As the digital economy becomes the economy, companies leading with analytics are outpacing competitors, seeing revenue grow while their peers remain flat or contract. Leveraging data to inform decisions across the organization is key to winning in today’s business landscape.
As data volumes explode, however, the most successful companies are breaking from the business analytics paradigms of the past. Instead of relying on pixel perfect dashboards painstakingly curated by a team of data experts, companies leading their industries are using AI analytics to go further and empower everyone with data-driven decision making. From natural language search and predictive capabilities to generative AI explaining insights as they emerge, the applications for AI in the world of data promise to fundamentally change how companies understand, measure, and act on their business. Doing so, however, requires understanding the vast potential of this technology, how it works, and potential benefits and use cases. In this post, we'll look at the definition of AI analytics, the four core pillars you should be aware of, and how to take this from experimental tech into real business use cases.
Table of contents:
AI analytics is an emerging field that brings together the world of artificial intelligence and machine learning with analytics to generate insights, automate processes, deliver predictions, and drive actions that lead to better business outcomes. By combining AI with business intelligence, AI analytics give organizations a more comprehensive view of their operations, customers, competitors, and the market. Users not only understand what happened, but why it happened, what’s likely to happen next, and what might happen if a particular course of action is taken.
With AI data analytics, companies can manage every facet of their operations more effectively—from predicting customer behavior to identifying hidden opportunities. These insights can inform strategies to optimize performance and stay ahead of competitors.
AI analytics is important because it enables organizations to gain insight into customer behavior, identify trends in user activity, and make informed decisions faster. The need to build a data-driven organization at every level has become one of the most important trends in analytics, driving increased interest in using AI and big data as part of a company’s analytics strategy to achieve these goals.
The best AI analytics solutions, like ThoughtSpot, pair these sophisticated capabilities with an intuitive, recognizable experience like a search bar so all kinds of users, not just technical users, can reap the benefits. By leveraging the insights from AI, companies can improve their products and services, optimize pricing strategies, increase customer loyalty, and develop more effective marketing strategies.
Unlock the potential of generative AI in your data analytics. Discover how it can drive deeper insights and smarter decisions. Learn more in the "Benefits of Generative AI for Data and Analytics" report!
Despite the value traditional analytics brought to businesses in the past, it is increasingly falling short in today’s fast-paced, data-driven environment. Organizations now face several key challenges that limit the effectiveness of legacy analytics solutions:
Siloed data sources: Traditional analytics often rely on data housed in different systems and platforms. This leads to data silos, where teams struggle to access a single source of truth. Without consolidated data, gaining holistic insights becomes difficult, slowing down decision-making processes.
Manual report generation: Business teams typically depend on data analysts to generate reports, which can be time-consuming. These reports often require multiple iterations before delivering the desired results. The reliance on manual processes and human intervention delays the speed at which insights can be acted upon.
Static dashboards: Traditional analytics relies heavily on pre-built dashboards that offer a snapshot of past performance. While useful, these dashboards are limited in scope, requiring constant updates to remain relevant. They also lack the flexibility to explore new data or run real-time analysis, leaving companies reactive rather than proactive.
Technical complexity: For non-technical users, traditional analytics tools often present a steep learning curve. These platforms require significant expertise to build complex queries and visualize data, meaning most business users cannot engage with their data in a meaningful way without relying on technical teams.
Inability to predict future trends: Traditional analytics is largely descriptive, answering “what happened” rather than “what will happen.” Without the ability to leverage predictive analytics, companies miss out on valuable opportunities to forecast trends, mitigate risks, or prepare for changing market conditions.
Limited scalability: As data volumes grow exponentially, traditional systems can struggle to scale. These older platforms were not designed to handle the velocity, variety, and volume of big data today, leading to performance bottlenecks and slower processing times.
AI analytics tools can help all kinds of business people get insights into their data more quickly so they can make better decisions, improve efficiency and productivity, and enhance customer experiences, while freeing the data team from endlessly answering questions from the business to focus on more strategic data initiatives.
Here are some of the key benefits of AI analytics:
Enhanced decision-making
Data exists to help inform nearly every decision we make in our business lives. However, the challenge of finding them means we often go with our gut, making decisions based on instinct. AI analytics rewrites this equation by making finding insights in data as easy as talking with a colleague that not only answers what happened, but why it happened, and what will likely happen next.
By analyzing large amounts of data quickly and accurately, AI analytics can help businesses identify trends, patterns, and outliers that may indicate potential opportunities or risks.
It can also help businesses automate decision-making processes, reducing the time and resources required to make complex decisions. For example, AI-powered fraud detection systems can automatically flag suspicious transactions and alert human analysts for further investigation.
