As a banking professional, you're no stranger to the complexities of financial operations and customer dynamics. But in a landscape where customer expectations are as diverse as the services you offer, how do you ensure your bank remains the preferred choice?
According to J.D. Power’s U.S. Retail Banking Satisfaction Study, 78% of banking consumers expect personalized support from their bank. This is where banking analytics emerges as your trusted ally. By tapping into the power of data, you're not just crunching numbers; you're shaping experiences, anticipating needs, and boosting your bank's reputation.
In this article, we’ll explore how banking analytics can revolutionize financial institutions’ operations in today's dynamic market.
Table of contents:
Banking analytics is the process of collecting, analyzing, and interpreting data within the banking industry. By extracting valuable information from various sources, such as customer transactions, market trends, risk assessments, and operational performance, financial institutions can gain valuable insights and make informed decisions. Banking analytics requires the use of various analytical techniques and technologies including data visualization, business intelligence, AI-assisted data modeling, data mining, real-time KPI monitoring, and big data platforms.
1. Enhanced customer insights
With banking analytics, and more specifically customer analytics, you gain comprehensive insights into customer behavior, preferences, and needs, enabling you to tailor products and services accordingly. This deep understanding empowers you to anticipate customer demands, identify emerging trends, and foster stronger relationships with your clientele, ultimately leading to increased customer loyalty and satisfaction.
Let’s take a look at Rakuten, for instance. In an episode of The Data Chief, Rakuten's former Managing Executive Officer & CDO, Takuya Kitagawa, shares how the company's integrated data approach has revolutionized customer experiences across diverse industries. By seamlessly connecting data across various lines of business and unifying login credentials, Rakuten ensures a seamless journey for users, fostering enhanced insights and unwavering loyalty.
2. Improved risk management
By analyzing transaction data and market trends, banking analytics facilitates proactive risk identification and assessment, reducing financial losses and enhancing security measures. You can detect potential fraud, assess credit risks with increased accuracy, and comply with regulatory requirements. This helps in safeguarding both your assets and reputation in the market.
3. Optimized operations
With banking analytics, you can identify and mitigate inefficiencies, leading to streamlined processes, heightened efficiency, and significant cost savings. By analyzing vast amounts of data, you can uncover inefficiencies in everything from transaction processing to customer service. This heightened efficiency not only improves customer satisfaction but also translates into significant cost savings as resources are utilized more effectively.
4. Data-driven decision making
Banking analytics equips you with the tools and insights necessary for making well-informed decisions about product development, marketing strategies, risk management, and overall business performance. This is achieved through rigorous historical data analysis and predictive analytics. By utilizing the power of data-driven insights, you can confidently navigate market uncertainties, and capitalize on emerging opportunities.
5. Competitive advantage
Banks that leverage analytics gain a formidable competitive edge. You drive innovation, enhance customer experiences, and effectively mitigate risks. This enables you to attract and retain customers while outpacing competitors. Such strategic advantage allows you to differentiate yourself in the market, seize opportunities, and maintain a strong foothold in an increasingly competitive industry landscape.
There are several types of banking data analytics, each serving different purposes and providing valuable insights for your financial institutions. Some of the key types include:
Descriptive analytics: Descriptive analytics focuses on summarizing historical data to help you understand what has happened in the past. It involves basic statistical analysis, data aggregation, and visualization techniques to provide insights into trends, patterns, and relationships within your data.
Diagnostic analytics: Diagnostic analytics goes deeper into understanding why certain events occurred by identifying the root causes of past outcomes. It involves more advanced data exploration techniques, such as drill-down analysis and correlation analysis, to uncover the underlying factors contributing to specific trends or anomalies.
Predictive analytics: Predictive analytics uses historical data to forecast future outcomes and trends. It employs statistical modeling, machine learning algorithms, and data mining techniques to identify patterns and relationships that can be used to make predictions about future events, such as customer behavior, market trends, and risk levels.
