Imagine a world where in year from now, Google (Waymo really) or Apple comes out with self-driving cars that actually work. You simply speak your destination, lean back, close your eyes and in 30 minutes, you wake up and you’re at work.
Also imagine that most other players have not advanced their tech. For example, Tesla still claims to be self driving but really most people try it once or twice and then don’t turn it on. A number of car companies are in a rush to come to this market so they have gone and quickly added radar and few cameras to their cars, which means if you're willing to control the steering, they could maintain distance from the next car. Having experienced suboptimal alternatives, many people may have a hard time believing that it actually works. Others will ask, how is this offering different from all the others that also have radars and cameras. But once you experience a real self driving vehicle, you will know that this is going to change the way we move around.
Finding the Holy Grail of Analytics
That’s exactly how we want our customers to feel the first time they try ThoughtSpot. It has always been a Holy Grail for the Industry to be able to simply allow business users to ask a question and get an answer. Anyone who has experienced how painful it is to get the data questions you need answered in most enterprises will tell you that no one has found that Holy Grail.
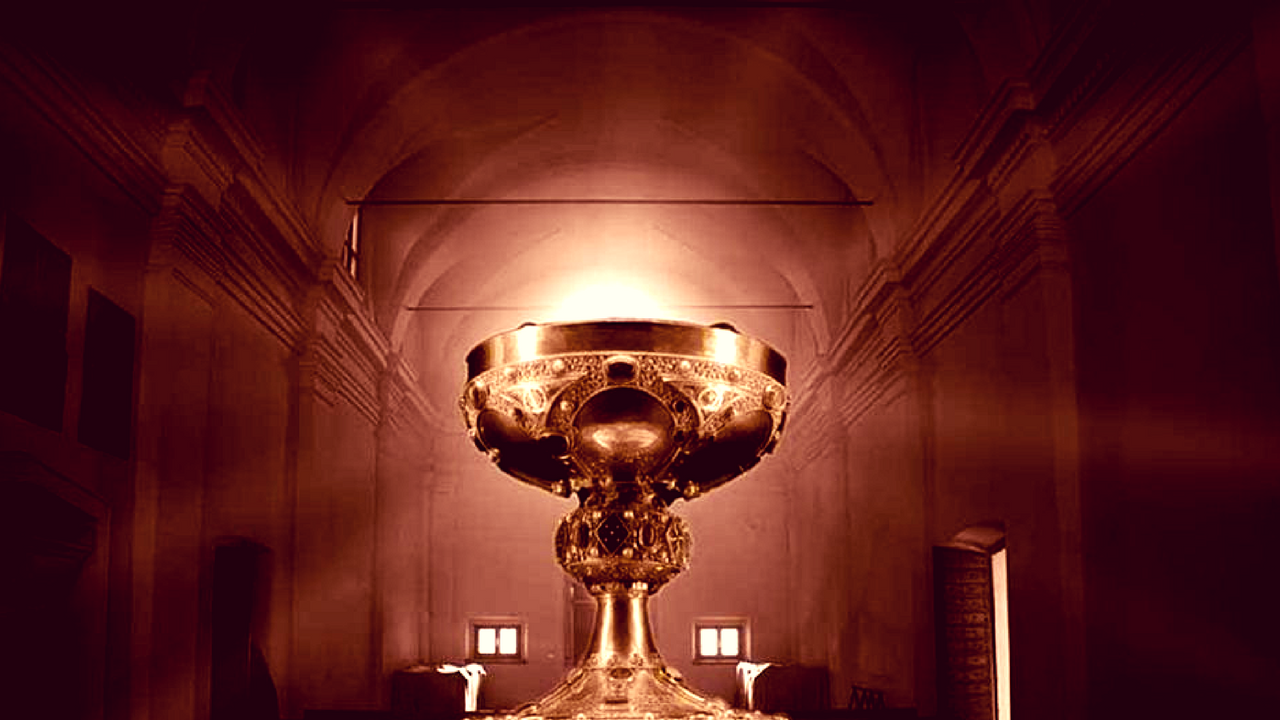
In the last 30 years, many have tried to build such products, but failed. Others have promised such products to the market, only to disappoint. When we started building our product in 2012, very few people thought we could succeed at this. We realized that to have any shot of solving this problem, we needed the holy trinity of UX Design, AI, and Distributed Systems. When we came out with our initial product in late 2013, the most common first response was a mixture of amazement and disbelief. And with all of our new capabilities, that continues to be true to this day.