ThoughtSpot's Spotter further simplifies this process. With Spotter, as your AI Analyst, business users can ask data-driven questions in plain English—like “What were our highest revenue-generating products last quarter?”—and gain instant insights without requiring the user to navigate complex dashboards. It also provides predictive insights that help guide future decisions, giving businesses an edge in fast-paced environments.
Improved efficiency and productivity
AI analytics can help businesses optimize their operations for greater efficiency and productivity. By automating repetitive tasks and streamlining processes, AI analytics can help businesses save time and resources, especially for data teams who spend too much time tweaking reports and dashboards. For example, with AI analytics, business users can build their own Liveboard, share that with colleagues, and collaborate directly on an insight without requiring the data team to build something new.
AI analytics can also help businesses identify areas for improvement and optimize their workflows. For example, by analyzing data on employee performance and productivity, businesses can identify areas where training or process improvements may be needed.
ThoughtSpot’s self-service analytics empowers all business users to access insights independently. With Liveboards, users can create and explore data visualizations in real-time, eliminating the need for IT intervention. ThoughtSpot’s intuitive platform allows teams to collaborate seamlessly, freeing up data teams to focus on high-priority projects rather than manual report creation.
Data-driven predictive insights
Predictive insights involve analyzing historical data through advanced statistical algorithms and machine learning techniques to forecast future outcomes, enabling businesses to stay ahead of the competition and make informed strategic decisions.
By collecting and analyzing past behaviors, organizations can build models that identify complex patterns that traditional methods might miss. Predictive insights also enhance risk management by identifying potential threats and opportunities, allowing organizations to implement preventive measures.
With ThoughtSpot’s SpotIQ, users can automate the discovery of insights by analyzing data for hidden patterns and correlations, all without requiring extensive statistical expertise. By leveraging predictive insights, businesses can make informed decisions, optimize resources, enhance customer engagement, and maintain a strategic advantage in the marketplace.
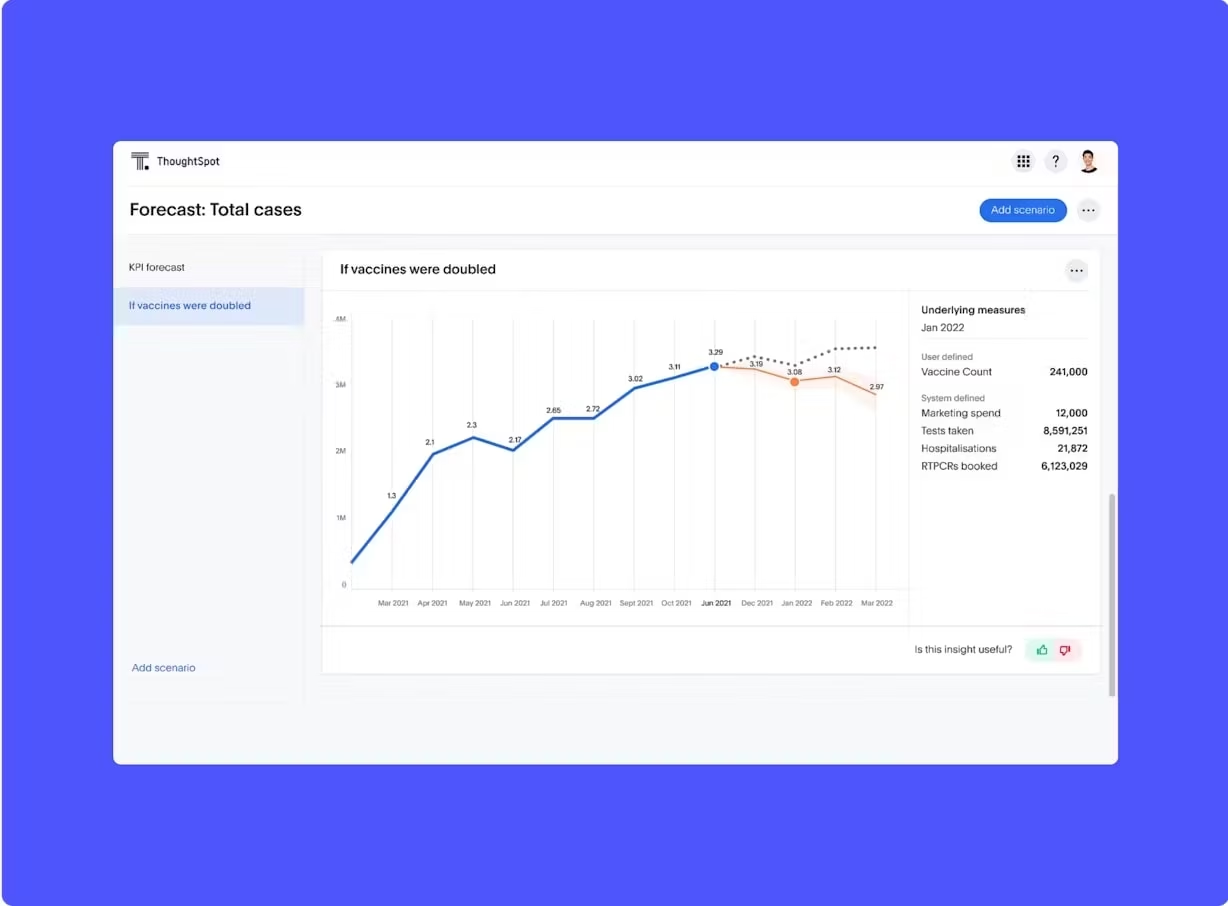
Enhanced customer experience
Delighting customers has never been more critical for brands. AI marketing analytics can help businesses improve customer experiences by providing personalized recommendations and improving customer service that may have otherwise been lost in summarized or aggregated data or buried, unnoticed by the system. By analyzing customer analytics data, businesses can identify customer preferences and behaviors, allowing them to tailor their products and services to meet specific customer needs.