Prescriptive analytics: Prescriptive analytics helps you go beyond predicting future outcomes to recommend actions that should be taken to achieve desired objectives. You can combine predictive models with optimization algorithms to simulate different scenarios and determine the best course of action based on your predefined business goals and constraints.
Real-time analytics: Real-time analytics involves analyzing data as it is generated in real-time to provide immediate insights and support decision-making processes. It is often used for monitoring transactions, detecting fraud, and responding to dynamic market conditions quickly.
Behavioral analytics: Behavioral analytics focuses on analyzing your customer's behavior and interactions to understand their preferences, needs, and patterns. It helps you personalize your services, improve customer experiences, and optimize marketing strategies based on individual customer insights.
Risk analytics: Risk analytics involves assessing and managing various types of risks, including credit risk, market risk, operational risk, and regulatory compliance risk. It uses quantitative models, stress testing techniques, and scenario analysis to measure and mitigate risks effectively.
Customer segmentation
With banking analytics, you can segment your customers based on demographics, behavior, and transactional patterns. By understanding the distinct needs and preferences of different customer segments, you can tailor your products and services to target specific groups more effectively.
For instance, Neobank Northmill was experiencing technical bottlenecks with its legacy BI tool. This limited its ability to leverage valuable new customers and effectively boost customer conversion rates. By implementing ThoughtSpotr self-service analytics, they were able to analyze user data and identify areas for improvement in the onboarding process. In turn, Northmill boosted its customer conversion rates by 30%.
