Since the introduction of computing systems, there has always been a need for data storage. Today, there are many cloud data warehouse solutions on the market—fully integrated shops like Amazon, Google, and Microsoft and standalone data platforms like Databricks and Snowflake. In this article, we’ll provide a practical overview comparing the top two standalone data platforms, Databricks vs Snowflake, so you can select the data solution that’s best for your business’s analytics.
Databricks vs Snowflake comparison in terms of:
Databricks’ cloud data platform offers a unified analytics workspace for big data processing, machine learning, and AI applications. It’s built on top of Apache Spark, an open-source, Resilient Distributed Datasets (RDDs) framework designed for processing and analyzing large volumes of data. This enables Databricks users to simplify their data processing and analysis tasks at scale.
Snowflake also is a single platform, fully managed cloud solution that mainly focuses on data storage, management, and analytics. It’s designed to support massive parallel processing (MPP), enabling fast data querying and analysis. Snowflake caters to various data applications, including AI/ML, data warehousing, data lakes, unistore, data engineering, data science, and data application development. Additionally, Snowflake offers secure data sharing and consumption in real-time or shared environments.
Takeaway: To pick the best architecture for your business, focus on the use cases you're trying to solve, and then make sure the architecture can support those efficiently and at scale.
Data structures refer to the way data is organized and stored within the warehouse to optimize query performance, facilitate analytics, and support efficient data processing. When and how you restructure your company data may play an important role in selecting the best solution for your business.
Databricks allows users to store and access all data types in their original format (e.g. unstructured, semi-structured, structured). Snowflake also supports all data types, so long as it’s transformed into Snowflake native format. This is an area both companies are continuously innovating and evolving based on market and customer feedback. Here’s why:
Structured vs unstructured data
Structured data refers to data organized in a predefined manner. Some benefits include data integrity, query performance, readiness for analytics, and data governance.
On this other hand, unstructured data lacks a predefined structure or schema. This is often used for data assets like text, images, audio, video, or social media content. While unstructured data does provide flexibility, it can be difficult to manage—creating complex queries and poor query performance during analysis.
Most businesses use a hybrid approach to data storage. And depending on what kind of data you collect, you may find benefit to storing unstructured data. But you should be wary of query cost and performance when you bring analytics into the unstructured data mix.
Takeaway: It’s important to ensure your cloud data provider and its allowed data structures are aligned with your business data and your goals.
When discussing cloud data warehouses and platforms, performance refers to the speed and efficiency that the cloud database processes and delivers query results. Good performance directly impacts the ability of organizations to derive insights, make informed decisions, and maintain a productive data processing environment. It also impacts your costs, so it’s important to keep a steady pulse on the performance factor.
As mentioned above, Databricks is designed to leverage the Spark framework—making fast work out of processing large volumes of data. To reduce the amount of data processed, it uses data pruning on partitions and Parquet file metadata. By selectively excluding unnecessary data and metadata, Databricks’s processing style can help reduce your storage footprint, improve query performance, and assist with data migration.
Using its micro partition storage approach, where data is organized into finely grained portions, Snowflake is able to scan less data than its larger petition counterparts—saving you time and money. Additionally, Snowflake’s ability to isolate workloads over its decoupled storage and processing system allows you to scale each component separately without competing for resources.
Takeaway: By understanding how each solution is built, you can gain invaluable insights into its performance. You'll want to ensure the cloud data platform is performant in the areas most valueable for your business.
It’s no secret that data security is a top concern for nearly every business today. This factor has even hindered some industries, like healthcare and finance, from moving into cloud data environments. But today’s cloud data warehouses and platforms are meeting this challenge head on. Here’s how Databricks and Snowflake stack up.
Databricks’ security measures include rest encryption, network isolation, and user-and role-based access control. It’s also designed to allow more control over data governance and resources access through its built-in integrations with Identity and Access Management (IAM) systems.