While we can’t claim to have found the holy grail yet, our customers think we have come closer than anyone else. That’s why one of the industry leaders in every major vertical, be it retail, tech, auto, medicine, or finance, has invested millions of dollars in this technology. Many of these companies have thousands of employees using ThoughtSpot every day to ask questions, find insights, and make decisions.
Why have enterprises in every major industry made ThoughtSpot the leader in search and AI? It’s true others are trying to add search to their BI platforms as a feature or through acquisitions, but when it comes to analytics, search is not a side hustle. It is the same analogy. Just like it’s easy to add a radar and camera in any old car, but very hard to make it truly self-driving, it is easy to add a search box on your UI and show demos, but very hard to make it work in real life.
So what makes ThoughtSpot work at enterprise scale?
1. A Powerful Scale-out System as a Data Platform: We realized early on, that you cannot call something Google-like Search if it takes minutes to answer a question. Also, it would have to be able to handle the billions of rows of data that modern enterprises have, which surpasses the limit of most single node solutions in the industry. A lot of our customers are running ThoughtSpot with upwards of 10B rows of data in tens or hundreds of nodes, and still get interactive responses to their questions at that scale. Their closest alternative requires them to go get a coffee before the answer loads.
2. Marriage of UX Design, Machine Learning, and Indexing System: Search-driven analytics is a really hard problem, and in many ways an “AI complete problem”. So while machine learning is the core of the solution, you need to consider UX design and large scale compute systems that work along with it. UX, because you need to design the system in a way that people can fill the gap when algorithms fail and the system has to get smarter everytime that happens. Large scale systems, because systems only get smart by knowing a lot about the world, and being able to quickly access that knowledge. Large scale systems are the only means of providing that access. We have built an indexing system that can index billions of facts and entities, which can be retrieved through search in the order of relevance within 5-10 millisecond. We have not seen any other product in the market make this kind of investment.
3. Large Scale Natural Language Processing: With the tremendous advances in NLP technology and abundance of open source libraries, these days it is relatively easy for anyone to add a dependency parser, or a named entity extractor to get going on building a demo-worthy natural language processing engine. The challenge comes from the fact that language and the entities change significantly from enterprise to enterprise. A drug company may have thousands of drugs and diseases in the language they express to ask questions while a retail company may have millions of products and product categories. Making the same NLP libraries work well in these diverse situations becomes a very interesting problem that we have been chipping away at for the last 4-5 years - including with the launch of SearchIQ.
4. Enterprise Class Security with Search: An enterprise analytics product that gives unprecedented access to data is not going to go far without properly securing the data. If you allow row-level security, where different users have access to different subsets of rows (for example, the person in charge of Germany can only see data around transactions in Germany), then you also have to secure the search suggestions. For example, if someone types “Dai” and one of the suggestions is “Daimler” this may expose the fact that “Daimler” exists as a prospect or customer, which may not be okay. Turns out it is not easy to solve it at scale. We had to do some fundamental computer science innovation to make it possible. And we’re not aware of any other product doing it.
5. A System that Truly Understands Relational Algebra and Complex Schemas: Most of the business intelligence products have mostly simplified their own lives by assuming that the users are supposed to provide a single table extract or a set of tables that form a star/snowflake schema. We have found that most of the value can be unlocked by bringing disparate sources of data. For example, bringing together sales and inventory with ad impressions and purchases uncovers insights you’d never find looking at those datasets in isolation. ThoughtSpot has invented new algorithms, which inspect the structure of relationships between tables and automatically generate the right sequence of aggregations needed to get accurate results.
6. Six years of iterative improvements: In the year 2000, Google was by far the best internet search engine in the world, but there is no comparison between how good the Google of today is with the Google of 2000. We have been going through a similar journey of our own. We have been working with some of world's largest enterprises over the last 6 years, and using feedback from their users to improve our self-service BI platform. Every year we have been adding more and more smarts to it, creating even more value for our users.
We believe the world is going to be a better place when all analytics tools have a search functionality. We are glad that the entire industry is coming to our way of thinking and wish them all the best with their search endeavors. By no means have we solved the problem completely and there is a ton of room for someone else to come along and do this better than us, but they will have to work hard for it, and innovate at a rapid pace.
We are certainly not stopping our innovation train anytime soon, and are eagerly looking forward to some fierce competition and better products in the market.