With rising pressure to act fast and stay ahead, today’s teams are drowning in dashboards, KPIs, and “insights.” But insight without action? That’s just noise.
Here’s the hard truth: Just knowing the numbers isn’t enough for decision-making. You need real-time, actionable insights that actually drive outcomes.
Understanding what happened or even what might happen doesn’t automatically tell you what to do next. And in high-stakes environments, guessing your way through a decision won’t cut it.
That’s where prescriptive analytics comes in. It goes beyond forecasting to recommend specific actions based on your data, business goals, and real-time context.
In this guide, we’ll break down what prescriptive analytics actually means, how it compares to other types of analytics, and how modern teams are using it to move faster, act smarter, and close the gap between information and execution.
Table of contents:
- What is prescriptive analytics?
- Comparing the different types of data analytics
- Predictive vs. prescriptive analytics
- What are the benefits of prescriptive analytics?
- Prescriptive analytics examples
- How does prescriptive analytics work?
- What are the common challenges with prescriptive analytics?
- Prescriptive analytics, reimagined for the AI era
Prescriptive analytics is a type of advanced data analytics that recommends specific actions based on real-time data, business goals, and potential outcomes. It’s like your data’s personal strategist, answering the question: “What should I do next?”
While descriptive analytics tells you what happened, and predictive analytics tries to guess what might happen, prescriptive analytics goes a step further. It suggests specific actions you can take and shows the possible outcomes of each choice.
These days, everyone talks about data, but most companies aren’t really using it to its full potential. Accenture found that only about one in five businesses actually unlock the true value of their data.
That’s because just knowing the facts isn’t enough; you need real-time, practical advice that helps you make decisions and get results. That’s exactly what prescriptive analytics offers.
Using advanced algorithms and machine learning, prescriptive analytics points you toward the best moves to make based on your goals. Let’s break it down.
To understand how prescriptive analytics fits into the bigger picture, it helps to see how it builds on the other types of analytics, each one answering a more complex (and more valuable) question.
Let’s say you’re trying to understand and reduce customer churn. Here’s how each analytics type contributes:
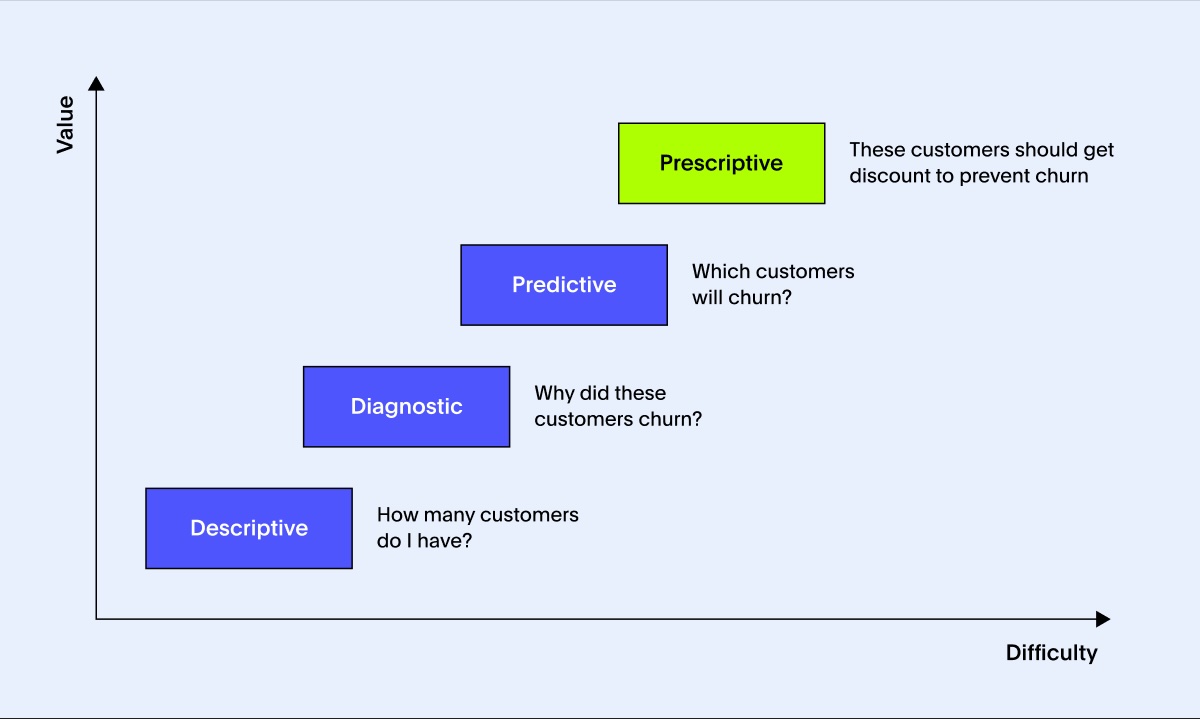
Descriptive analytics
Answers “How many customers do I have?”