Of course, Snowflake's security architecture is also crafted to ensure the utmost protection of customer data. This is achieved through various measures, such as facilitating encryption for data at rest and in-transit, implementing network isolation, and enforcing access control based on users and roles. In addition, Snowflake offers features like data masking and secure views, which play a pivotal role in safeguarding sensitive data.
Takeaway: Data security is top-of mind for data teams, cloud data providers, and BI solutions alike. Together, we have the tools and policies in place to keep consumer data safe and governed, but it’s always smart to learn more about a company’s security before including them in your modern data stack.
As your business and data volumes grow, it’s becoming increasingly important that your data cloud is built to scale with you. Of course, it’s not just about having the ability to expand data capacity. Scalability in cloud data warehouses also determines how well the system can handle increasing user load and processing demands.
While data clouds are generally designed to accommodate growth at scale more efficiently than an on-prem data warehouse, let’s look at a few distinctions between Databricks and Snowflake.
Both Databricks and Snowflake exhibit excellent scalability. Databricks allows autoscaling of the clusters based on the workloads. It also allows flexibility in selecting nodes and the number of scale-out nodes. Snowflake also supports auto-scaling horizontally for higher query concurrency during peak hours. However, Snowflake’s main scalability factor lies in its decoupled storage/compute architecture. This design supports the ability to resize clusters without downtime.
Takeaway: When selecting which cloud option is best for your data storage and scalability, consider your own architecture—how you store data and where you currently see and anticipate the largest growth in data.
When it comes to selecting a cloud data solution, it’s important to take stock of your team’s current skill sets. Ask yourself if you’re going to have to train current employees or hire new headcount. Either way, this is an additional cost you need to factor into your implementation.
Aside from skillsets, you should also assess how easy it is to complete every-day actions in the tool. If repetitive actions are even an increment more difficult than a previously used tool, your team will not only spend more time, but they’ll also encounter more frustrating scenarios. Let’s take a look at what the industry says about Databricks vs Snowflake:
Databricks is seen as the professional tool geared towards more technical audiences.The user interface (UI) is notably intricate, and demands more hands-on input for tasks such as adjusting cluster sizes, modifying configurations, or switching preferences. There is a steeper learning curve to overcome.
Many in the industry say that Snowflake is one of the most user-friendly warehouses on the market. Its intuitive SQL centric interface simplifies the process of setup and initiation. Furthermore, it offers plenty of automation features to facilitate ease of use.
Takeaway: Consult your data team—the folks who will be working in the tool each and every day. See what tools they are familiar with, have them try out the free trials, and choose the tool that’s going to make their job easiest.
Of course, cost is always an important factor when making a SaaS purchase, but cloud computing costs are an especially important consideration. The way you use the tool may be better suited for the pricing structure of one data warehouse over the other—this is all depending on your company’s computing needs.
Comparing Databricks and Snowflake in terms of pricing, Databricks has an interesting cost structure which can be broken down into two sub-components. The first element is paid directly to your cloud service provider for the duration of time that your compute processing is active. The second element is where Databricks comes in. This price is structured based on the amount of DBUs or compute resources you consume—basically how long that compute cluster is running. This is why performance is so important. That said, it’s important to note that there are no up-front fees because Databricks uses per-second billing for this pay-as-you-go model.
Snowflake’s pricing is more aligned with a traditional cloud data warehouse like Redshift or Google Big Query. Their cost is an aggregate of the cost of using data transfer, data storage, and compute resources. This model is designed to offer flexibility and scalability for organizations of different sizes and data processing needs.
Takeaway: We recommend having a demo or one-on-one consultation with both Databricks and Snowflake to understand what types of costs you can expect based on your organization’s data volume, consumption, and individual use cases. There is no one-size-fits-most calculator here.
More reading: How to optimize your cloud data costs.
Perhaps even more important than cost is use case analysis—understanding what kind of businesses are using what kind of data storage solution to best perform their core functions.
