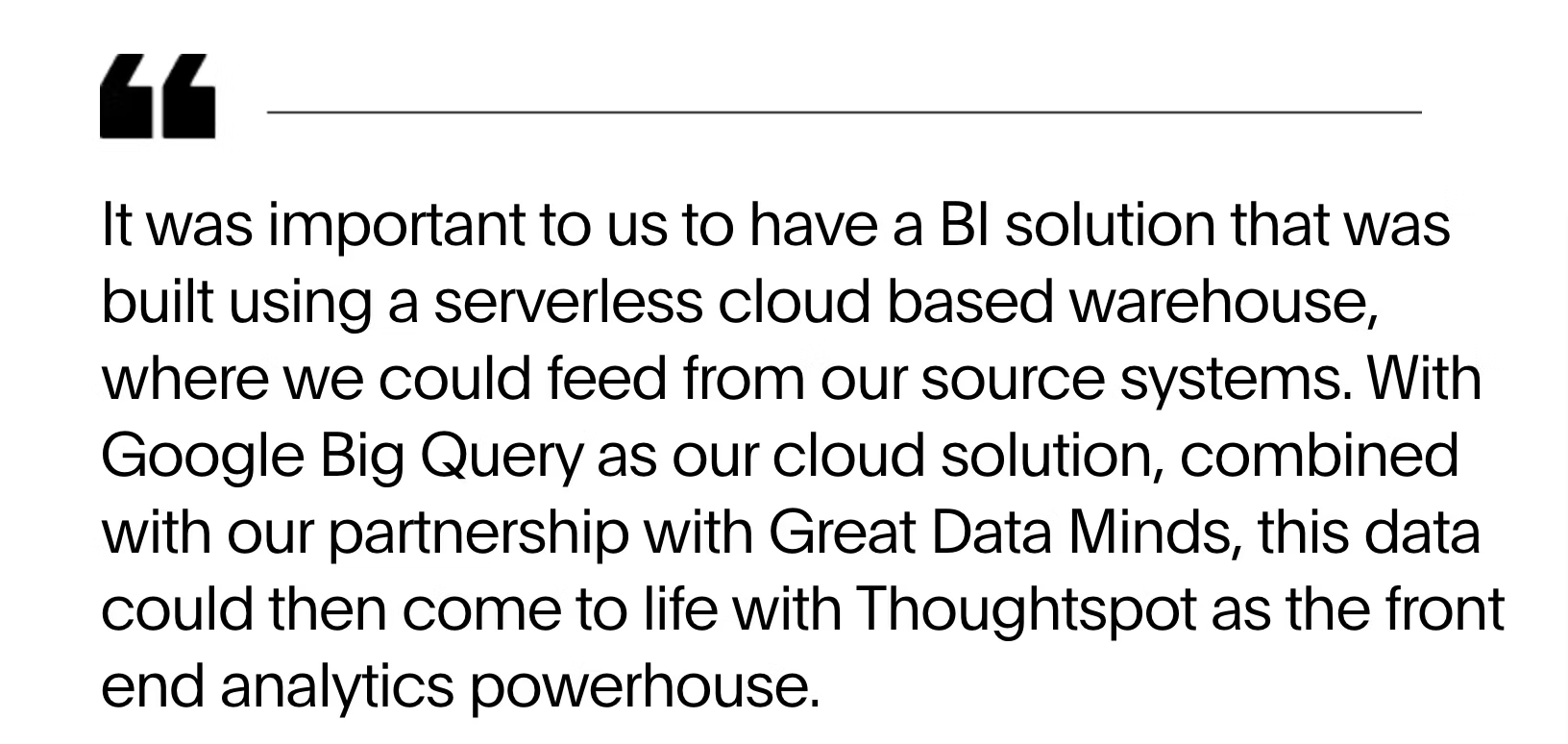
3. Supply chain optimization
Supply chain management is intricate work; BI tools are instrumental in enhancing efficiency. By tracking inventory levels, monitoring production efficiency, and identifying areas for improvement, BI empowers businesses to optimize procurement processes, empowering teams to cut costs and ensure optimal resource allocation for a streamlined and cost-effective supply chain.
Let’s take a real-world example of Fabuwood. The Azure SQL database and the traditional BI tool, Power BI, proved inadequate for Fabuwood's data team to cope with the increasing demand for business insights. Instant access to sales data was crucial for executives to make informed, data-driven decisions regarding product line profitability, supply chain logistics, and manufacturing workflows. In search of a self-service analytics solution, David Samet, Fabuwood's Director of Technology, came across ThoughtSpot. Presently, 50 employees at Fabuwood actively leverage ThoughtSpot to integrate data-driven decision-making seamlessly into their daily workflows.