“It is all about being as relevant and personal as possible so users’ personal finance can benefit from the insights. It can be getting a notification of a discount deal at your favorite cafe or a tailored offer to lower the cost of your insurance.”
Credit risk assessment
Banking analytics plays a crucial role in assessing credit risk by analyzing factors such as credit scores, income levels, debt-to-income ratios, and repayment histories. Predictive models and machine learning algorithms help you predict the likelihood of default and determine the creditworthiness of borrowers. For example, you can use analytics to approve or deny loan applications based on the risk profile of the applicant.
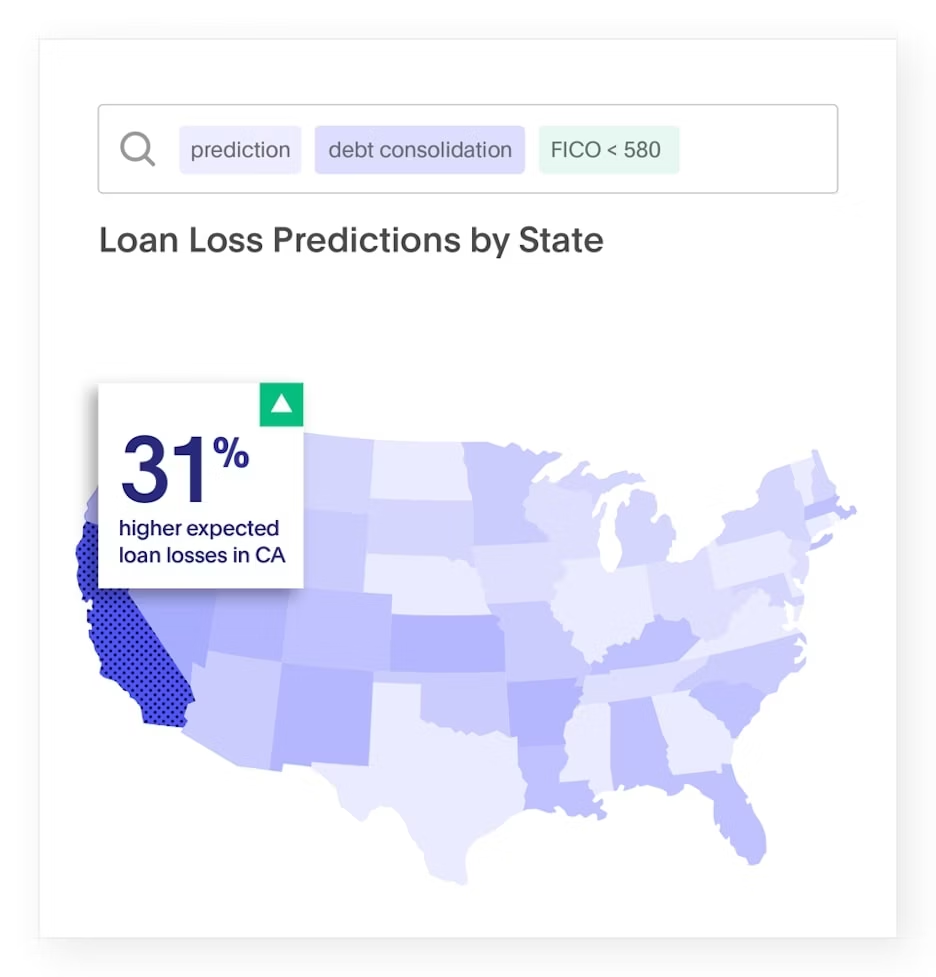
Fraud detection
With analytics, you can also detect and prevent fraudulent activities, such as unauthorized transactions, identity theft, and account takeover scams. Machine learning algorithms analyze transactional data in real time to identify suspicious patterns and flag potentially fraudulent activities for further investigation. You can also use analytics to detect unusual spending patterns or multiple failed login attempts, indicating potential fraud.
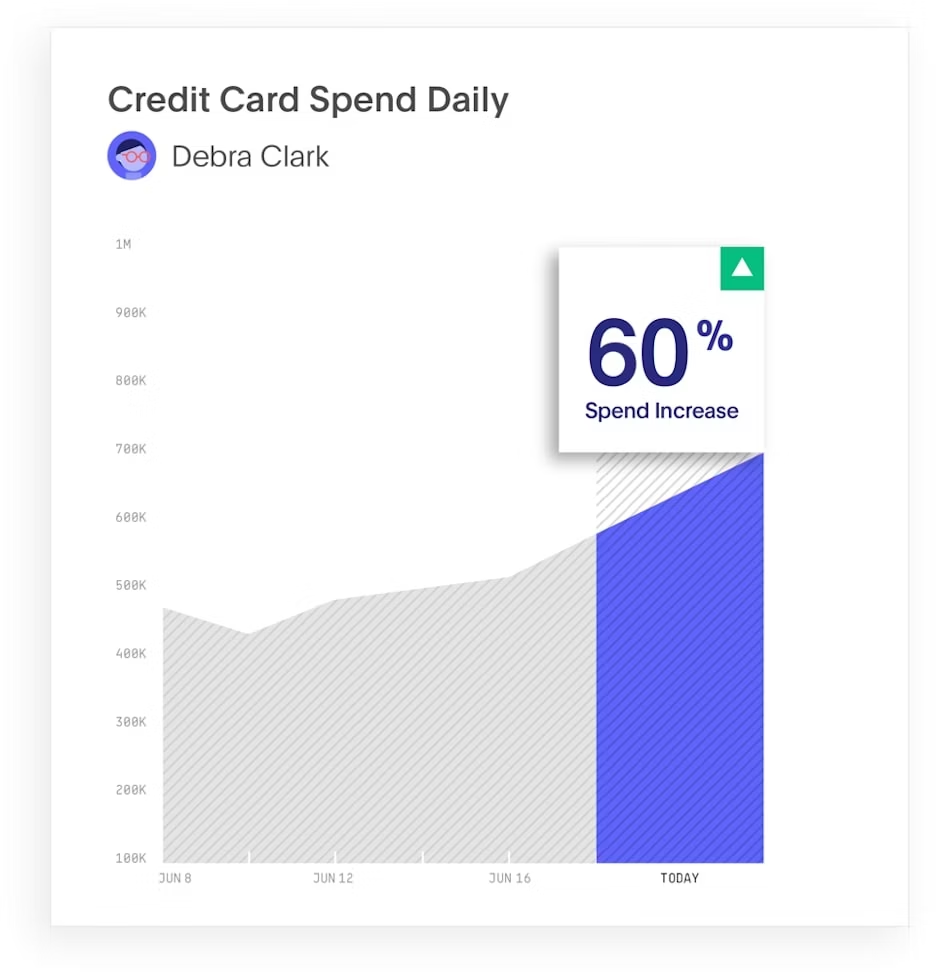
Cross-selling and upselling
Your go-to-market team might leverage analytics to identify opportunities to cross-and up-sell additional products and services to your existing customers. By analyzing customer data and transaction histories, you can identify complementary products that are likely to appeal to specific customer segments. For example, you can use analytics to recommend mortgage refinancing options to customers with existing home loans.