It helps you track overall customer counts and churn rates over time, useful for spotting trends and understanding the scope of the issue.
Example: In Q1, you gained 2,850 new customers and lost 720, ending the quarter with 18,300 active customers.
Diagnostic analytics
Answers “Why did these customers churn?”
It digs into the reasons behind the numbers like usage drops, pricing issues, or support delay,s so you can understand what’s driving churn.
Example: After reviewing onboarding data, you find that 62% of the 720 churned customers reported unresolved issues within their first two weeks.
Predictive analytics
Answers “Which customers will churn?”
It uses historical data to identify patterns and predict who’s at risk, allowing teams to anticipate problems before they happen.
Example: Your model flags 950 customers who had fewer than two logins in their first month and haven’t interacted with support as high churn risks for Q2.
Prescriptive analytics
Answers “What should we do about it?”
It recommends specific actions like offering discounts or personalized outreach to retain those high-risk customers and avoid revenue loss.
Example: The system suggests offering a loyalty incentive to the top 400 at-risk customers and assigning customer success reps to follow up with the rest.
Each type plays a distinct role, but when used together, they help you move from reactive to proactive decision-making.
It’s easy to lump these two together. Both go beyond describing what happened and why, and both aim to guide future decisions, but they solve fundamentally different problems.
Predictive analytics is about foresight. It uses historical data, trends, and machine learning models to forecast what might happen. For example, if you're running a subscription business, predictive analytics might highlight which customers are at high risk of churning based on behavior signals like decreased usage or delayed payments.
This is incredibly valuable, but only half the story.
Prescriptive analytics takes those predictions and adds a layer of decision intelligence. It tells you what to do about the insight. Building on the churn example above, prescriptive analytics might simulate the impact of different retention strategies, weigh the cost of each one, and prioritize actions based on your goals—whether that’s reducing churn, protecting margins, or improving customer satisfaction.
It can even simulate different scenarios, weigh trade-offs, and suggest the most effective action based on your goals, whether that’s minimizing churn, maximizing revenue, or balancing the two.
In short:
Predictive = "Here’s what’s likely to happen."
Prescriptive = "Here’s what you should do about it."
Why does this distinction matter? Because a forecast without a clear path forward still leaves the decision-making burden on your shoulders. Prescriptive analytics bridges that gap by helping you act, not just react.
And in fast-paced, high-stakes environments, that difference is what separates missed opportunities from confident, timely decisions.
💡Relevant read: Predictive analytics vs. machine learning: How to leverage both?
Making decisions in today’s fast-paced world is tough. Economic uncertainty, rising customer expectations, and endless streams of data can leave even experienced teams flying blind. That’s where prescriptive analytics comes in. It doesn’t just predict what’s next—it guides your next move with confidence.
Here’s how it cuts through the noise:
Real-time, actionable insights
Makes complex data simple
Gives you the full picture
Speeds up decision-making
Reduces bias and guesswork
Aligns decisions with business goals
1. Real-time, actionable insights
Forget waiting around for static reports or lagging dashboards. Prescriptive analytics processes fresh data as it flows in and suggests next-best actions you can take immediately, like rerouting a delivery to avoid disruption, optimizing ad spend mid-campaign, or flagging a price change to stay competitive.
2. Makes complex data simple
Data comes at you fast and from every direction: sales, customer support, web traffic, supply chain, and more. Analyzing all of it manually isn’t realistic. Prescriptive analytics uses algorithms to sift through the chaos, spotting relationships and trade-offs that would be impossible to see otherwise. You get clear, context-aware recommendations you can actually act on.
3. Gives you the full picture
Prescriptive analytics doesn’t stop at one recommendation. It runs simulations, models different what-if scenarios, and lays out multiple paths you could take, often paired with interactive visualizations that show the likely outcomes of each. Instead of reacting to data in isolation, you get a side-by-side view of your options and the impact each one could have.