4. Customer segmentation
In marketing, BI becomes pivotal for customer segmentation strategies. By dissecting customer data, including demographics, purchasing behavior, and preferences, BI tools enable businesses to craft targeted marketing campaigns. Sales teams can leverage this segmentation to tailor product offerings, improve customer engagement, and boost overall sales performance.
Ready to uncover the strategic insights driving marketing efforts? Join Katie Dunn, our Senior Demand Manager, as she reveals the power of business intelligence in marketing and how we utilize ThoughtSpot internally.
5. Employee performance analysis
BI tools are essential for in-depth analysis of employee performance. Through meticulous examination of various metrics, including attendance, productivity, and other KPIs, HR gains actionable insights. HR professionals can visually grasp key metrics, identify high-performing employees, address performance gaps, and make strategic decisions for talent management and workforce planning.
Discover the impact firsthand with insights from Alana Roesler, end-to-end data flow architect at Schneider. She reveals how ThoughtSpot empowers HR professionals to unearth real-time insights from employee performance metrics.
Data analytics is the process of examining and interpreting data sets to extract valuable insights, draw conclusions, and support decision-making. Using various techniques, tools, and methods, data analytics aims to analyze raw data, identify patterns, trends, and correlations, and convert the information into actionable intelligence.
Key components of data analytics
Data collection: Gathering relevant data from various sources, which can include structured data from databases, unstructured data from text documents, and semi-structured data from sources like social media.
Data cleaning and preprocessing: Preparing the data for analysis by cleaning and transforming it. This involves handling missing values, removing outliers, and standardizing or normalizing data to ensure consistency and accuracy.
Exploratory data analysis (EDA): An initial exploration of the data to understand its characteristics, distribution, and relationships. EDA helps identify potential patterns and trends that may inform further analysis.
Data modeling: Applying statistical and machine learning techniques to build models that can make predictions or classify data based on patterns identified during analysis.
Data visualization: Representing the results of analysis in a visual format, such as charts, graphs, and dashboards, to make it easier for stakeholders to understand and interpret the findings.
Interpretation and communication: Translating the analytical results into meaningful insights that inform decision-making is crucial. Effective communication of key findings is especially important for stakeholders who may not have a technical background. Utilizing data storytelling empowers stakeholders to grasp the significance and implications of the analytical results.
Examples of data analytics
1. Predictive maintenance in manufacturing:
In manufacturing, data analytics is applied to predict equipment failures. By collecting and analyzing sensor data from machinery, analytics algorithms can identify patterns indicative of potential issues. This proactive approach not only minimizes downtime but also maximizes the return on investment (ROI) by reducing maintenance costs and enhancing overall operational efficiency.
2. Social media sentiment analysis
Marketing teams use data analytics techniques, such as sentiment analysis, to gauge public sentiment on social media platforms. Natural language processing (NLP) algorithms analyze text data to determine whether mentions of the brand are positive, negative, or neutral. This information helps the marketing team adjust their strategies based on real-time public perception.
3. Fraud detection in financial transactions
Financial organizations employ advanced analytics to detect unusual patterns and anomalies in transaction data. Machine learning algorithms analyze historical transaction data to identify potentially fraudulent activities. Real-time monitoring helps organizations quickly detect and prevent fraudulent transactions, protecting both customers and the organization from financial losses.
4. Healthcare predictive analytics
Healthcare providers use predictive analytics to improve patient outcomes. By analyzing patient data, medical histories, and treatment responses, healthcare professionals can predict potential health issues, optimize treatment plans, and reduce hospital readmission rates. Predictive analytics contributes to more personalized and effective patient care.
5. E-commerce recommendation systems
In e-commerce, data analytics is applied to build recommendation systems. Machine learning algorithms analyze customer browsing and purchasing history to predict and suggest products that are likely to interest each user. This enhances the user experience, increases engagement, and contributes to higher conversion rates and sales.
Take, for example, the Modern Milkman which provides customers with an alternative to traditional supermarkets, substituting single-use plastics with more eco-friendly options and delivering them directly to customer’s doors. Leveraging advanced data and analytics, they can monitor the amount of plastic saved from landfills by their customers. Additionally, they empower users to delve into the details, enabling each customer to see their specific contribution to the collective environmental initiative.
Scope and purpose
BI is primarily concerned with providing historical, current, and predictive views of business operations. It focuses on reporting, querying, and dashboards to help organizations make informed decisions based on past and present data. BI helps businesses understand what has happened and what is happening in real time so they can make decisions that have real-world impacts on the business.
Data analytics, on the other hand, is a broader field that involves examining datasets to draw conclusions and identify patterns. It often includes predictive analytics, which involves forecasting future trends based on historical data and real-time analytics for immediate insights. Using a range of techniques from statistical analysis to machine learning, the goal of data analytics is to uncover insights that support decision-making.