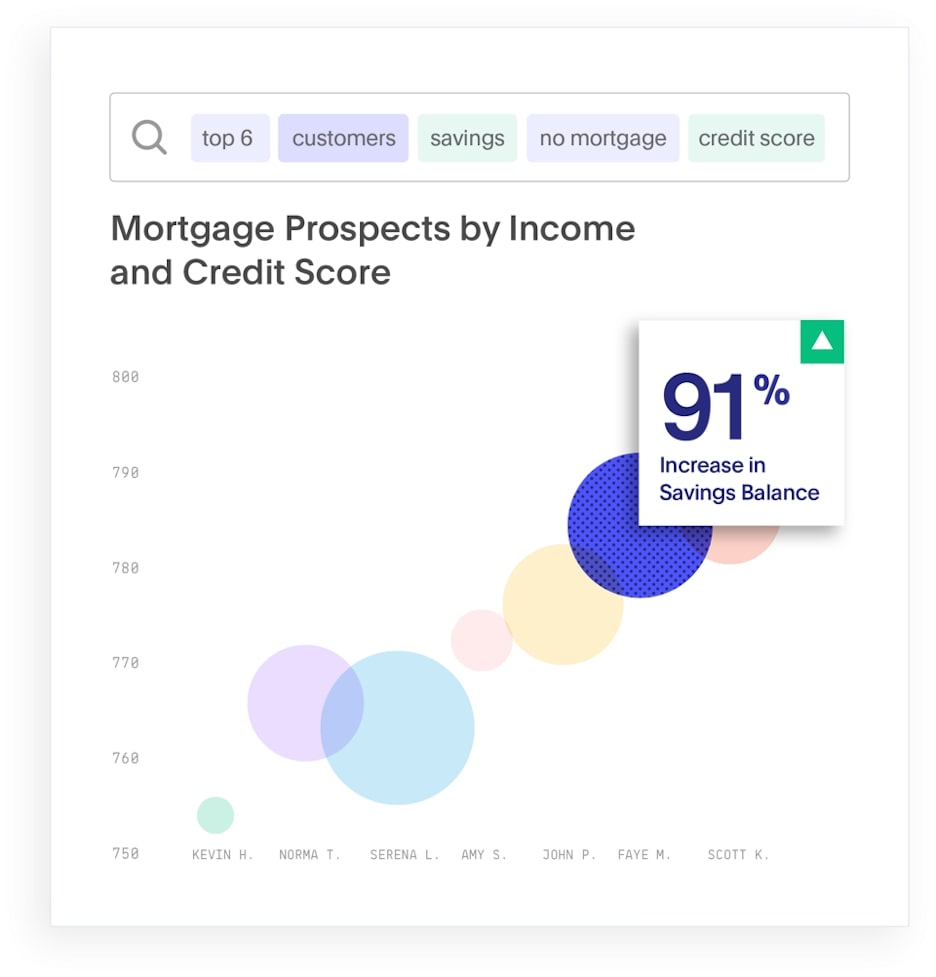
Marketing campaign optimization
Marketing campaign optimization goes beyond customer segmentation. By analyzing specific campaigns, strategies, and tactics used to target and engage different customer segments, you can understand which sources and messaging have the most impact.. This targeted approach leads to higher conversion rates, increased customer engagement, and improved return on investment for your marketing efforts.