4. Speeds up decision-making
When stakes are high, long deliberation isn’t always a luxury. Prescriptive analytics helps you make smarter calls faster. By automating the heavy analysis and narrowing your choices to the best few, you spend less time overthinking and more time doing.
5. Reduces bias and guesswork
Even the most experienced decision-makers can fall into patterns. Favoring the familiar, playing it safe, or leaning too hard on instinct. Prescriptive analytics brings data-driven objectivity to the table. It’s not replacing judgment, it’s grounding it in real, explainable logic.
6. Aligns decisions with business goals
Every recommendation from prescriptive analytics is made with context: your KPIs, constraints, and goals. Whether you're focused on reducing costs, growing revenue, improving customer satisfaction, or hitting SLAs, prescriptive analytics tailors its suggestions to fit what actually matters to your business.
Let’s take a look at how Austin Capital Bank puts prescriptive analytics into action. With ThoughtSpot, they were able to democratize data access, improve decision-making in real time, and ultimately boost customer retention by 15% while increasing profit margins by 30%.
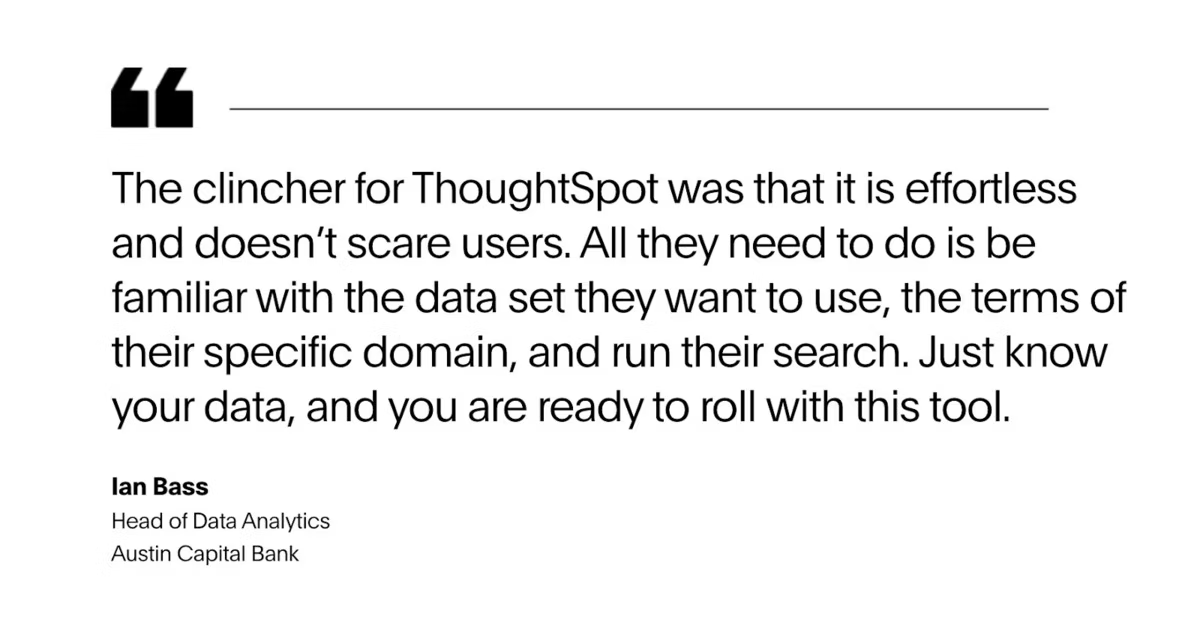
Let’s discover how prescriptive analytics and AI-powered insights are driving data-driven decisions across different industries with real-world examples:
1. Finance services
Financial institutions are always on the hunt for smarter, faster ways to serve their customers. With prescriptive analytics, teams can analyze market behavior and customer data to spot gaps and steer strategic choices.
Loan Market Group, for example, used ThoughtSpot Embedded to give over 4,000 brokers real-time insights and interactive reports. By connecting ThoughtSpot with Snowflake and dbt, they delivered a reimagined reporting experience in under three months.
2. Healthcare
Prescriptive analytics supports better patient care while controlling costs. It compares treatment paths, highlights cost-saving opportunities, and supports more personalized care plans. Hospital leaders can also spot operational inefficiencies and make targeted improvements.
