Picture it: You are the owner of a growing digital purveyor of premium baked goods. You see that your chocolate chip, macadamia nut cookies with a hint of coconut are going gangbusters whereas sales for peanut butter cookies are declining. This is bad for margins because the peanut butter cookies cost less to produce than the chocolate chip, macadamia nut, coconut cookies. You decide to offer a special coupon for your loyalty customers to grow the peanut butter cookie sales. Your analytics platform sends you a KPI alert two weeks later. Boom! Peanut butter cookie sales have increased by double digits. Your coupon paid off and your decision was sound, at least for now. You need to see if this promotion has staying power so you keep an eye on your Liveboard by customer segment, region, and week with the promotion date clearly flagged on the trend line.
This is decision intelligence and the ideal process of leveraging data from insight to action to assessing the impact of that action.
Decision intelligence vs Business Intelligence
Decision intelligence is the process of capturing decision points, applying data and human judgment to these decision points, and monitoring the impact of these decisions.
Gartner defines decision intelligence as: “a practical discipline used to improve decision making by explicitly understanding and engineering how decisions are made and how outcomes are evaluated, managed and improved via feedback.”
Back in the 1980s, mainframe-based dashboards were called decision support systems or DSS. With the rise of relational databases and client server technologies in the 1990s, the category was renamed business intelligence (BI), conveying both a shift in the technology but also the degree to which decision makers could interact with a report or perform their own queries.
Throughout the industry’s waves of innovation, the concept of tracking which analytical assets supported specific decisions has largely been a manual process. For example, a marketer may have a Liveboard called “campaign effectiveness,” but decisions related to specific campaigns might appear only in note tiles at best. The degree that decisions were tied to a specific business outcome with full automation and auditability is largely absent in the BI and business analytics world.
Meanwhile, with machine learning and operationalization of machine learning (ML) models, these decisions are beautifully documented with a clear impact.
Approve a loan if the credit score is above 700, decline it if the score is below 700.