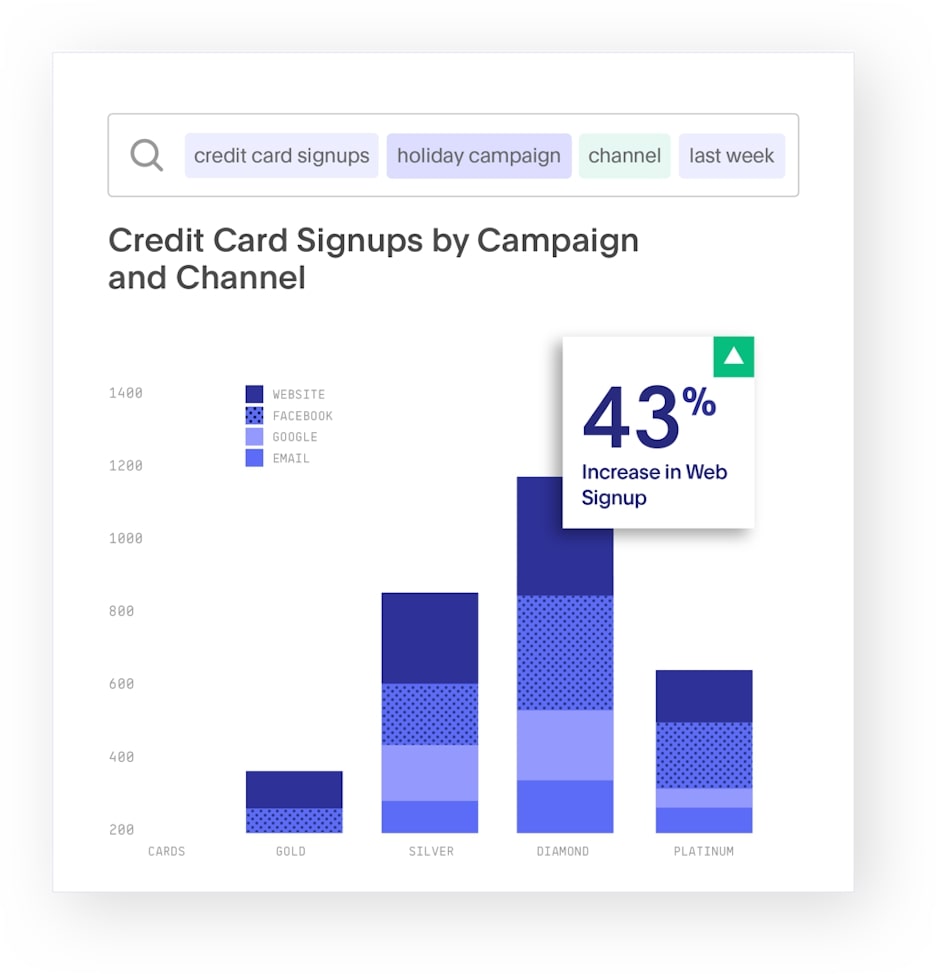
Consider Austin Capital Bank. Their complex and disconnected data environment delayed marketing optimization efforts. With ThoughtSpot AI-Powered Analytics, Austin Capital Bank empowered users to effortlessly generate insights and drill down into their data. With these new self-service capabilities, their marketing team achieved a 50% reduction in paid search spend, creating a 30% increase in revenue margin.