ThoughtSpot’s AI analytics provides deep insights into customer behavior, making it easier to deliver highly personalized experiences. The platform enables businesses to analyze granular customer data and act on real-time insights, enhancing both customer satisfaction and retention efforts.
For instance, Neobank Northmill utilized customer segmentation to personalize banking experiences, resulting in a 30% increase in conversions.
“It is all about being as relevant and personal as possible so users’ personal finance can benefit from the insights.”
Comprehensive real-time updates
Real-time updates are critical for businesses seeking to make timely decisions. AI analytics allows organizations to process and analyze data as it streams in, enabling immediate access to relevant information. This capability empowers businesses to respond swiftly to market changes, customer demands, and emerging trends. For instance, a retail company can adjust inventory levels based on real-time sales data, preventing stockouts or overstock situations.
Features like Change Analysis and AI Highlights harness advanced algorithms to deliver real-time insights. Change Analysis empowers users to pinpoint the factors driving changes and conduct root-cause analysis, while AI Highlights quickly highlights key metric shifts, allowing users to focus on what truly matters.
If you haven’t checked out the Gartner 2024 Magic Quadrant™ for Analytics and BI Platforms, download your copy today.
Every industry is a data industry today, which is AI analytics has a wide range of applications across various industries. Here are some of the key applications of AI analytics:
1. Healthcare
AI in healthcare is helping physicians and healthcare providers to make better decisions, leading to better patient outcomes while controlling costs. Two key use cases of AI analytics in healthcare are predictive analytics for disease detection and personalized treatment plans.
Predictive analytics for disease detection:
AI-powered healthcare analytics is helping healthcare providers to predict and detect diseases in their patients before they become serious. By analyzing large amounts of anonymized patient data, AI algorithms can identify patterns that may identify a disease state more quickly. This early detection allows for earlier interventions and ultimately better patient outcomes.
Personalized treatment plans:
AI analytics is also being used to develop personalized treatment plans for patients. By analyzing data such as patient medical history, genetics, and lifestyle, AI algorithms can develop targeted treatment plans that are tailored to each patient's specific needs. This personalized approach is leading to better treatment adherence, improved patient outcomes, and higher patient satisfaction.
2. Retail
AI in business analytics can help retailors gain valuable insights into their customers, inventory, and sales trends, turning the sea of data from point of sales, marketing, and ecommerce channels into powerful insights that shape strategy and operations. Two key use cases of AI analytics in retail are inventory management and customer service optimization.
Inventory management
AI analytics is helping retailers to optimize their inventory management. By analyzing data such as sales trends, seasonality, and customer behavior, AI can help retailers to determine the optimal amount of inventory to carry. This reduces the risk of stockout costs rising, leading to more efficient operations, and higher profits. Understanding what inventory management is helps retailers appreciate the importance of these optimizations.
See how Canadian Tire is leveraging SKU level insights to drive double digit revenue growth with ThoughtSpot
Customer service optimization
AI-powered retail analytics is also being used to optimize customer service in the retail industry. By analyzing customer data such as purchase history, behavior, and feedback, retailers can use AI analytics to uncover insights into what their customers want and need. This enables retailers to personalize their customer service, scale marketing analytics to increase campaign effectiveness, improve customer satisfaction and sales forecasts.
3. Finance and Banking
AI in finance is helping financial institutions to detect fraud and make more informed investment decisions.
Fraud detection
AI-powered financial analytics help financial institutions detect and prevent fraud. By analyzing large amounts of data, including streaming and real-time data, AI algorithms can identify patterns that may indicate fraudulent activity conducted by people and machines. This early detection allows for earlier interventions and ultimately reduces the risk of financial losses, unhappy customers, and uncertain investors.