Interview the candidate if they majored in business analytics and had a certain GPA, reject if there is a career gap.
Display a coupon on the website at check out time if the shopping cart is greater than $100 and the customer is in the loyalty program.
Different types of decisions
The type of decision being made and by whom is also correlated with the degree that a decision can be automated via an ML model or exploratory analytics. In Smart Enough Systems, authors Neil Raden and James Taylor provide a useful model in thinking about the types of decisions in the context of value and volume.
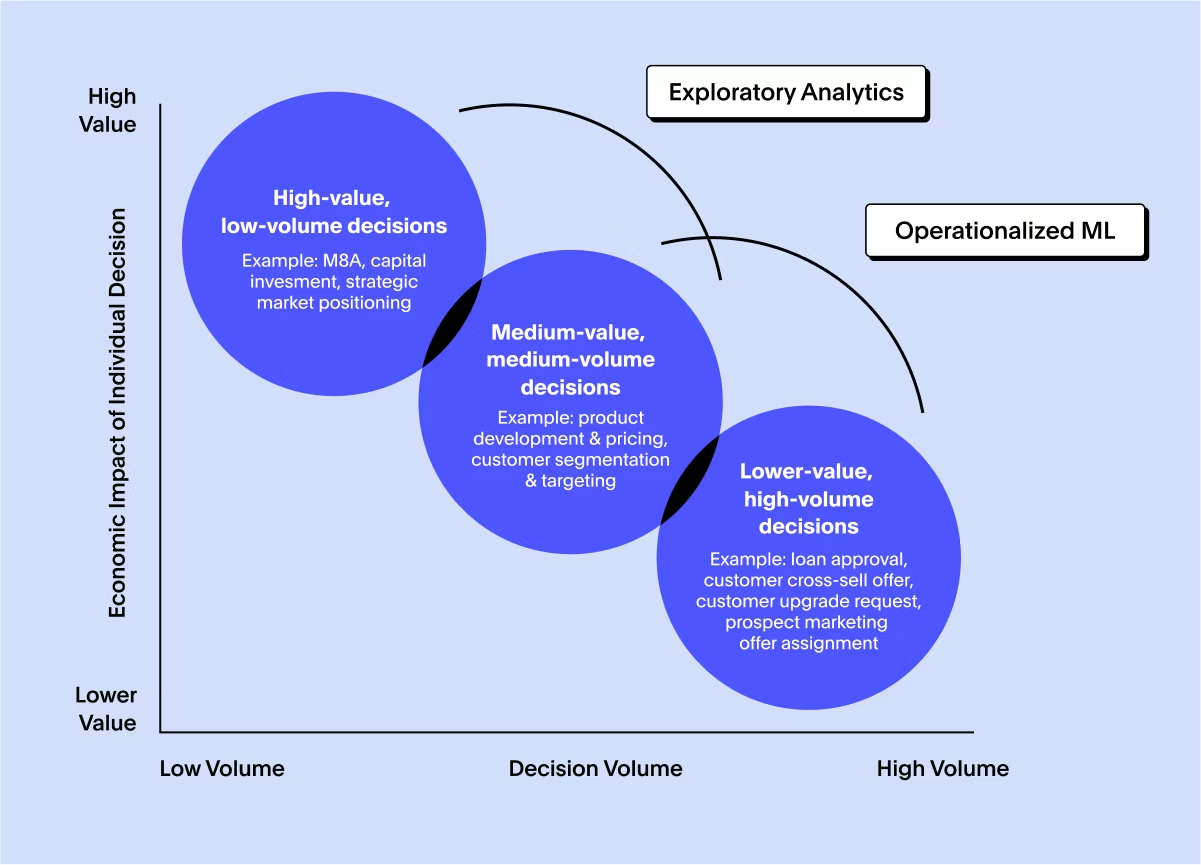
Micro decisions vs. one-time decisions
Micro decisions are the lower-value but high-volume decisions that front-line workers typically make. Offering a coupon or recommending a move can be automated. At the other end of the spectrum, the decision to enter a new market, or perhaps to expand the online bakery to physical locations is a one-time decision in which the questions and answers cannot be automated. It requires much more iteration and human interactions: which locations? Stand-alone store versus distribution through convenience stores?
Using decision intelligence to empower frontline employees
In our fictional bakery store example, the decision-maker is the owner and therefore is clearly empowered to make the decision on offering a coupon and to whom.
But what about if it is a customer service representative who is dealing with an angry customer? Does this representative have visibility into the data to see what went wrong with the order or how often has this customer ordered in the past? All of these questions may start with a Liveboard that then requires additional exploration. “Oh, I see there was a crazy weather pattern in your town, so the order was late and for a special occasion. I see you are a loyal customer so let me refund your order or offer you a discount on a future order. Which would you prefer?”
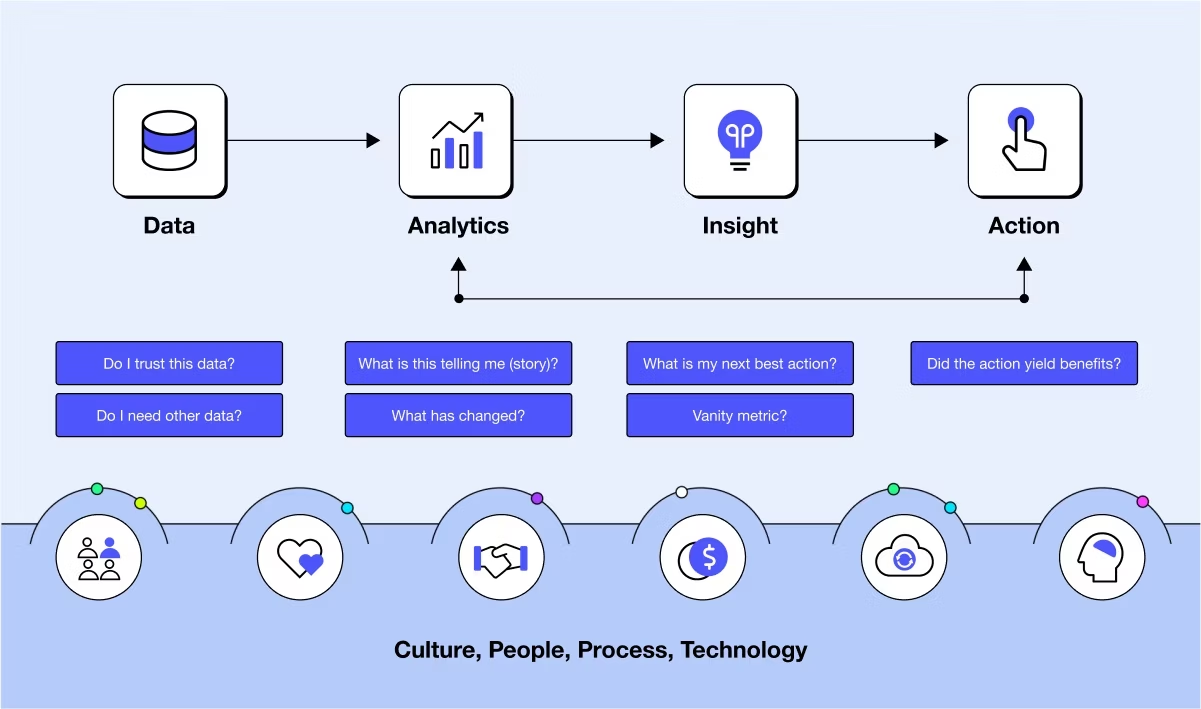
In this example, the rep is empowered to make amends for the order that went wrong, even though the bakery is clearly not responsible for weather delays. There is a culture of delighting the customer, no matter what caused the order delay. For the rep to investigate the error and understand this specific customer, they also need access to granular data—and to trust that the data is complete. What if loyalty data is incomplete? What if the unhappy customer appears in the system to be a one-time customer but is actually a top-10 customer? Lack of trust in data is yet another reason that customer-facing and other frontline decision makers will default to stricter, rules-based decisions.