MDAudit leaned on ThoughtSpot to rethink healthcare compliance and revenue integrity. More than 1,000 users could access their own insights, contributing to 25 %+ business growth in 2023. Teams improved audit outcomes, streamlined revenue processes, and made faster decisions. in 2023. Healthcare providers optimized audits, streamlined revenue, and made decisions with ease, improving both outcomes and efficiency.
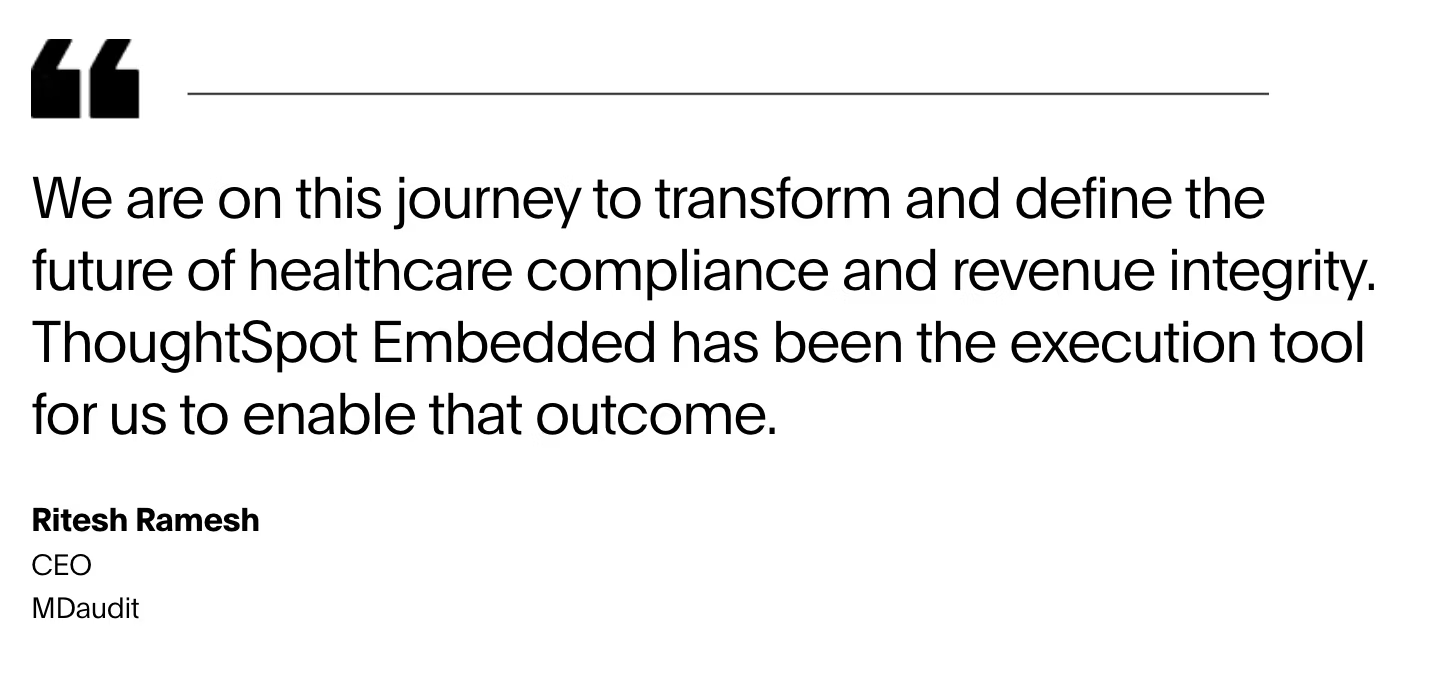
3. Marketing
Marketing teams often juggle scattered data and shifting customer behavior. Prescriptive analytics helps decode what’s working, where to focus, and how to refine your next move.
At Frontify, teams used ThoughtSpot to dive into campaign data and quickly spot performance patterns. Just five months in, 75 users had self-serve access to insights, helping them fine-tune lead generation and improve ROI.
“It’s a super tool for us to be able to visualize our data, KPIs, our overall funnel, and make smarter decisions.”
4. Manufacturing
Prescriptive analytics gives manufacturers a way to get ahead of costly breakdowns, off-track forecasts, and supply chain headaches. It helps teams understand what’s driving problems and how to fix them fast.