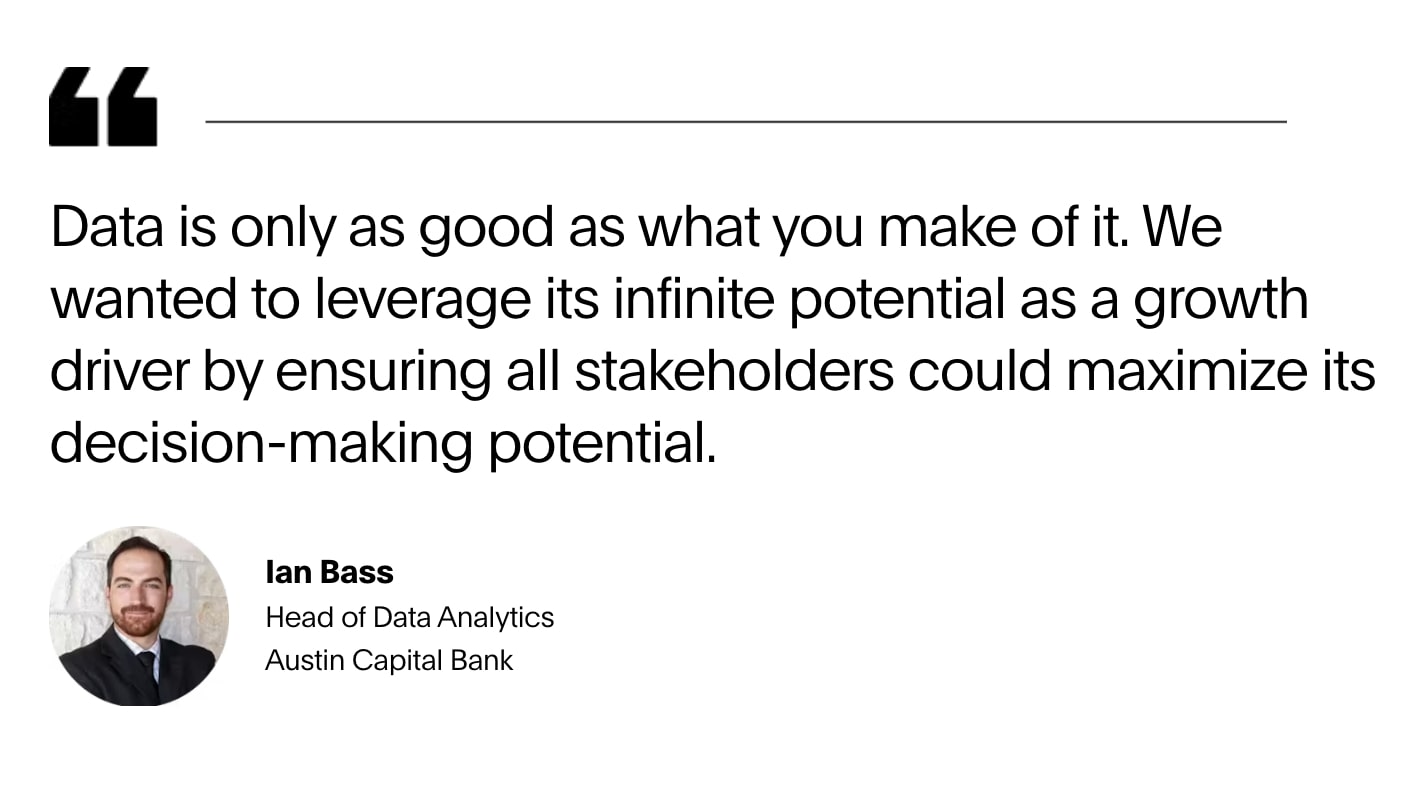
The right analytics platform can positively transform your performance in the finance sector. ThoughtSpot AI-Powered Analytics is tailored for banking. With Spotter, as your AI Analyst, ThoughtSpot empowers any member of your team to discover actionable insights—regardless of their technical data experience.
Ready to unlock the full potential of banking analytics? Join a live ThoughtSpot demo today!