Everyone seems to be discussing machine learning and artificial intelligence, but few people really know how to take advantage of the new technology. Did you know that according to the MIT Sloan Management Review 76% of companies say they're using machine learning to increase their sales growth?
So why does almost every BI and analytics professional I talk to think that machine learning is the domain of a few statisticians or data scientists trained to use algorithms or advanced analytics technologies? Make no mistake, machine learning, artificial intelligence, and the newer offshoot deep learning are complex topics, but you don’t need to understand them in depth to take advantage of them.
In fact, your data analytics strategy should focus on these technologies. But where do you get started? How do you apply machine learning concepts to an analytic or business intelligence strategy?
After you read this post, you should have the beginning of a roadmap to help you take advantage of this exciting technology. There’s no simple formula, but there are simple things you can do to get started down the path.
"By far the greatest danger of Artificial Intelligence is that people conclude too early that they understand it."—Eliezer Yudkowsky
1. Hire Those Experts
Advanced analytics is much more than statisticians sitting in a dark corner preparing data and running complex algorithms. But whether you call them statisticians or data scientists, they're still an important part of your business intelligence (BI) strategy.
Why? Because once they've built the models and found some initial insights, the resulting information needs to find its way to the people who own the business decisions. And making data available for decisions is the foundation of business intelligence.
Who they are
The Data Scientist job title is sometimes mistakenly applied to general analysts or other BI professionals, but what you're looking for here are people who have mathematical training as well as experience applying statistical models to find patterns in data.
It's not a job for generalists, but the best candidates will have experience with analytic tools like SPSS, SAS, R, or even python. In fact, Data Scientist is one of the fastest growing job categories in technology.
Start with a small team—or only one—to get up and running.
Job Postings for Data Scientists
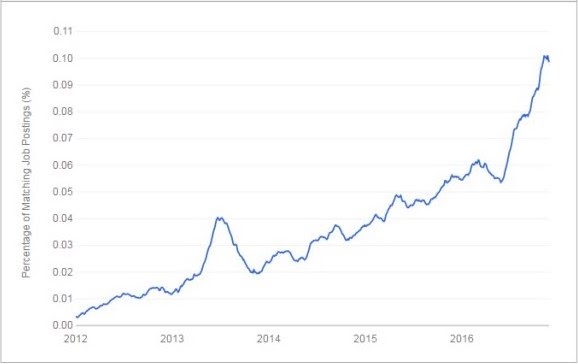
Source: indeed.com job trends - https://www.indeed.com/jobtrends
Common Use Cases
So what will these people do? Analyze data, of course! Data Scientists identify trends and patterns in the data using “guided machine learning”—machine learning that’s utilized by an expert in the field.
Some common business challenges a data scientist might focus on include:
Fraud analysis. Combing through huge amounts of transactional data--call detail records, point of sale transactions, trading activity--to find specific activity that's likely to be fraud.
Reduce inventory carry costs. How many harvest gold throw pillows do you really need to stock for that upcoming resurgence of 70s nostalgia? Advanced analytics can tell you.
Market basket analysis. Who knew that people who buy diapers are much more likely to buy beer in the same shopping trip? Data scientists, of course!
Risk analysis. Understand the risk of a portfolio before the next market crisis.
Guided machine learning isn't the only game in town though, and it's rapidly becoming less critical as other more automated methods come of age.
2. Find Real Time Anomalies
Do you have systems that constantly stream data? Collecting that data and analyzing it has value, but wouldn't it be even better to find outliers as they're happening?
By identifying the outliers in real-time, you can take immediate action. This is particularly useful for fraud analysis (find the fraud as it’s occurring, not a week later), online buying behaviors (keep the customer on your website instead of identifying them after they’ve bought an item somewhere else), and anywhere else where immediate information can drive valuable decisions.
Traditionally the models built by data scientists have been used by streaming analytics software to do exactly that, but with the maturation of machine learning it's even more effective. Software like IBM Streams and DataTorrent enables this, and those anomalies can trigger another action (sound a fraud alert!) or be collected for later analysis.
This information should be fed back into your BI system to inform product development and policy changes. Catching problems as they occur is great, but wouldn't it be better to fix the system so that they don't occur in the first place?
I worked with a large oil and gas firm a few years ago that used real-time analytics to identify anomalies in wave patterns in the Gulf of Mexico. Being able to react in real-time to changes in current and weather allowed them to take the right action on their oil rigs, resulting in greater operating efficiency and more effective identification of potential drilling sites.
Machine learning and advanced analytics combined with a modern business intelligence platform can make that happen.
3. Use a Search-based BI Platform
Business intelligence has traditionally been about skilled analysts producing answers for other people. That’s what reports and dashboards are after all—sophisticated answers to known questions.
The industry has been focused on making those answers increasingly sophisticated over the past 20 years, but other than that not much has changed.
The incredible success of search-based consumer information systems like Google, LinkedIn, and Yelp is starting to change that. Companies like ThoughtSpot and Microsoft’s Power BI are bringing that same search experience to our work life.
The success of these BI platforms—both consumer and business—is very dependent on machine learning.
Train the data and the business intelligence platform to get answers for people instead of building all answers manually in advance. This is the approach products like Amazon take. Search suggestions change as the questions people ask change. The look of the product also changes based on behavior. My Amazon home screen and the suggestions I get don’t look the same as yours, but nobody is picking those suggestions manually—they were created with machine learning.
Leveraging search-based BI is critical to closing that last mile for end users, and I believe is in the process of revolutionizing BI. After all, that’s why I chose to work for ThoughtSpot!
4. Hire a Bot
BI is all about getting the right data to the point of decision in the easiest way possible. And for most of us, what’s easier than talking?
Enter the bots.
I don’t know about you, but when I think of bots the first thing that comes to mind is the often not-so-helpful “how can I help you” bots on the web pages of airlines and hotel companies. And the initial wave of bot technology often wasn’t that helpful.
Those bots were more about offloading simple questions from support than they were about actually helping people.
That’s not what today’s bots are about, though. Instead of serving a support function, think of today’s bots as bringing data to existing workflows in as low-impact a way as possible. And it’s all enabled with machine learning, sprinkled with an ever-increasing dose of artificial intelligence.
Maybe you’re typing away in Slack or Salesforce Chatter, asking someone how many customers you have in the retail industry. The best bots today will see that question, and jump in with an answer.
Your workflow doesn’t change. The answer comes to you, you don’t have to come to the answer.
What platforms do your internal customers use every day? How do their workflows intersect with those platforms? What bots can you leverage to change the way people get answers?
It’s all business intelligence, after all.
5. Forecast the Future
Centuries ago, most “forecasting” was done with tea leaves and crystal balls. These days, we like more “intelligence” in our “business.”
Forecasting has evolved a lot since those days. We’ve moved from gut instinct, to Excel-based statistical forecasting, to demand modeling. But things are changing.
This is where machine learning comes in.
Whether you’re trying to estimate future monthly sales, optimize your supply chain, or choose the optimal price for hotel rooms, forecasting is all about predicting the future using data from the past. And when it comes to churning through large amounts of data, nobody can beat a machine.
In fact, recent advances in neural networks are pushing this technology to a new level, giving business people actual answers instead of models they can use to predict those answers. Forecasting will be one of the immediate beneficiaries of this technology, making very accurate estimates of future behavior just as accessible as historical data.
How are you using machine learning to get people answers?
Tell me your thoughts in the comments below, I’d love to hear from you.