Because Databricks is a mature, Spark-based platform, they’re trusted for processing streaming data, machine learning, and data science-based analytics use cases. And its ability to handle raw, unprocessed data makes it great for non-standardized file types like images, text, and even social media data.
Snowflake is also used for machine learning and data science, thanks to their new-ish Snowpark and Snowflake ML packages. However, Snowflake is probably best known for its easy-to-use SQL editor. This feature makes Snowflake an incredible tool for data transformation, analysis, and reporting.
Takeaway: Before you invest in a tool, make sure you are aligned on your data strategy. How will your company be using data? If ML or AI are an important part of your product, be sure to test out both tools and see which one is the best fit for your data team.
Full disclaimer, at ThoughtSpot, we strategically partner with both Snowflake and Databricks. We believe both solutions are an essential part of the cloud data ecosystem, and our customers are often more than satisfied with the technology, support, and success they have.
Consider Wellthy, who doubled their data team’s velocity of output by combining ThoughtSpot and Snowflake. When asked about the value this brought their business, here’s what Kelly Burdine, Head of Data Science and Analytics at Wellthy, had to say:
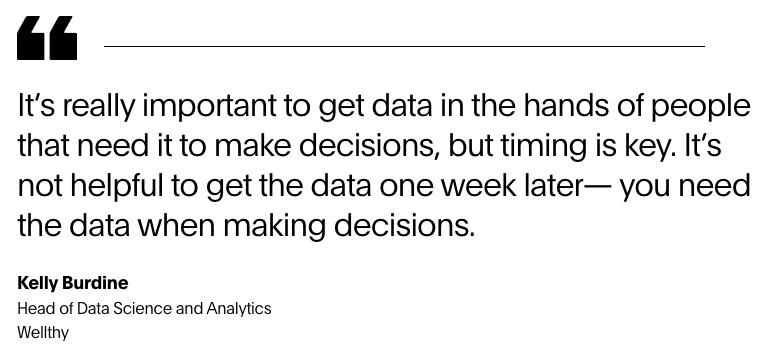
Or consider Fabuwood, who decommissioned over 50 manual reports by working with Databricks and ThoughtSpot. Now, the sales team can access live sales data without having to wait a month to generate a new Power BI report. Here’s what David Samet, Director of Technology at Fabuwood, had to say:
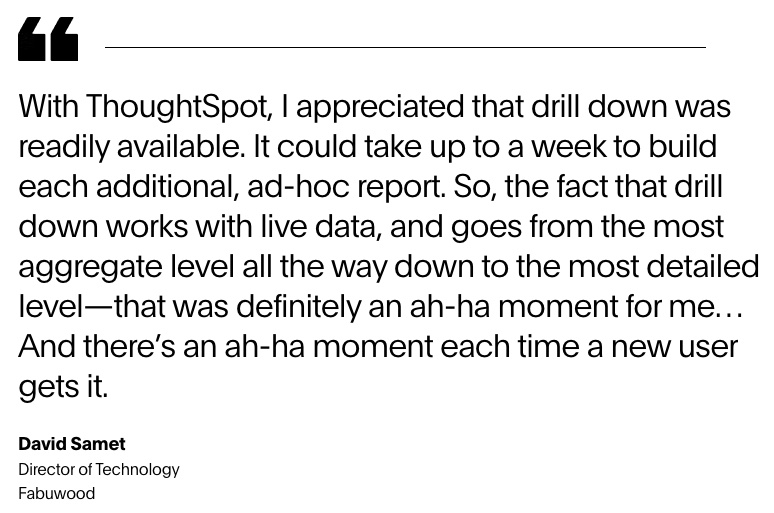
At the end of the day, it’s all about helping customers get the most out of their data investments—and we do that through co-innovation and collaboration. Learn more about our partnership with Databricks and Snowflake, or schedule a one-on-one demo to discuss how we can help you build out a modern data stack for your business.