A genuine decision intelligence system would track the impact of these micro decisions to see if the customer does indeed remain loyal. Note that we are also talking about very detailed analysis at the customer level, not just at a customer segment level. In the past, on-premises data platforms had limitations in terms of scalability and granularity of insights, but flexible cloud data platforms have opened the doors to deeper analysis.
Is decision intelligence technology ready for the enterprise?
The idea of decision intelligence has existed for decades, but as a technology, there have been historical barriers to anything beyond rule-based or operationalized ML. Fast forward to 2023, and decision intelligence is more than demo ware. However, since Gartner identified decision intelligence as a top trend in 2022, some vendors have rebranded their platforms to ride this hype cycle without actually changing the underlying capabilities. Very few are capturing decision flows.
Emerging players such as Cloverpop and Diwo.ai allow organizations to model decision flows and operationalize AI. Diwo.ai includes recommended actions based on AI-generated insights. Others such as Aera focus on specific process flows such as supply chain optimization.
Sisu, which started as an ML powered automated analytics platform has repositioned itself as decision intelligence, focusing on KPI optimization. Traditional BI vendor Pyramid Analytics has rebranded as decision intelligence, but the capabilities remain in the category of exploratory analytics. ThoughtSpot has continued to position itself as an AI-Powered Analytics platform, but also includes a number of insight-to-action capabilities as well as integration with ML models.
In the insight-to-action example below, our SVP of Product Marketing shows how you can use ThoughtSpot to perform complex segmentations. He also demonstrates how ThoughtSpot connects with other CRMs, like HubSpot, allowing you to orchestrate downstream marketing activities, like setting up a segmented email list, using custom actions.
Try it out for yourself with our 14-day free trial.
With more data and modern cloud ecosystems, decision intelligence can now be smarter, fueled by the best combination of human insight and technical advances to support both strategic and operational decisions.
The following table shows how the technology has evolved.