Fabuwood faced slow reports and clunky tools that held them back. After adopting ThoughtSpot, they tapped into real-time insights across sales and manufacturing workflows. That shift drove a 300% increase in query efficiency and led to sharper, faster production decisions.
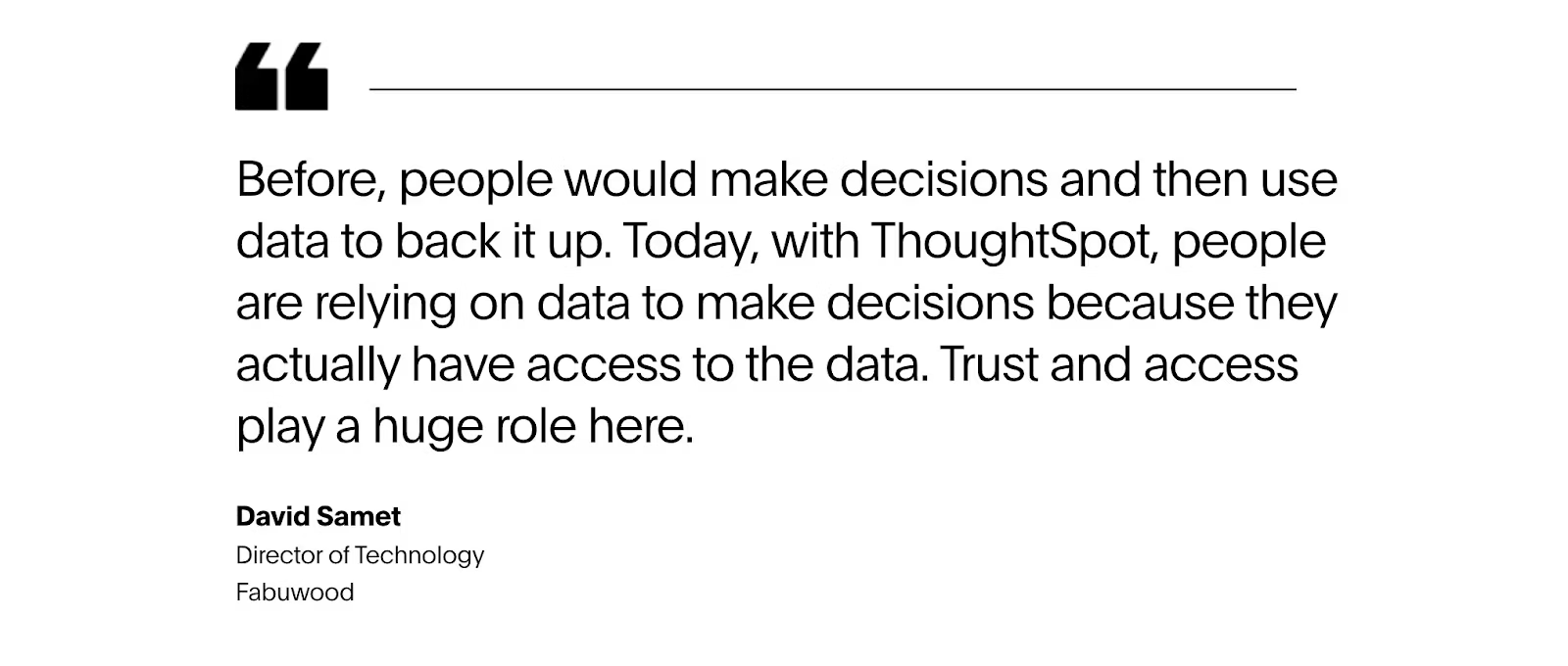
5. Banking
Banks are using prescriptive analytics to stay ahead of fraud and manage risk. These models spot unusual behavior, simulate possible scenarios, and recommend the best response.
Northmill moved from a sluggish legacy BI tool to a modern, self-serve platform with ThoughtSpot and Snowflake. The result? Better customer conversion, faster decision-making, and deeper insights into where users drop off.
“What moves the needle is turning insight into actions. To run a business, the ability to produce nice graphs and monitor interesting data is not even half the story—it’s what you do with it that’s important.”
While traditional BI tools focus on reporting and visualizing data, prescriptive analytics uses machine learning and AI algorithms to analyze large datasets and generate actionable outcomes. These outcomes help you decide the best course of action for your business goal. While this may sound simple in theory, it’s complex to deploy the model without the right tools in place.