4. Manufacturing
AI analytics in manufacturing is helping businesses to improve efficiency and reduce downtime.
Predictive maintenance
AI-powered manufacturing analytics is helping manufacturers to predict when equipment is likely to fail so they can intercede before the failure results in delays or impacts customers. By analyzing manufacturing KPIs such as equipment performance, temperature, and vibration, AI algorithms can detect patterns that may indicate a breakdown is imminent. This enables manufacturers to perform preventative maintenance before a breakdown occurs, reducing downtime, and increasing efficiency.
AI analytics incorporates various elements of analytics and artificial intelligence to deliver a modern data experience. Some of the most commonly used include:
Natural language processing
Natural Language Processing (NLP) is a branch of AI that focuses on enabling machines to understand and interpret human language. NLP techniques are used to analyze text-based data, such as social media posts, customer reviews, and support tickets. NLP can help businesses identify customer sentiment, extract key topics and themes, and classify data based on specific criteria. With the rise of LLMs like GPT 3 and 4, powered in part by massive advancements in transformer architecture, companies are looking for ways to bring the flexibility of natural language to data. Doing so effectively, however, requires pairing natural language processing with a platform that can securely deliver accurate answers your users will actually trust.
We’re seeing a flood of lightweight ChatGPT integrations in the market right now. What stands out about ThoughtSpot’s work is that it is more than just a cool demo. They have carefully thought through the implications of LLMs for their architecture and user experience - and they have even taken consideration of what IT needs to know in order to provision, secure and govern these features. So yes, it’s a cool demo and a feature many will enjoy using. But it’s also the first serious enterprise implementation I have seen of these LLM features in a mainstream analytics platform.
Machine learning
Machine learning involves using algorithms and statistical models to analyze data and make predictions or decisions without explicit instructions. Machine learning algorithms are usually trained on large amounts of data to identify patterns and make predictions. For example, businesses can use machine learning to identify fraud patterns, predict customer churn, or recommend products based on customer preferences. With AI analytics, business users can more easily leverage the hard work data science teams put into building these models.
Neural networks
Neural networks are a type of machine learning algorithm that is inspired by the structure and function of the human brain. Neural networks are composed of layers of interconnected nodes that are designed to process and analyze data. Neural networks can be used for a variety of applications, such as image recognition, speech recognition, and natural language processing.
Deep learning
Deep learning is a subset of neural networks that involves training models on large amounts of data to make accurate predictions or decisions. Deep learning algorithms are capable of processing and analyzing large and complex datasets, making them ideal for applications such as image and speech recognition. Deep learning is a key technology behind many AI applications, including self-driving cars and voice assistants, and relevant for AI analytics, particularly around unstructured data.
AI analytics is a powerful way to extract key insights from large datasets, charting a new course for data teams and businesses at large as they look to capitalize on the once-in-a-generation opportunity. AI analytics closes the chasm between humans and machines like never before, bringing insights to users before they even know to ask, while letting them endlessly explore until their curiosity is satisfied.
By deeply understanding the various functions of AI analytics, companies can use its benefits to gain a competitive edge in their respective industries. Whether you are looking for fraud detection or analytical insights on consumer behavior, AI analytics has something to offer everyone. Take advantage of all this technology's great features and schedule a free demo today.
1. What is the difference between AI analytics and traditional analytics?
Traditional analytics primarily focuses on descriptive insights, which help businesses understand what happened in the past. AI analytics, on the other hand, leverages artificial intelligence and machine learning to go beyond historical data. It provides predictive and prescriptive insights, helping users understand not only what happened, but also why it happened, what is likely to happen next, and what actions they should take.
2. How do AI-driven analytics tools differ from AI data analytics?
Both terms refer to analytics powered by AI technologies, but there is a subtle distinction. AI-driven analytics typically refers to AI technologies actively guiding or recommending decisions based on insights, whereas AI data analytics focuses on the AI's role in processing and analyzing large datasets to surface patterns and trends. In short, AI-driven analytics is more action-oriented, while AI data analytics is centered on data discovery.
3. How is AI analytics different from business intelligence (BI)?
While both AI analytics and BI help organizations leverage data for insights, AI analytics takes it a step further by automating complex tasks, offering predictive and prescriptive insights, and using machine learning to improve accuracy over time. Traditional BI typically focuses on reporting and visualizing historical data, whereas AI analytics helps predict future outcomes and suggests actionable steps.
4. Can AI analytics integrate with existing data tools and systems?
Yes, most AI analytics platforms, including ThoughtSpot, are designed to integrate with a wide range of data sources and tools such as CRMs, ERPs, data lakes, and data warehouses. This ensures that businesses can continue using their existing systems while layering AI analytics on top to enhance data analysis capabilities.