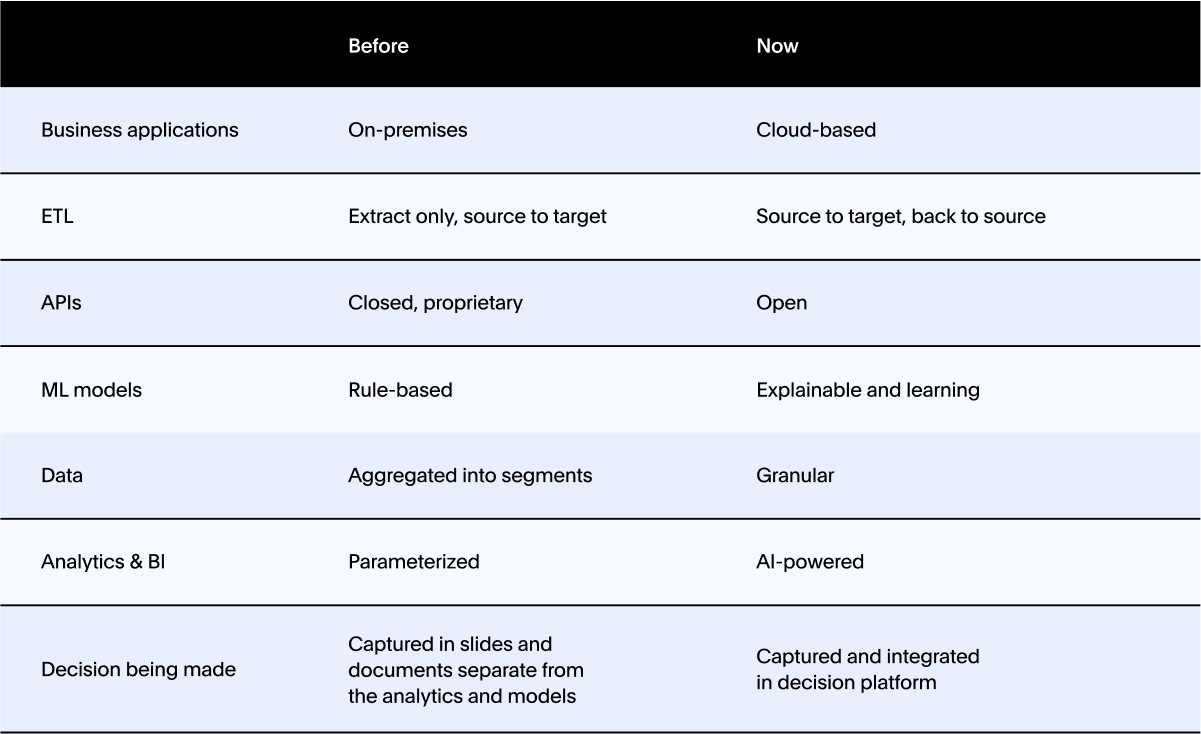
What do look for when evaluating a decision intelligence platform
Now that we have addressed the differences between business intelligence and decision intelligence, as you evaluate alternatives consider:
The degree that the solution is providing analytics as opposed to capturing decision points and rationale for those decisions. Be wary of marketing hype.
The degree that an analytics platform allows a decision maker to execute an action from within the platform.
How much the platform is modernized as described in the table.
The types of decisions being made, whether high value/low volume, aka strategic or lower value/high volume aka operational and micro decisions.
Best practices for decision intelligence
Back to our bakery example, the owner was so thrilled with the outcome of his peanut butter cookie campaign and improved margins, that they are exploring broadening the distribution for this cookie via high end coffee shops in select markets. They will bring data to bear in this decision as analytics leaders do. The owner may or may not capture the dates of these decision points in a slide, a strategy document, or a decision platform. Some may remain purely part of the tribal knowledge of an organization.
As you look to bring decision intelligence into your organization, consider the following best practices:
Embrace data informed decisions to improve business outcomes.
Recognize the differences in volume, value, and people making decisions and align the technology capabilities according to these differences.
Take a buyer-beware approach and understand if a technology provider is providing analytics to support decision making or a true platform to operationalize decision making.