Customers wanting to drive self-service analytics as part of creating a data-driven organization will often ask, “Can we achieve self service analytics, when our work force has low data literacy?” Or they might say they are not ready for self-service analytics, incorrectly thinking they need first to improve data literacy.
But the two are inextricably linked. I liken it to teaching a child to read without giving them any books on which to build their skills.
What is data literacy?
As I wrote in ”Successful Business Intelligence”, there is a big difference between technical literacy and data literacy. As an industry, we have spent far too much time training people on hard-to-use BI tools and a woefully insufficient amount of time on data literacy. Gartner defines data literacy as the ability to read, write, and speak data in a business context. But instead of thinking about this as data — something potentially intimidating and new — you should think of it as the language of the business. For example, baseball analysts speak the language of RBI (runs batted in) and WHIPS (wins, hits, per innings pitched). Professionals in people analytics understand attrition, retention rates, and diversity metrics. And those who work in insurance will be familiar with ICDs (insurance claims data) and CHAM (Client High Acuity Model)
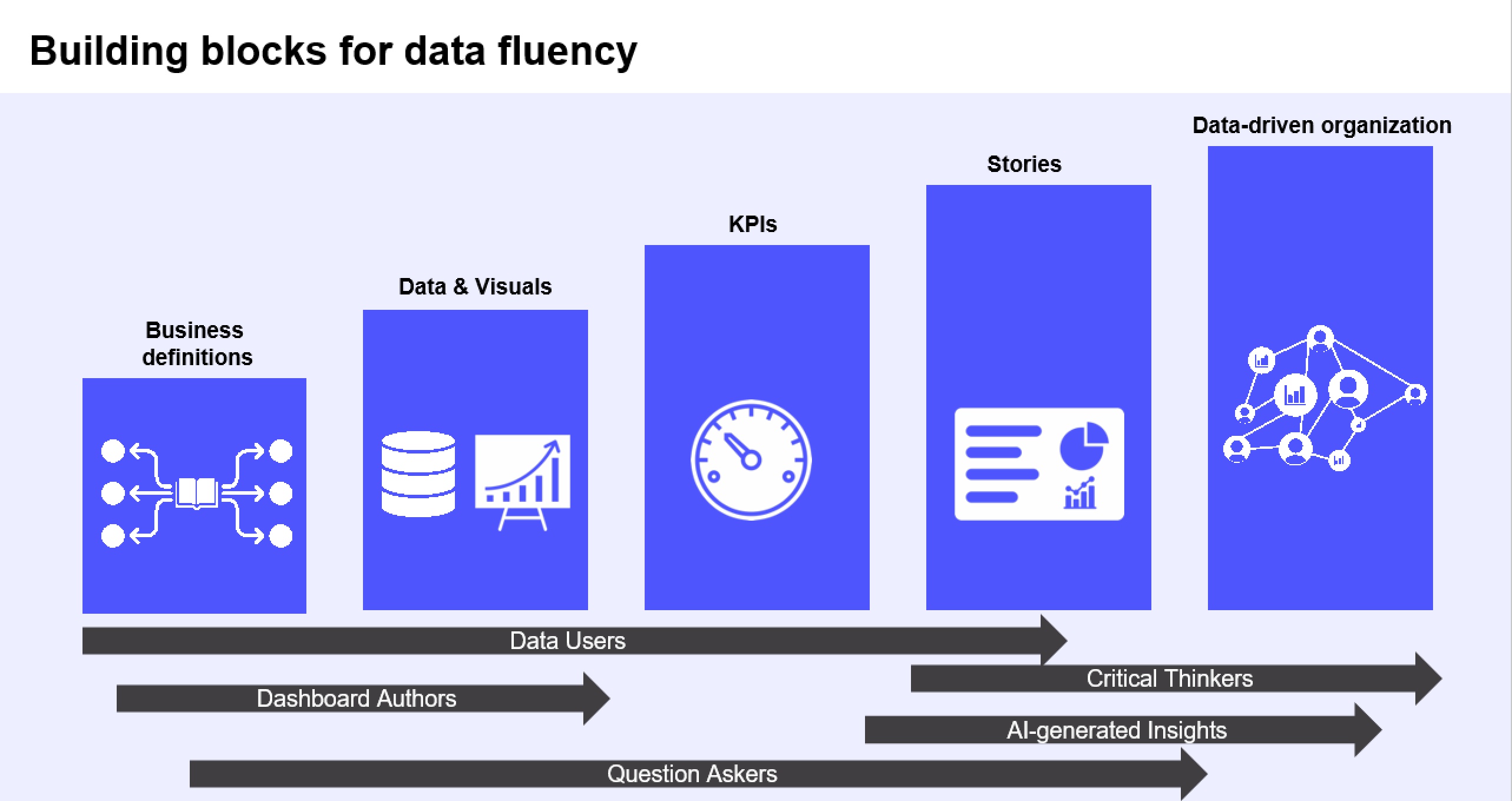
Data literacy vs. data fluency
Data fluency is about more than the ability to read and communicate with data, it’s about thinking in data terms. After twenty years in this space, I routinely think about what data can be brought to bear on any business problem. For example:
Supply chain disruption: Where else can I source products from? Will human mobility tell me where demand is shifting?