Focus on users
BI tools are often designed for business users, executives, and decision-makers who need to monitor key performance indicators (KPIs) and make data-driven decisions. Data analytics is often performed by data scientists, analysts, and other specialists who use advanced statistical and computational methods to explore data and extract meaningful insights.
ThoughtSpot and Mode are two such platforms that cater to different user segments. ThoughtSpot primarily targets business users, providing intuitive and user-friendly interfaces for querying and visualizing data without requiring extensive technical expertise. Whereas, Mode is tailored towards data analysts and data specialists who model and manipulate the data to reveal insights that the business users can then consume.
Data sources
BI typically relies on structured data from internal sources such as business apps, databases, and ERP systems. This data then goes through an ELT/ETL process, resulting in structured data that is ready for consumption in the BI tool. Once in your BI solution, users can interact with standardized reports, dashboards, and key performance indicators (KPIs) for monitoring and analyzing business performance.
Data analytics can involve a wider variety of data sources, including unstructured and semi-structured data alongside structured data. This variety may include text documents, multimedia files, and sensor data, among others. Due to the heterogeneous nature of these sources, data analytics often involves extensive cleaning and preprocessing steps to transform raw data into a usable format for analysis. These preprocessing tasks may include data normalization, text parsing, sentiment analysis, and feature extraction.
Decision-making vs. exploration
BI focuses on utilizing structured data from internal sources like databases and ERP systems to generate clear, predefined reports and dashboards. These tools facilitate decision-making processes by providing standardized, easily interpretable information that monitors key metrics.
Data analytics involves exploratory analysis, aiming to discover patterns and trends not immediately apparent. By employing iterative and hypothesis-driven approaches, data analysis allows analysts to refine their approach based on new findings and feedback. Unlike traditional BI, which is often used to deliver predefined reports, data analytics offers flexibility and true data discovery.
Tools and technologies
BI tools are often user-friendly, with a focus on easy-to-use interfaces for generating reports and dashboards. Embedding BI tools enables business users to analyze data and extract insights without requiring advanced programming skills.
Data analytics involves a broader set of tools and skill sets, ranging from statistical software (like R and Python libraries) to machine learning frameworks (such as TensorFlow and scikit-learn). These tools empower data teams to perform more advanced analysis, including predictive modeling and prescriptive analytics, to derive deeper insights from data.
To make an informed decision on your investment, it's crucial to recognize that a strategic blend of both business intelligence and data analytics is essential for your business. If your focus is on gaining retrospective insights into performance and utilizing structured data for decision-making, BI will be your go-to tool.
That said, incorporating data analytics is a vital component of achieving accurate, meaningful insights and real-time analytics from your diverse and ever-expanding data sources. This integrated approach positions your business to drive innovation and make strategic decisions effectively in a dynamic environment. That’s why we believe BI and data analytics are better together.
If you want to opt for a hybrid approach, combining the strengths of both business intelligence and data analytics, ThoughtSpot stands out as a modern solution that seamlessly integrates these two disciplines. Through ThoughtSpot's self-service analytics features, every business user gains the ability to delve into data, unearth valuable insights, and integrate data seamlessly into their decision-making process.
With our diversified product offering through Mode, our solutions also provide significant benefits to the data team—offering a versatile platform that caters to both code-first and code-free users. This unique combination empowers both business users and data teams to collaborate effectively and efficiently. Here are a few points that set ThoughtSpot apart:
Data visualization: See your data in a whole new way with Liveboards. Create interactive dashboards and visualizations that make your data easy to understand and act on.
AI-driven search: Ask questions and get answers effortlessly using natural language search with ThoughtSpot Sage.
Data connectivity: Connect to all your data sources, like warehouses and cloud stores, with ThoughtSpot. Your data team can start searching for live data quickly.
Data modeling: You can establish governed, reusable, and logical data models, facilitating the generation of insights through AI-Powered Analytics. Using AI-assisted data modeling, ThoughtSpot Sage also automates the generation of suggested synonyms to optimize the search experience.
Collaboration and sharing: Share your insights, reports, and dashboards with your team, Additionally, ThoughtSpot's note tiles allow the inclusion of important directions for analyzing or using specific visualizations.
Multi-modal experience: Boost user engagement through a versatile approach to data exploration. This includes real-time mobile monitoring, seamless integration with workplace apps like Salesforce and Google Sheets, and the ability to push insights back to workforce applications such as Slack.
The landscape of business analytics is evolving, and understanding where to invest in your data and business intelligence strategy is a highly nuanced decision. Through all this, ThoughtSpot and Mode present a dynamic solution, offering an integrated experience that goes beyond traditional constraints. It's not just about analyzing the past or preparing for the future; it's about unlocking the value of your data.
Join a live demo of ThoughtSpot and embrace a new era of AI-Powered Analytics.