Step 1: Define the problem
Step 2: Gather the data you need
Step 3: Develop the model
Step 4: Test and train the model
Step 5: Deploy the model
Step 6: Map outputs to outcomes
Step 7: Adjust and monitor
Step 1: Define the problem
Start by identifying the problem you want the model to address. You should also include defining the decision variables, constraints, and other relevant factors required to generate an actionable output.
Step 2: Gather the data you need
Make sure the data feeding your model is accurate, complete, and relevant. To do this, you need to remove data with missing values, include external information, and label the datasets clearly.
Step 3: Develop the model
Use the cleaned data to develop a model tailored to your objective. You’ll need coding and analytical skills to structure the logic and integrate the right machine learning algorithms.
Step 4: Testing and training
Run your model and watch for issues like data duplication or inconsistencies. You should adjust the parameters as needed to optimize performance and ensure accuracy.
Step 5: Deploy the model
Put your fully tested model into production. You should integrate it into your business systems and workflows so the recommendations become part of day-to-day decision-making, not just insights on the side.
Step 6: Map outputs to outcomes
Create a process that links model recommendations to your strategic priorities. This helps you ensure the insights directly support the outcomes you care about most.
Step 7: Adjust and monitor
Track results, gather feedback, and refine your model over time to keep it aligned and effective. After all, prescriptive analytics isn’t a ‘set it and forget it’ approach.
Prescriptive analytics sounds great in theory, but getting it right takes more than just plugging in a model. Here are some common hurdles businesses run into:
Data quality and availability
Complexity of models
Change management
Balancing automation and human judgment
Costs and resources
Keeping models up to date
1. Data quality and availability
Prescriptive analytics depends heavily on clean, reliable data. If your data is incomplete, outdated, or inconsistent, the recommendations you get might be off—or even misleading. You’ll need to invest time in cleaning, integrating, and governing data from multiple sources.
2. Complexity of models
Building and maintaining prescriptive models isn’t just about advanced algorithms or machine learning—it also takes real data science expertise and a deep understanding of your business. Without that mix, it’s tough to build models that work consistently or evolve with your needs.
3. Change management
Even the best recommendations don’t do much if teams don’t trust or use them.To succeed, you’ll need to shift how decisions are made, get buy-in from stakeholders, and integrate analytics into daily workflows.
4. Balancing automation and human judgment
Prescriptive analytics can suggest actions based on data, but it can’t replace human insight. Striking the right balance between trusting automated guidance and applying your own experience is crucial—and sometimes tricky.
5. Costs and resources
Deploying prescriptive analytics tools and maintaining them takes investment. From software licenses to hiring talent, the upfront cost and ongoing resources needed can be a barrier for some organizations.
6. Keeping models up to date
Markets, customers, and conditions change fast, and models need continuous monitoring and updating to stay relevant. Without this, your prescriptive analytics can quickly lose accuracy and value.
Great decisions don’t come from dashboards alone; they come from understanding what to do next. And that requires more than static charts or backward-looking metrics. You need analytics software that can model data in real time, simulate outcomes, and guide your next move, not just highlight past trends.
That’s exactly where ThoughtSpot shines. With Spotter, your AI Analyst, teams can move from insight to action faster and with greater impact.
Join the ranks of successful businesses that build customer-oriented products with data. Book your free demo today!
FAQs
1. What’s the difference between prescriptive and predictive analytics?
Predictive analytics forecasts what might happen based on historical data. Prescriptive analytics takes it a step further by recommending specific actions based on those forecasts. While predictive models might flag churn risks, prescriptive models suggest what to do about them, like offering a discount or improving onboarding.
2. What kind of data do I need to get started with prescriptive analytics?
You’ll need clean, reliable data, ideally historical and real-time, from across your business systems. This includes sales, marketing, customer behavior, support tickets, and operational data. The better your data quality, the more accurate your models and outcomes will be.
3. Can prescriptive analytics be used without a data warehouse?
It’s possible, but not ideal. A cloud data warehouse helps you centralize, clean, and scale data, making it easier for analytics platforms to model data and generate accurate, actionable insights.
4. What’s the difference between AI-driven insights and prescriptive analytics?
AI-driven insights surface patterns or anomalies. Prescriptive analytics takes it further by turning those insights into recommended actions based on your goals and constraints. It’s about “what now?” not just “what happened?”