Public health: Will DNA, blood type, and lifestyle data tell me who is more likely to get seriously ill from COVID-19? (Yes, it will..)
Just as not everyone reads at the same level of proficiency, not everyone needs to be at the same level of data fluency. A data engineer may need to understand where data originates, if there are data gaps, potential biases and so on, while a manager may only need to interpret and interact with data. However, I would argue that there is a baseline level of fluency everyone needs in order to be able to think critically about data and to recognize when there are both gaps and biases.
Two recent examples that show how poor we are at this:
LinkedIn recently shared data on the mass exodus of workers out of Silicon Valley. But did that many people actually move? Or is this only knowledge workers likely to have LinkedIn profiles? What about those in the hospitality sector? This data set has many gaps and biases, as do most data sources.
COVID-19 case counts are drastically underreported in many countries because there is not widespread testing nor an ability to capture the data. The same was true in the USA and Europe in the early days of the pandemic. Testing may be more readily available now, but not everyone who gets sick gets tested, continuing to skew data.
In this way, it’s just as Valerie Logan, founder of The Data Lodge and former lead research analyst on the topic at Gartner, describes it: data literacy is not just a business skill, it is a life skill.
The state of data literacy
According to research by Accenture, only 21% of business people feel confident with data. The World Economic Forum cites data-related jobs as the top three in demand but with a wide gap in available talent. These skills are not yet being taught in most education systems, although this varies by country. The U.S. federal government has made improving data literacy part of the Federal Data Strategy.
As organizations have accelerated their digital transformation efforts and the desire to be data-driven is now an executive and board-level conversation, companies must take charge in establishing formal data fluency programs. As a June 2021 LinkedIn poll shows below, many are exploring such programs, with 13% well on their way and scaling their programs.
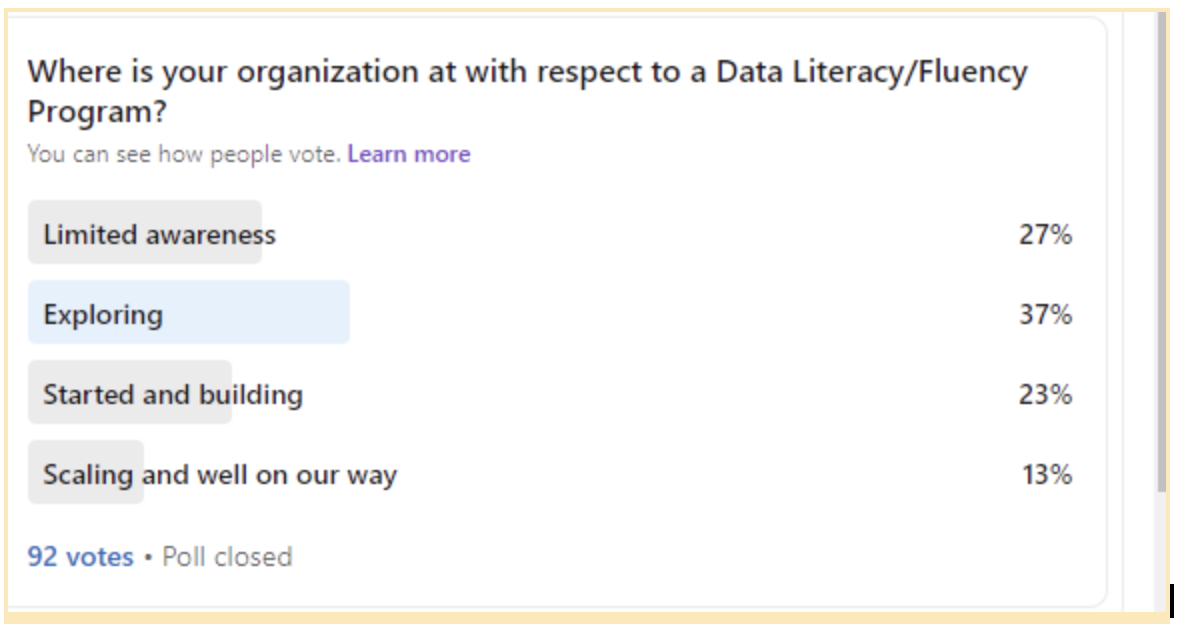
Best practices to drive data fluency
To build a more data fluent workforce, follow these best practices:
Make improving data fluency a core organization objective, led by the CDO but in partnership with People Ops and lines of business.
Partner with local universities and online education providers in fostering analytical, data storytelling, and technical skills.
Differentiate between technical skills and data skills with the requisite domain expertise and in-source the delivery of domain-specific training.
Evaluate job roles and levels to inventory existing skills and map the different levels of proficiency required by role and level.
Ensure upskilling is continuous and bite-sized. Think beyond traditional classroom-style trainings to include lunch and learns, weekly office hours, analytics days, and in-app learning.
Reward and gamify skill building with badges and certificates.
Additional resources to dig deeper
Tune in to the The Data Chief Live to hear how Red Hat and Nationwide Building are investing in data fluency, with best practices from Valerie Logan, The Data Lodge
How to build data literacy in your company, MIT Management